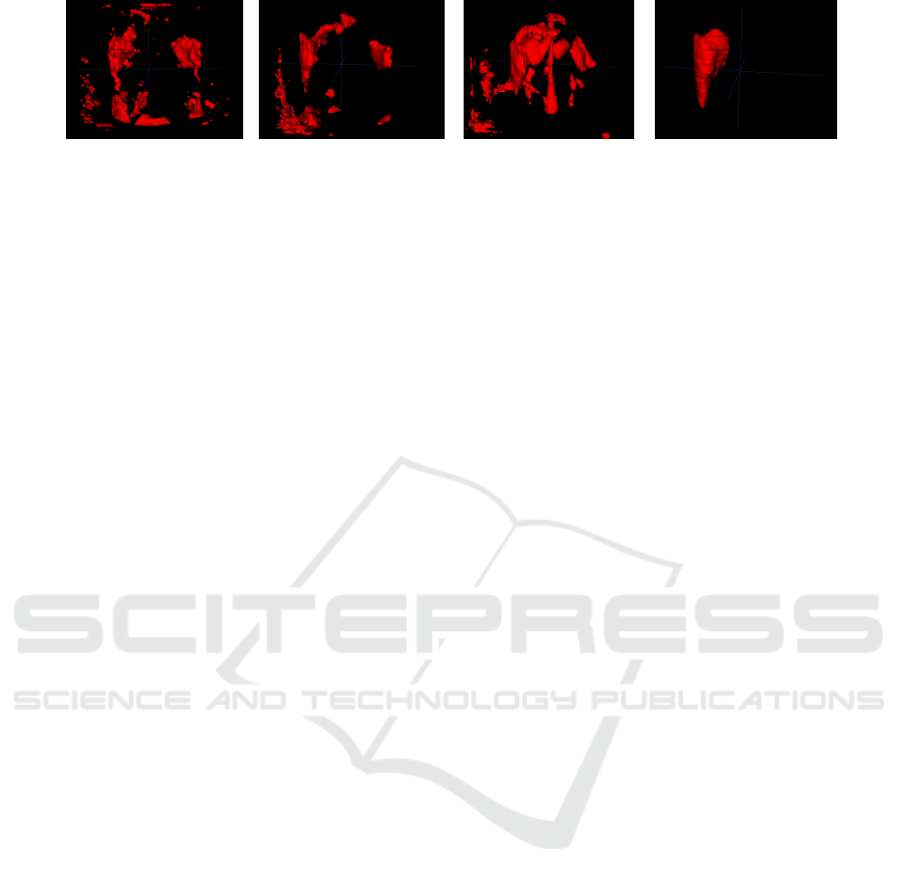
Figure 10: Exemplary segmentation results from each architecture on the situs inversus setting. Left to right: U-Net, ACNN,
IE
2
D, IRE
3
D.
that the incorporation of Oktay et al.’s regularization
scheme yields smoother results and fewer outliers for
both the ACNN and our proposed IRE
3
D architecture,
which is reflected by the resulting DSC scores. How-
ever, shape regularization does not seem to reduce the
maximal outlier distance.
5 CONCLUSION
In this work we investigated the one-shot segmen-
tation capability of a standard U-Net and examined
how incorporating anatomical priors may change the
outcome on the example of liver segmentation from
CT. The U-Net delivers promising results in settings,
where the position of the liver shows low variation,
which is often the case when training and testing data
sets that come from the same source. We also ob-
served, that in cases of different data sources, where
the liver position may change drastically, the U-Net
shows strong weaknesses due to overfitting of the po-
sition, and that incorporating anatomical priors may
improve the segmentation results. We proposed a new
architecture, that incorporates anatomical information
in 2 ways and achieved promising and competitive re-
sults, particularly in settings of different data sources.
We demonstrated this on the example of the situs in-
versus setting, in which we achieved best results for
most cases regarding DSC and Hausdorff distance.
This was specifically notable in the more challenging
cases. In the future, we intend to further examine how
little data may be feasible for the U-Net to reach good
segmentation results in a constrained setting. We also
aim to extend our architecture for multi-organ one-
/few-shot segmentation tasks.
REFERENCES
Clark, K., Vendt, B., et al. (2013). The cancer imag-
ing archive (tcia): maintaining and operating a pub-
lic information repository. Journal of digital imaging,
26(6):1045–1057.
Dalca, A. V., Guttag, J., and Sabuncu, M. R. (2018).
Anatomical Priors in Convolutional Networks for Un-
supervised Biomedical Segmentation. In Proceedings
of the IEEE Conference on Computer Vision and Pat-
tern Recognition, pages 9290–9299.
Dong, N. and Xing, E. P. (2018). Few-shot semantic seg-
mentation with prototype learning. In BMVC, vol-
ume 3, page 4.
Gibson, E., Giganti, F., et al. (2018). Automatic multi-organ
segmentation on abdominal ct with dense v-networks.
IEEE transactions on medical imaging, 37(8):1822–
1834.
Girdhar, R., Fouhey, D. F., et al. (2016). Learning a Pre-
dictable and Generative Vector Representation for Ob-
jects. In European Conference on Computer Vision,
pages 484–499. Springer.
Isensee, F., Kickingereder, P., et al. (2018). No new-net. In
International MICCAI Brainlesion Workshop, pages
234–244. Springer.
Koch, G., Zemel, R., and Salakhutdinov, R. (2015).
Siamese neural networks for one-shot image recogni-
tion. In ICML Deep Learning Workshop, volume 2.
Landman, B., Xu, Z., et al. (2015). MICCAI multi-atlas la-
beling beyond the cranial vault - workshop and chal-
lenge. https://doi.org/10.7303/syn3193805.
Michaelis, C., Bethge, M., and Ecker, A. (2018). One-shot
segmentation in clutter. In International Conference
on Machine Learning, pages 3546–3555.
Oktay, O., Ferrante, E., et al. (2018). Anatomically Con-
strained Neural Networks (ACNNs): Application to
Cardiac Image Enhancement and Segmentation. IEEE
Transactions on Medical Imaging, 37(2):384–395.
Pham, D. D., Dovletov, G., Warwas, S., Landgraeber, S.,
J
¨
ager, M., and Pauli, J. (2019). Deep learning with
anatomical priors: imitating enhanced autoencoders in
latent space for improved pelvic bone segmentation in
mri. In 2019 IEEE 16th International Symposium on
Biomedical Imaging (ISBI 2019), pages 1166–1169.
IEEE.
Ravishankar, H., Venkataramani, R., et al. (2017). Learning
and Incorporating Shape Models for Semantic Seg-
mentation. In International Conference on Medical
Image Computing and Computer-Assisted Interven-
tion, pages 203–211. Springer.
Ronneberger, O., Fischer, P., and Brox, T. (2015). U-net:
Convolutional Networks for Biomedical Image Seg-
mentation. In International Conference on Medical
Image Computing and Computer-Assisted Interven-
tion, pages 234–241. Springer.
Roth, H. R., Farag, A., et al. (2016). Data From Pancreas-
CT. The Cancer Imaging Archive. http://doi.org/10.
7937/K9/TCIA.2016.tNB1kqBU.
BIOIMAGING 2021 - 8th International Conference on Bioimaging
180