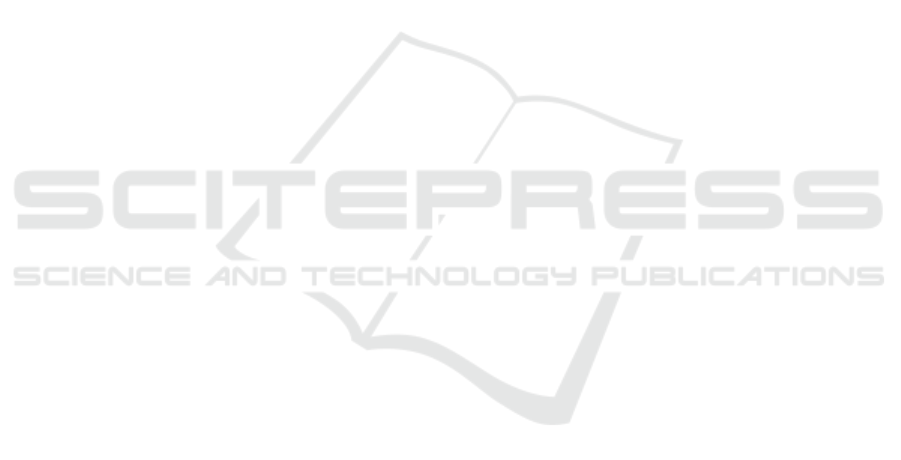
because we cannot obtain the latent, subclinical
infectious, and clinical infectious states from in-
cubation and infectious states. Although we can
randomly generate the latent and sub-clinical du-
rations to match the incubation duration and the
sub-clinical and clinical durations to match the
infectious duration in InterSpread Plus, this will
generate high uncertainty due to lack of knowl-
edge.
In summary, the definition of criteria for parame-
ter transformation is dependent on a number of con-
cepts that are reflected in the parameters’ settings.
• Concepts must exist in both models. It is a condi-
tion for a transformation to take place.
• With missing concepts, we can perform the pa-
rameter transformation as described in a transfor-
mation procedure with uncertainty.
4 CONCLUSIONS
In this paper, we propose the use of a meta-ontology
framework to capture the semantics of parameters and
related domain knowledge associated with an FMD
course of infection in animal disease spread simula-
tion models. It permits parameter knowledge sharing,
parameter assessment and parameter transformation
between models. Our motivation for this approach
is to minimize the ambiguity that exists in parame-
ter settings and allow a standard way to describe pa-
rameter settings and the related domain knowledge.
It promotes the interoperability between simulation
models, and the ability to assess domain knowledge.
By explicitly describing parameter knowledge and es-
tablishing the linkage between parameters and doc-
umented domain knowledge, this allows us to have
an understanding of the differences between different
models’ parameters and views of the domain knowl-
edge. It strives to provide a basis for a new way to
understand and assess parameter and related views of
domain knowledge. The central piece of this work is
the focus on the meta-ontology framework construc-
tion in capturing the semantics of the parameters, the
related FMD course of infection domain concepts and
assisting in the assessment and the transformation of
parameters between models. This work reports on a
novel ontological organization that separates domain
knowledge from the knowledge about the parameters
in different comparable simulation models and for-
malizes a relationship between parameters by linking
to the domain knowledge part of the ontological struc-
ture. It allows explicit knowledge representation, a
means to compare animal disease spread simulation
models and a means to evaluate views (as expressed
in parameters) related to simulation models and do-
main knowledge. This work also acknowledges the
limitations in ontology creation. It is a time consum-
ing process that requires great effort and collaboration
of a number of experts in different domains. In gen-
eral, without experts’ assistance, parameter settings
alone are not sufficient to account for the differences
between the models’ parameters due to differences in
parameter representation of the models and their as-
sumptions. The introduction of an ontology provides
a standard means to document and describe the views
of simulation models and views of the domain knowl-
edge. These views are built from ontological concepts
that are reflected by the parameters, their semantics
and related domain knowledge. The ability to cap-
ture conceptual relations, properties and the ability
to verify the consistency of the ontology allows facts
related to parameter settings to be assessed not only
with other simulation models but also to the related
domain knowledge.
In future work, we hope to extend our ontological
concepts to other domains and to extend the number
and types of tasks that our semantic engine can per-
form including validation of requirements, compari-
son of concepts between related ontologies, and the
transformation of concepts and values between on-
tologies. We anticipate that these extensions will find
use in many domains where there is a need to compare
and reconcile competing ontologies.
REFERENCES
Arendt, P. D., Apley, D. W., and Chen, W. (2012). Quantifi-
cation of model uncertainty: Calibration, model dis-
crepancy, and identifiability. Journal of Mechanical
Design, Transactions of the ASME.
C., K. A., Patterson, G., L., V. K., E., C. M., and M., P. A.
(2016). Parameter values for epidemiological models
of foot-and-mouth disease in swine. Frontiers in Vet-
erinary Science, 3:44.
Fox, M. S. and Gruninger., M. (1995). Methodology for the
design and evaluation of ontologies. In Proc. of the
Workshop on Basic Ontological Issues in Knowledge
Sharing, 1995.
G., D. (2001). The foot and mouth disease (fmd) epidemic
in the united kingdom. Comp imm, Microb and Inf Dis
2002, 1(25):331–343.
Harvey, N. and Reeves, A. (2012). Model Description for
the North American Animal Disease Spread Model
4.0. The NAADSM Development team.
Harvey, N., Reeves, A., Schoenbaum, M., et. al. (2007).
The North American Animal Disease Spread Model:
A simulation model to assist decision making in eval-
HEALTHINF 2021 - 14th International Conference on Health Informatics
232