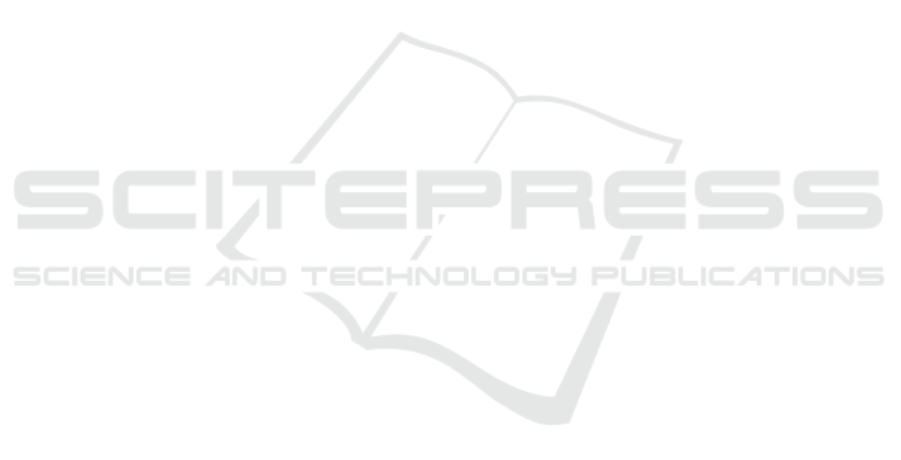
Harel, J., Koch, C., and Perona, P. (2007). Graph-based vi-
sual saliency. In Sch
¨
olkopf, B., Platt, J. C., and Hoff-
man, T., editors, Advances in Neural Information Pro-
cessing Systems 19, pages 545–552. MIT Press.
Hariharan, B. and Girshick, R. (2017). Low-shot visual
recognition by shrinking and hallucinating features. In
Pro. of ICCV, pages 3018–3027.
He, K., Zhang, X., Ren, S., and Sun, J. (2016). Deep
residual learning for image recognition. In IEEE Con-
ference on Computer Vision and Pattern Recognition,
pages 770–778.
Hoffman, J., Gupta, S., and Darrell, T. (2016). Learning
with side information through modality hallucination.
In Proc. of CVPR, pages 826–834.
Huang, S., Xu, Z., Tao, D., and Zhang, Y. (2016). Part-
stacked cnn for fine-grained visual categorization. In
IEEE Conference on Computer Vision and Pattern
Recognition, pages 1173–1182.
Itti, L., Koch, C., and Niebur, E. (1998). A model of
saliency-based visual attention for rapid scene anal-
ysis. IEEE Transactions on Pattern Analysis and Ma-
chine Intelligence, 20(11):1254–1259.
Ji, R., Wen, L., Zhang, L., Du, D., Wu, Y., Zhao, C., Liu, X.,
and Huang, F. (2020). Attention convolutional binary
neural tree for fine-grained visual categorization. In
Proc. of CVPR, pages 10468–10477.
K
¨
ummerer, M., Wallis, T. S. A., and Bethge, M. (2016).
Deepgaze ii: Reading fixations from deep fea-
tures trained on object recognition. arXiv preprint
arXiv:1610.01563.
Kong, S. and Fowlkes, C. (2017). Low-rank bilinear pool-
ing for fine-grained classification. In Proc. of CVPR,
pages 365–374.
Krause, J., Stark, M., Deng, J., and Fei-Fei, L. (2013).
3d object representations for fine-grained categoriza-
tion. In 4th IEEE Workshop on 3D Representation and
Recognition, at ICCV, pages 1–8.
Krizhevsky, A., Sutskever, I., and Hinton, G. E. (2012). Im-
agenet classification with deep convolutional neural
networks. In Advances in Neural Information Pro-
cessing Systems, pages 1097–1105.
Lin, D., Shen, X., Lu, C., and Jia, J. (2015). Deep
lac: deep localization, alignment and classification for
fine-grained recognition. In Proc. of CVPR, pages
1666–1774.
