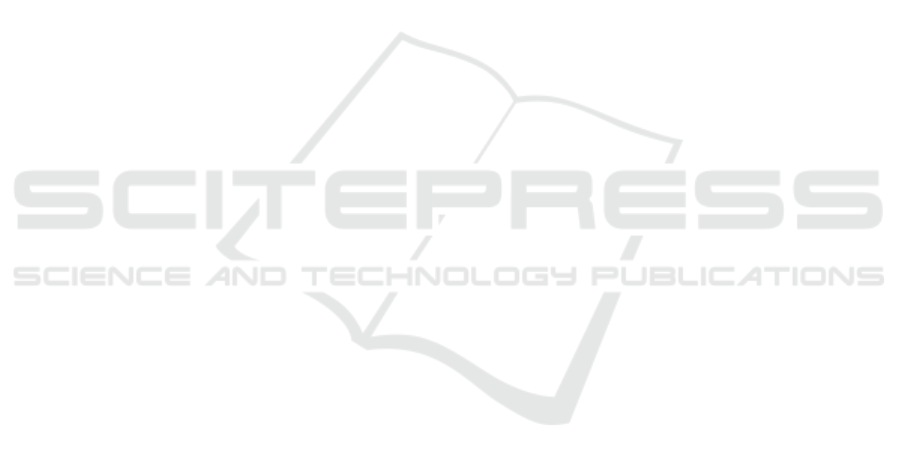
Everitt, B. (2005). Classification and Regression Trees.
Chapman & Hall.
Fan, R.-E., Chen, P.-H., and Lin, C.-J. (2005). Working
set selection using second order information for train-
ing support vector machines. J. Mach. Learn. Res.,
6:1889–1918.
Fang, H., Harris, S. C., Liu, Z., Zhou, G., Zhang, G., Xu, J.,
Rosario, L., Howard, P. C., and Tong, W. (2016). Fda
drug labeling: Rich resources to facilitate precision
medicine, drug safety, and regulatory science. Drug
discovery today, 21(10):1566–1570.
FDASIA (2012). (Accessed on 5/21/2020).
Fiks, A. G., Scheindlin, B., and Shone, L. (2016). 30th
anniversary of pediatric research in office settings
(pros): An invitation to become engaged. Pediatrics,
138(3):e20161126.
Fung, K. W., Jao, C. S., and Demner-Fushman, D. (2013).
Extracting drug indication information from struc-
tured product labels using natural language process-
ing. Journal of the American Medical Informatics As-
sociation : JAMIA, 20(3):482–488.
Gnanasakthy, A., Barrett, A., Evans, E., D’Alessio, D., and
Romano, C. D. (2019). A review of patient-reported
outcomes labeling for oncology drugs approved by
the fda and the ema (2012-2016). Value in Health,
22(2):203–209.
Green, D. J., Sun, H., Burnham, J., Liu, X. I., van den
Anker, J., Temeck, J., Yao, L., McCune, S. K., and
Burckart, G. J. (2019). Surrogate endpoints in pedi-
atric studies submitted to the us fda. Clinical Phar-
macology & Therapeutics, 105(3):555–557.
HL7 Standards (2019). (Accessed on 3/14/2019).
Hoon, D., Taylor, M. T., Kapadia, P., Gerhard, T., Strom,
B. L., and Horton, D. B. (2019). Trends in off-label
drug use in ambulatory settings: 2006–2015. Pedi-
atrics, 144(4).
Hu, L.-Y., Huang, M.-W., Ke, S.-W., and Tsai, C.-F.
(2016). The distance function effect on k-nearest
neighbor classification for medical datasets. Springer-
Plus, 5(1):1304–1304.
Ito, S. (2017). Drugs for children. Clinical Pharmacology
& Therapeutics, 101(6):704–706.
Khare, R., Wei, C.-H., and Lu, Z. (2014). Automatic ex-
traction of drug indications from fda drug labels. In
AMIA Annual Symposium Proceedings, volume 2014,
page 787. American Medical Informatics Association.
Kuhn, M., Letunic, I., Jensen, L. J., and Bork, P. (2016).
The sider database of drugs and side effects. Nucleic
acids research, 44(D1):D1075–D1079.
Li, Q., Deleger, L., Lingren, T., Zhai, H., Kaiser, M.,
Stoutenborough, L., Jegga, A., Cohen, K., and Solti,
I. (2013). Mining fda drug labels for medical condi-
tions. BMC medical informatics and decision making,
13:53.
Lowenthal, E. and Fiks, A. G. (2016). Protecting children
through research. Pediatrics, 138(4):e20162150.
lxml (2019). (Accessed on 2/18/2019).
Mehta, D., Uber, R., Ingle, T., Li, C., Liu, Z., Thakkar,
S., Ning, B., Wu, L., Yang, J., Harris, S., et al.
(2020). Study of pharmacogenomic information in
fda-approved drug labeling to facilitate application of
precision medicine. Drug Discovery Today.
Mulugeta, L. Y., Yao, L., Mould, D., Jacobs, B., Florian, J.,
Smith, B., Sinha, V., and Barrett, J. S. (2018). Lever-
aging big data in pediatric development programs:
Proceedings from the 2016 american college of clini-
cal pharmacology annual meeting symposium. Clini-
cal Pharmacology & Therapeutics, 104(1):81–87.
N
´
ev
´
eol, A. and Lu, Z. (2010). Automatic integration of
drug indications from multiple health resources. In
Proceedings of the 1st ACM International Health In-
formatics Symposium, IHI’10, pages 666–673, New
York, NY, USA. Association for Computing Machin-
ery.
Pandey, A., Kreimeyer, K., Foster, M., Dang, O., Ly, T.,
Wang, W., Forshee, R., and Botsis, T. (2019). Ad-
verse event extraction from structured product labels
using the event-based text-mining of health electronic
records (ether) system. Health informatics journal,
25(4):1232–1243.
Prospective and retrospective cohort studies (2019). (Ac-
cessed on 11/23/2019).
Refaeilzadeh, P., Tang, L., and Liu, H. (2009). Cross-
validation. Encyclopedia of Database Systems, pages
532–538.
Rodriguez, L. M. and Fushman, D. D. (2015). Automatic
classification of structured product labels for preg-
nancy risk drug categories, a machine learning ap-
proach. In AMIA Annual Symposium Proceedings,
volume 2015, page 1093. American Medical Infor-
matics Association.
Scikit-Learn (2019). (Accessed on 4/19/2019).
Structured product labeling (2019). (Accessed on
3/15/2019).
Tiftikci, M.,
¨
Ozg
¨
ur, A., He, Y., and Hur, J. (2019). Machine
learning-based identification and rule-based normal-
ization of adverse drug reactions in drug labels. BMC
bioinformatics, 20(21):1–9.
U.S. Congress. Best Pharmaceuticals for Children Act
Amending Section 505A of the Federal Food, Drug &
Cosmetic Act (Public Law 107-109). (2002) (2002).
(Accessed on 5/4/2020).
U.S. Congress. Pediatric Research Equity Act amending
Section 505B of the Federal Food, Drug & Cosmetic
Act (Public Law 108-155). (2003) (2003). (Accessed
on 5/4/2020).
U.S. Food and Drug Administration. New pediatric labeling
information dataset (2020). (Accessed on 5/20/2020).
Mining Biomedical Texts for Pediatric Information
71