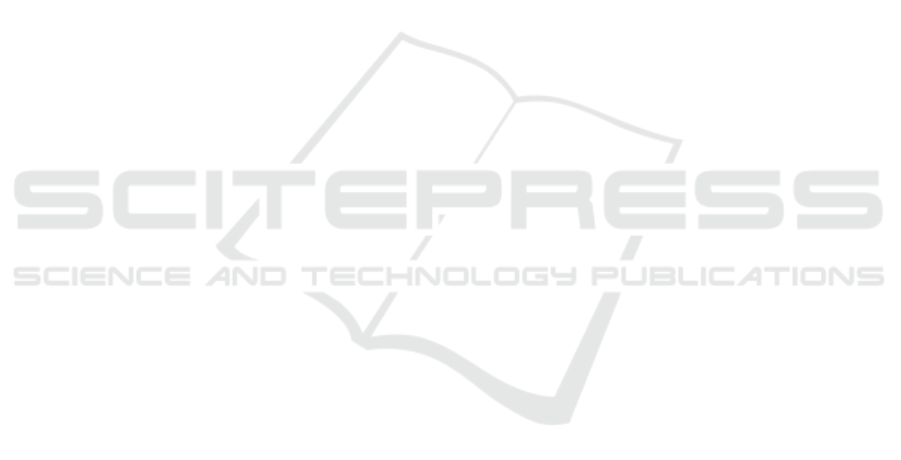
glow. Nature, 575(7783):464–467.
Aharonian, F., Ait, B. F., Bernl
¨
ohr, K., Bordas, P.,
Casanova, S., Chakraborty, N., Deil, C., Donath, A.,
Hahn, J., Hermann, G., et al. (2019). Resolving the
crab pulsar wind nebula at teraelectronvolt energies.
Nature Astronomy.
Ambrosi, G., Awane, Y., Baba, H., et al. (2013). The
Cherenkov Telescope Array Large Size Telescope.
Proceedings of the 33rd International Cosmic Ray
Conference, pages 8–11.
Bahdanau, D., Cho, K., and Bengio, Y. (2015). Neural ma-
chine translation by jointly learning to align and trans-
late. In Bengio, Y. and LeCun, Y., editors, 3rd Interna-
tional Conference on Learning Representations, ICLR
2015, San Diego, CA, USA, May 7-9, 2015, Confer-
ence Track Proceedings.
Brunel, A., Pasquet, J., PASQUET, J., Rodriguez, N.,
Comby, F., Fouchez, D., and Chaumont, M. (2019).
A cnn adapted to time series for the classification of
supernovae. Electronic Imaging, 2019(14):90–1.
Cao, J., Li, Y., and Zhang, Z. (2018). Partially shared
multi-task convolutional neural network with local
constraint for face attribute learning. In Proceedings
of the IEEE Conference on Computer Vision and Pat-
tern Recognition, pages 4290–4299.
Caruana, R. (1997). Multitask learning. Machine learning,
28(1):41–75.
Chen, Z., Badrinarayanan, V., Lee, C.-Y., and Rabinovich,
A. (2018). GradNorm: Gradient normalization for
adaptive loss balancing in deep multitask networks.
In Dy, J. and Krause, A., editors, Proceedings of the
35th International Conference on Machine Learning,
volume 80 of Proceedings of Machine Learning Re-
search, pages 794–803. PMLR.
de Naurois, M. and Rolland, L. (2009). A high performance
likelihood reconstruction of γ-rays for imaging atmo-
spheric Cherenkov telescopes. Astroparticle Physics,
32(5):231–252.
Feng, Q., Lin, T. T., Collaboration, V., et al. (2016). The
analysis of veritas muon images using convolutional
neural networks. Proceedings of the International As-
tronomical Union, 12(S325):173–179.
Fiasson, A., Dubois, F., Lamanna, G., Masbou, J., and
Rosier-Lees, S. (2010). Optimization of multivariate
analysis for IACT stereoscopic systems. Astroparticle
Physics, 34.