Luo, W., Yang, X., Mo, X., Lu, Y., Davis, L., Li, J., Yang,
J., and Lim, S.-N. (2019). Cross-x learning for fine-
grained visual categorization. In Proc. of ICCV.
Murabito, F., Spampinato, C., Palazzo, S., Pogorelov, K.,
and Riegler, M. (2018). Top-down saliency detection
driven by visual classification. Computer Vision and
Image Understanding, 172:67–76.
Nilsback, M.-E. and Zisserman, A. (2008). Automated
flower classification over a large number of classes. In
Sixth Indian Conference on Computer Vision, Graph-
ics & Image Processing, pages 722–729.
Sun, M., Yuan, Y., Zhou, F., and Ding, E. (2018). Multi-
attention multi-class constraint for fine-grained image
recognition. In Proc. of ECCV, pages 834–850.
Torralba, A., Oliva, A., Castelhano, M. S., and Hender-
son, J. M. (2006). Contextual guidance of eye move-
ments and attention in real-world scenes: The role of
global features in object search. Psychological Re-
view, 113(4):766–786.
Wang, F., Jiang, M., Qian, C., Yang, S., Li, C., Zhang, H.,
Wang, X., and Tang, X. (2017). Residual attention
network for image classification. In IEEE Conference
on Computer Vision and Pattern Recognition, pages
3156–3164.
Wang, Y., Morariu, V. I., and Davis, L. S. (2018a). Learn-
ing a discriminative filter bank within a cnn for fine-
grained recognition. In Proc. of CVPR, pages 4148–
4157.
Wang, Y.-X., Girshick, R., Hebert, M., and Hariharan, B.
(2018b). Low-shot learning from imaginary data. In
Proc. of CVPR, pages 7278–7286.
Wang, Z., Wang, S., Yang, S., Li, H., Li, J., and Li, Z.
(2020). Weakly supervised fine-grained image classi-
fication via guassian mixture model oriented discrim-
inative learning. In Proc. of CVPR, pages 9749–9758.
Wei, X.-S., Xie, C.-W., Wu, J., and Shen, C. (2018).
Mask-cnn: Localizing parts and selecting descriptors
for fine-grained bird species categorization. Pattern
Recognition, 76:704 – 714.
Welinder, P., Branson, S., Mita, T., Wah, C., Schroff, F.,
Belongie, S., and Perona, P. (2010). Caltech-UCSD
Birds 200. Technical Report CNS-TR-2010-001, Cal-
ifornia Institute of Technology.
Xiao, T., Xu, Y., Yang, K., Zhang, J., Peng, Y., and Zhang,
Z. (2015). The application of two-level attention
models in deep convolutional neural network for fine-
grained image classification. In Proc. of CVPR, pages
842–850.
Xie, G.-S., Zhang, X.-Y., Yang, W., Xu, M., Yan, S., and
Liu, C.-L. (2017). Lg-cnn: From local parts to global
discrimination for fine-grained recognition. Pattern
Recognition, 71:118–131.
Zhang, H., Xu, T., Elhoseiny, M., Huang, X., Zhang, S., El-
gammal, A., and Metaxas, D. (2016a). Spda-cnn: Uni-
fying semantic part detection and abstraction for fine-
grained recognition. In IEEE Conference on Com-
puter Vision and Pattern Recognition, pages 1143–
1152.
Zhang, H., Zhang, J., and Koniusz, P. (2019). Few-shot
learning via saliency-guided hallucination of samples.
In Proc. of CVPR, pages 2770–2779.
Zhang, N., Donahue, J., Girshick, R., and Darrell, T. (2014).
Part-based r-cnns for fine-grained category detection.
In European Conference on Computer Vision, pages
834–849.
Zhang, X., Xiong, H., Zhou, W., Lin, W., and Tian, Q.
(2016b). Picking deep filter responses for fine-grained
image recognition. In Proc. of CVPR, pages 1134–
1142.
Zheng, H., Fu, J., Mei, T., and Luo, J. (2017). Learning
multi-attention convolutional neural network for fine-
grained image recognition. In Proc. of ICCV, pages
5209–5217.
Hallucinating Saliency Maps for Fine-grained Image Classification for Limited Data Domains
171