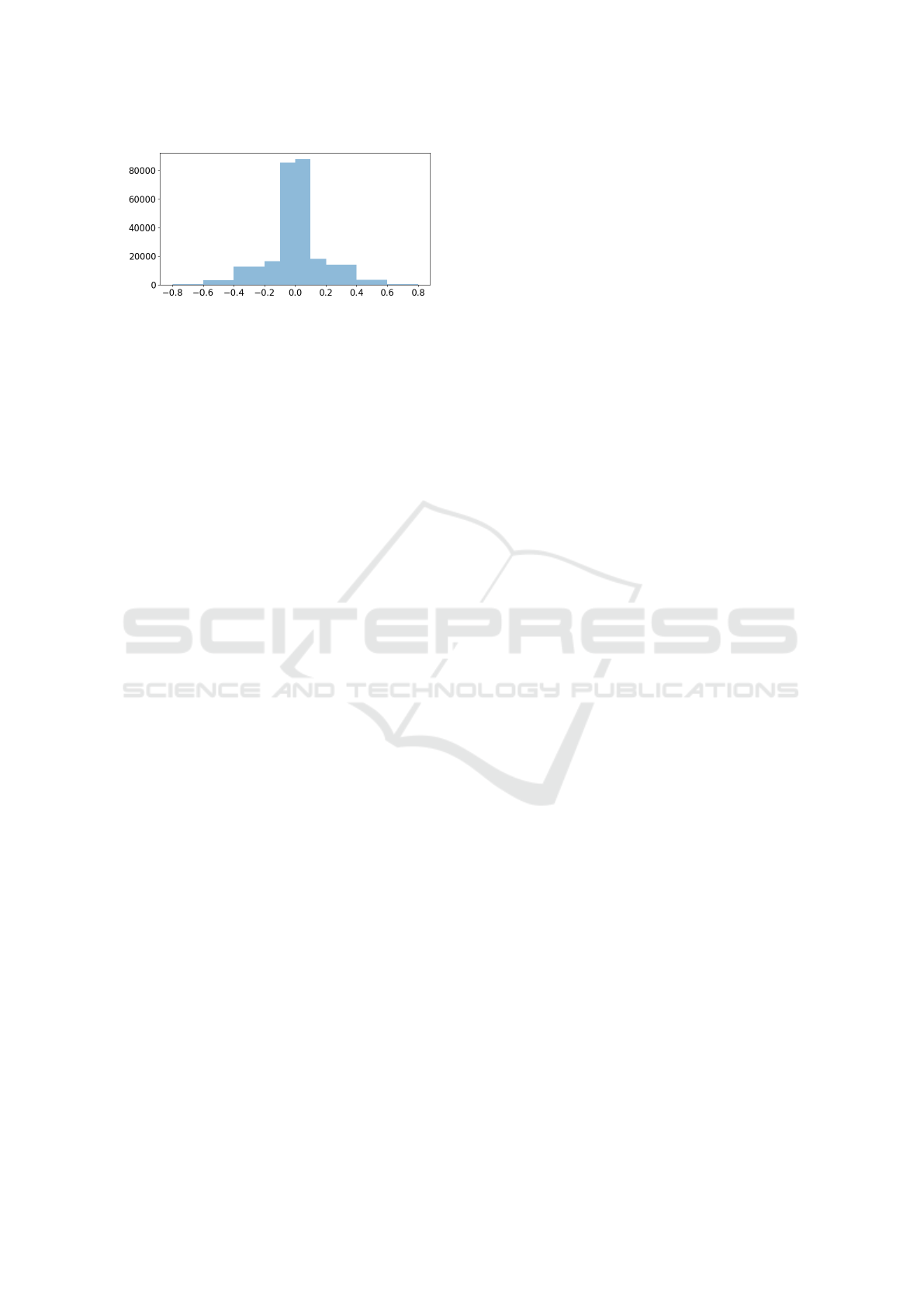
Figure 7: Differences in the abnormality prediction scores
obtained by DenseNet121 on MIMIC-CXR and style-
adjusted MIMIC-CXR by CycleGAN is binned into non-
overlapping intervals, and the counts in every interval are
displayed.
to abnormality detection with adversarial training is
also possible, which is out-of-scope of this paper. Our
main contribution in this work is the introduction of
distribution of high-frequency components to char-
acterize the data sources and relating it to the diffi-
culty of pre-trained models to generalize on unseen
domains. In this work, we have introduced a frame-
work for domain-shift detection and removal to over-
come this problem.
ACKNOWLEDGEMENTS
This research is sponsored in whole or in part by the
AI Initiative (LOIS 9613) and Privacy research (LOIS
9831) as part of the Laboratory Directed Research and
Development Program of Oak Ridge National Labo-
ratory.