Guo, M., Haque, A., Huang, D.-A., Yeung, S., and Fei-Fei,
L. (2018). Dynamic task prioritization for multitask
learning. In Proceedings of the European Conference
on Computer Vision (ECCV), pages 270–287.
He, K., Gkioxari, G., Dollar, P., and Girshick, R. (2017).
Mask R-CNN. In Proceedings of the IEEE Interna-
tional Conference on Computer Vision (2017).
He, K., Zhang, J., Ren, S., and Sun, J. (2016a). Iden-
tity mappings in deep residual networks. In Euro-
pean conference on computer vision, pages 630–645.
Springer.
He, K., Zhang, X., Ren, S., and Sun, J. (2016b). Deep resid-
ual learning for image recognition. In Proceedings of
the IEEE conference on computer vision and pattern
recognition, pages 770–778.
Hillas, A. (1985). Cerenkov light images of eas produced
by primary gamma. In International Cosmic Ray Con-
ference, volume 3.
Hoppe, S. et al. (2009). Detection of very-high-
energy gamma-ray emission from the vicinity of
PSR B1706-44 with H.E.S.S. arXiv e-prints, page
arXiv:0906.5574.
Hu, J., Shen, L., and Sun, G. (2018). Squeeze-and-
excitation networks. In Proceedings of the IEEE con-
ference on computer vision and pattern recognition,
pages 7132–7141.
Iizuka, S., Simo-Serra, E., and Ishikawa, H. (2016). Let
there be color! joint end-to-end learning of global and
local image priors for automatic image colorization
with simultaneous classification. ACM Transactions
on Graphics (ToG), 35(4):1–11.
Jacquemont, M., Antiga, L., Vuillaume, T., Silvestri, G.,
Benoit, A., Lambert, P., and Maurin, G. (2019). In-
dexed operations for non-rectangular lattices applied
to convolutional neural networks. In Proceedings of
the 14th International Joint Conference on Computer
Vision, Imaging and Computer Graphics Theory and
Applications - Volume 5: VISAPP,, pages 362–371.
INSTICC, SciTePress.
Kendall, A., Gal, Y., and Cipolla, R. (2018). Multi-task
learning using uncertainty to weigh losses for scene
geometry and semantics. In Proceedings of the IEEE
Conference on Computer Vision and Pattern Recogni-
tion, pages 7482–7491.
Kim, B., Brill, A., Miener, T., Nieto, D., and Feng,
Q. (2019). DL1-Data-Handler: DL1 HDF5 writer,
reader, and processor for IACT data. https://doi.org/
10.5281/zenodo.3336561. v0.8.1-legacy.
Kim, E. J. and Brunner, R. J. (2016). Star-galaxy clas-
sification using deep convolutional neural networks.
Monthly Notices of the Royal Astronomical Society,
page stw2672.
Kingma, D. P. and Ba, J. (2015). Adam: A method for
stochastic optimization. In Bengio, Y. and LeCun,
Y., editors, 3rd International Conference on Learn-
ing Representations, ICLR 2015, San Diego, CA, USA,
May 7-9, 2015, Conference Track Proceedings.
Luvizon, D. C., Picard, D., and Tabia, H. (2018). 2d/3d
pose estimation and action recognition using multitask
deep learning. In Proceedings of the IEEE Conference
on Computer Vision and Pattern Recognition.
Mangano, S., Delgado, C., Bernardos, M. I., et al. (2018).
Extracting gamma-ray information from images with
convolutional neural network methods on simulated
cherenkov telescope array data. In IAPR Workshop
on Artificial Neural Networks in Pattern Recognition,
pages 243–254. Springer.
Nieto, D., Brill, A., Feng, Q., Jacquemont, M., Kim, B.,
Miener, T., and Vuillaume, T. (2019). Studying deep
convolutional neural networks with hexagonal lattices
for imaging atmospheric cherenkov telescope event
reconstruction. In ICRC 2019 - 36th International
Cosmic Ray Conference.
Multi-Task Architecture with Attention for Imaging Atmospheric Cherenkov Telescope Data Analysis
543