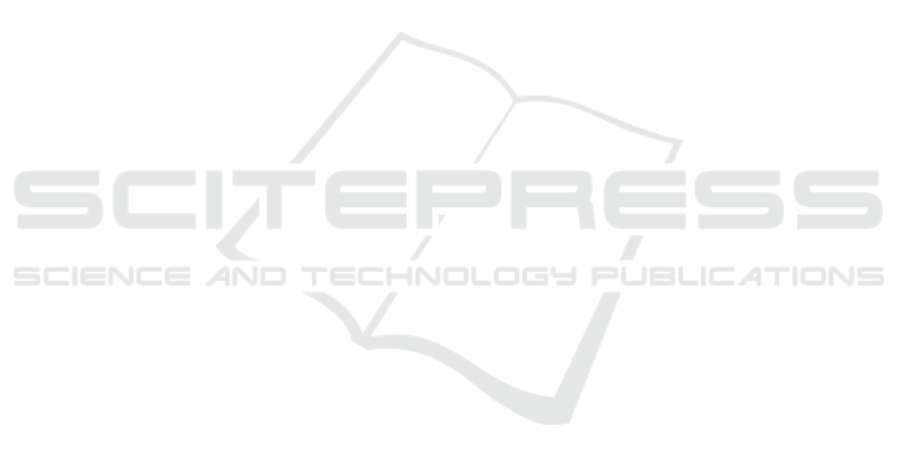
p value increases, specially in the low slice results
(Table 5). CE and Focal BCE do not converge as of-
ten as the proposed losses in tested scenarios. Even
though, their best IoU in Table 5 is comparable with
power functions. Models trained with p = 1.5 per-
form better than classical Jaccard in both slices.
5 CONCLUSIONS
In this work, we propose generalized loss functions to
perform semantic segmentation by introducing power
Jaccard. We evaluated it in different types of images
such as gray-scale, RGB and point cloud projections
in binary and multiclass segmentation tasks. Obtained
results demonstrate that the use of power losses out-
performs classical losses such as cross-entropy, Jac-
card and Dice score.
In order to evaluate the stability of the models, we
repeated several times the same configuration and we
stated that the use of power losses helps to increase
the rate of convergence. This is useful in deep learn-
ing models where the stability of the models is critical
and it is strongly associated with the randomness of
the initialization parameters.
We performed several experiments with differ-
ent types of images, different dataset of segmenta-
tion task, demonstrating that the advantage of power
losses is not an isolated case.
Additionally, to the results presented in this pa-
per, we had conducted some experiments by includ-
ing a power term in the classical Dice score in the
same spirit of our proposal. Obtained results demon-
strate that the use of p equal to two also improves the
performance compared against the classical Dice loss
in several scenarios. Accordingly, for future work,
we will investigate a generalization of power terms
on loss functions for semantic segmentation and a
method to estimate the best value of p in different sce-
narios.
ACKNOWLEDGEMENTS
This work was partially funded by REPLICA FUI 24
project and ARMINES.
REFERENCES
Alpaydin, E. (1997). Voting over multiple condensed near-
est neighbors. In Lazy learning, pages 115–132.
Springer.
Badrinarayanan, V., Kendall, A., and Cipolla, R. (2017).
Segnet: A deep convolutional encoder-decoder ar-
chitecture for image segmentation. IEEE transac-
tions on pattern analysis and machine intelligence,
39(12):2481–2495.
Caliv
´
a, F., Iriondo, C., Martinez, A. M., Majumdar, S.,
and Pedoia, V. (2019). Distance map loss penalty
term for semantic segmentation. arXiv preprint
arXiv:1908.03679.
Cha, S.-H. (2007). Comprehensive survey on dis-
tance/similarity measures between probability density
functions. City, 1(2):1.
Cordts, M., Omran, M., Ramos, S., Rehfeld, T., Enzweiler,
M., Benenson, R., Franke, U., Roth, S., and Schiele,
B. (2016). The cityscapes dataset for semantic urban
scene understanding. In Proc. of the IEEE Conference
on Computer Vision and Pattern Recognition (CVPR).
Decenci
`
ere, E., Velasco-Forero, S., Min, F., Chen, J., Bur-
din, H., Gauthier, G., La
¨
y, B., Bornschloegl, T., and
Baldeweck, T. (2018). Dealing with topological infor-
mation within a fully convolutional neural network. In
International Conference on Advanced Concepts for
Intelligent Vision Systems, pages 462–471. Springer.
Deza, M. M. and Deza, E. (2009). Encyclopedia of dis-
tances. In Encyclopedia of distances, pages 1–583.
Springer.
Diakogiannis, F. I., Waldner, F., Caccetta, P., and Wu, C.
(2020). Resunet-a: a deep learning framework for
semantic segmentation of remotely sensed data. IS-
PRS Journal of Photogrammetry and Remote Sensing,
162:94–114.
Hern
´
andez, J. and Marcotegui, B. (2009). Point cloud seg-
mentation towards urban ground modeling. In 2009
Joint Urban Remote Sensing Event, pages 1–5. IEEE.
Jaccard, P. (1901). Distribution de la flore alpine dans le
bassin des dranses et dans quelques r
´
egions voisines.
Bull Soc Vaudoise Sci Nat, 37:241–272.
Johnson, J. M. and Khoshgoftaar, T. M. (2019). Survey on
deep learning with class imbalance. Journal of Big
Data, 6(1):27.
Jun, M. (2020). Segmentation loss odyssey. arXiv preprint
arXiv:2005.13449.
Kervadec, H., Bouchtiba, J., Desrosiers, C., Granger, E.,
Dolz, J., and Ayed, I. B. (2018). Boundary loss
for highly unbalanced segmentation. arXiv preprint
arXiv:1812.07032.
Ku, T., Veltkamp, R. C., Boom, B., Duque-Arias, D.,
Velasco-Forero, S., Deschaud, J.-E., Goulette, F.,
Marcotegui, B., Ortega, S., Trujillo, A., et al. (2020).
Shrec 2020 track: 3d point cloud semantic segmenta-
tion for street scenes. Computers & Graphics.
Lin, T.-Y., Goyal, P., Girshick, R., He, K., and Doll
´
ar, P.
(2017). Focal loss for dense object detection. In
Proceedings of the IEEE international conference on
computer vision, pages 2980–2988.
Martire, I., da Silva, P., Plastino, A., Fabris, F., and Fre-
itas, A. A. (2017). A novel probabilistic jaccard dis-
tance measure for classification of sparse and uncer-
tain data. In de Faria Paiva, E. R., Merschmann,
L., and Cerri, R., editors, 5th Brazilian Symposium
On Power Jaccard Losses for Semantic Segmentation
567