REFERENCES
Andreux, M., Angles, T., Exarchakis, G., Leonarduzzi, R.,
Rochette, G., Thiry, L., Zarka, J., Mallat, S., And
´
en,
J., Belilovsky, E., et al. (2020). Kymatio: Scattering
transforms in python. Journal of Machine Learning
Research, 21(60):1–6.
Bousmalis, K., Trigeorgis, G., Silberman, N., Krishnan, D.,
and Erhan, D. (2016). Domain separation networks. In
Advances in neural information processing systems,
pages 343–351.
Bruna, J. and Mallat, S. (2013). Invariant scattering convo-
lution networks. IEEE transactions on pattern analy-
sis and machine intelligence, 35(8):1872–1886.
Dubey, A. (2018). Symmetric completion of deformable reg-
istration via bi-residual inversion. PhD thesis, PhD
thesis). Duke University, Durham, NC, USA.
Dubey, A., Iliopoulos, A.-S., Sun, X., Yin, F.-F., and Ren, L.
(2018). Iterative inversion of deformation vector fields
with feedback control. Medical physics, 45(7):3147–
3160.
Gretton, A., Borgwardt, K. M., Rasch, M. J., Sch
¨
olkopf, B.,
and Smola, A. (2012). A kernel two-sample test. Jour-
nal of Machine Learning Research, 13(Mar):723–773.
Iliopoulos, A.-S., Dubey, A., and Sun, X. (2019). “idvf“:
Iterative inversion of deformation vector field with
adaptive bi-residual feedback control. Journal of
Open Source Software, 4(35):1076.
Ilse, M., Tomczak, J. M., Louizos, C., and Welling, M.
(2019). Diva: Domain invariant variational autoen-
coders. arXiv preprint arXiv:1905.10427.
Irvin, J., Rajpurkar, P., Ko, M., Yu, Y., Ciurea-Ilcus, S.,
Chute, C., Marklund, H., Haghgoo, B., Ball, R., Sh-
panskaya, K., et al. (2019). Chexpert: A large chest
radiograph dataset with uncertainty labels and expert
comparison. In Proceedings of the AAAI Conference
on Artificial Intelligence, volume 33, pages 590–597.
Muandet, K., Balduzzi, D., and Sch
¨
olkopf, B. (2013). Do-
main generalization via invariant feature representa-
tion. In International Conference on Machine Learn-
ing, pages 10–18.
Peng, Y., Wang, X., Lu, L., Bagheri, M., Summers, R.,
and Lu, Z. (2018). Negbio: a high-performance tool
for negation and uncertainty detection in radiology re-
ports. AMIA Summits on Translational Science Pro-
ceedings, 2018:188.
Pooch, E. H., Ballester, P. L., and Barros, R. C. (2019).
Can we trust deep learning models diagnosis? the im-
pact of domain shift in chest radiograph classification.
arXiv preprint arXiv:1909.01940.
Selvaraju, R. R., Cogswell, M., Das, A., Vedantam, R.,
Parikh, D., and Batra, D. (2017). Grad-cam: Visual
explanations from deep networks via gradient-based
localization. In Proceedings of the IEEE international
conference on computer vision, pages 618–626.
Tang, Y.-X., Tang, Y.-B., Peng, Y., Yan, K., Bagheri, M.,
Redd, B. A., Brandon, C. J., Lu, Z., Han, M., Xiao,
J., et al. (2020). Automated abnormality classification
of chest radiographs using deep convolutional neural
networks. NPJ Digital Medicine, 3(1):1–8.
Wang, X., Peng, Y., Lu, L., Lu, Z., Bagheri, M., and Sum-
mers, R. M. (2017). Chestx-ray8: Hospital-scale chest
x-ray database and benchmarks on weakly-supervised
classification and localization of common thorax dis-
eases. In Proceedings of the IEEE conference on
computer vision and pattern recognition, pages 2097–
2106.
Yao, L., Prosky, J., Covington, B., and Lyman, K.
(2019). A strong baseline for domain adaptation and
generalization in medical imaging. arXiv preprint
arXiv:1904.01638.
Zaremba, W., Gretton, A., and Blaschko, M. (2013). B-test:
A non-parametric, low variance kernel two-sample
test. In Advances in neural information processing
systems, pages 755–763.
Zhu, J.-Y., Park, T., Isola, P., and Efros, A. A. (2017).
Unpaired image-to-image translation using cycle-
consistent adversarial networks. In Proceedings of
the IEEE international conference on computer vi-
sion, pages 2223–2232.
BIOIMAGING 2021 - 8th International Conference on Bioimaging
72