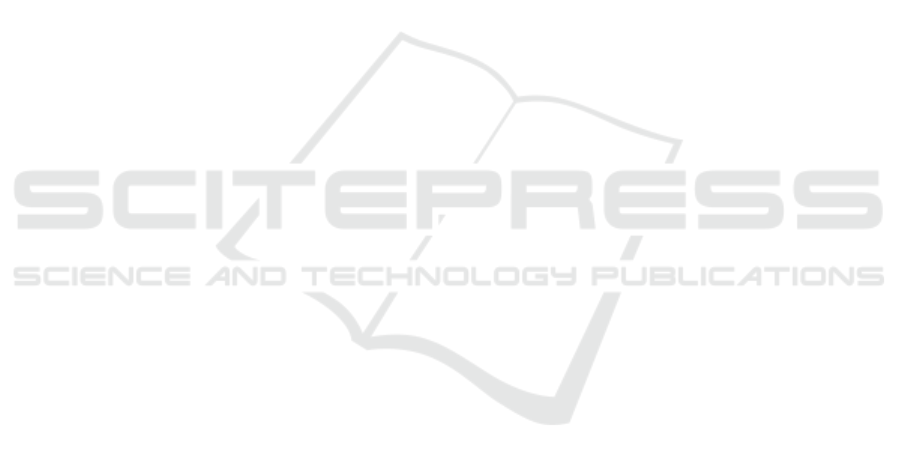
ing a suitable measure. The performance of similarity
measures may vary depending on different datasets.
In this paper, we studied a quantitative comparison for
different similarity measures on UT images of ingots.
The aim of this study was to clarify which similarity
measures are more appropriate and applicable when
searching for specific ultrasonic patterns. Further, we
conducted interviews with domain experts in the anal-
ysis of UT indications images comparison and used
this feedback to define a ground truth for our eval-
uation. We provided a discussion and demonstrated
the possible insights enabled by our approach and its
potential to support production data exploration.
Future work includes investigation of process data
corresponding to groups of similar ingots and batches,
and potentially discovering key influential parameters
in the process data. As future work, we also want
to include advanced multidimensional data visualiza-
tions, to support pattern detection and parameter cor-
relation. Furthermore, automatic classification of cer-
tain quality patterns, based on interactively provided
expert examples, is considered an interesting future
extension of an existing visual analytics solution.
ACKNOWLEDGEMENTS
This research work is done by Pro2Future and AMAG
Austria Metall AG. Pro2Future is funded within the
Austrian COMET Program-Competence Centers for
Excellent Technologies under the auspices of the Aus-
trian Federal Ministry of Transport, Innovation and
Technology, the Austrian Federal Ministry for Digital
and Economic Affairs and of the Provinces of Upper
Austria and Styria. COMET is managed by the Aus-
trian Research Promotion Agency FFG.
REFERENCES
Bazan, E., Dokl
´
adal, P., and Dokladalova, E. (2019). Quan-
titative analysis of similarity measures of distribu-
tions.
Behrisch, M., Korkmaz, F., Shao, L., and Schreck, T.
(2014). Feedback-driven interactive exploration of
large multidimensional data supported by visual clas-
sifier. In 2014 IEEE Conference on Visual Analytics
Science and Technology (VAST), pages 43–52. IEEE.
Bokeh Development Team (2018). Bokeh: Python library
for interactive visualization.
Cha, S.-H. (2007). Comprehensive survey on dis-
tance/similarity measures between probability density
functions. City, 1(2):1.
Deza, M.-M. and Deza, E. (2006). Dictionary of distances.
Elsevier.
Hern
´
andez-Rivera, E., Coleman, S. P., and Tschopp, M. A.
(2017). Using similarity metrics to quantify differ-
ences in high-throughput data sets: application to x-
ray diffraction patterns. ACS combinatorial science,
19(1):25–36.
Jekic, N., Mutlu, B., Faschang, M., Neubert, S., Thalmann,
S., and Schreck, T. (2019). Visual analysis of alu-
minum production data with tightly linked views. In
EuroVis (Posters), pages 49–51.
Jo, J., Huh, J., Park, J., Kim, B., and Seo, J. (2014). Live-
gantt: Interactively visualizing a large manufactur-
ing schedule. IEEE transactions on visualization and
computer graphics, 20(12):2329–2338.
Lee, J., Kao, H.-A., Yang, S., et al. (2014). Service innova-
tion and smart analytics for industry 4.0 and big data
environment. Procedia Cirp, 16(1):3–8.
Lee, J., Lapira, E., Bagheri, B., and Kao, H.-a. (2013). Re-
cent advances and trends in predictive manufacturing
systems in big data environment. Manufacturing let-
ters, 1(1):38–41.
Liu, S., Cui, W., Wu, Y., and Liu, M. (2014). A survey on
information visualization: recent advances and chal-
lenges. The Visual Computer, 30(12):1373–1393.
Matkovic, K., Hauser, H., Sainitzer, R., and Groller, M. E.
(2002). Process visualization with levels of detail. In
IEEE Symposium on Information Visualization, 2002.
INFOVIS 2002., pages 67–70. IEEE.
McKinney, W. (2012). Python for data analysis: Data
wrangling with Pandas, NumPy, and IPython. ”
O’Reilly Media, Inc.”.
Scherer, M., Bernard, J., and Schreck, T. (2011). Retrieval
and exploratory search in multivariate research data
repositories using regressional features. In Proceed-
ings of the 11th annual international ACM/IEEE joint
conference on Digital libraries, pages 363–372.
Shao, L., Schleicher, T., Behrisch, M., Schreck, T., Sipiran,
I., and Keim, D. A. (2016). Guiding the exploration of
scatter plot data using motif-based interest measures.
Journal of Visual Languages & Computing, 36:1–12.
Shirkhorshidi, A. S., Aghabozorgi, S., and Wah, T. Y.
(2015). A comparison study on similarity and dissim-
ilarity measures in clustering continuous data. PloS
one, 10(12):e0144059.
Soban, D., Thornhill, D., Salunkhe, S., and Long, A.
(2016). Visual analytics as an enabler for manufactur-
ing process decision-making. Procedia Cirp, 56:209–
214.
Sun, D., Huang, R., Chen, Y., Wang, Y., Zeng, J., Yuan,
M., Pong, T.-C., and Qu, H. (2019). Planningvis: A
visual analytics approach to production planning in
smart factories. IEEE transactions on visualization
and computer graphics, 26(1):579–589.
Suschnigg, J., Ziessler, F., Brillinger, M., Vukovic, M.,
Mangler, J., Schreck, T., and Thalmann, S. (2020). In-
dustrial production process improvement by a process
engine visual analytics dashboard. In Proceedings of
the 53rd Hawaii International Conference on System
Sciences, pages 1320–1329.
Thalmann, S., Mangler, J., Schreck, T., Huemer, C., Streit,
M., Pauker, F., Weichhart, G., Schulte, S., Kittl, C.,
Similarity Measures for Visual Comparison and Retrieval of Test Data in Aluminum Production
217