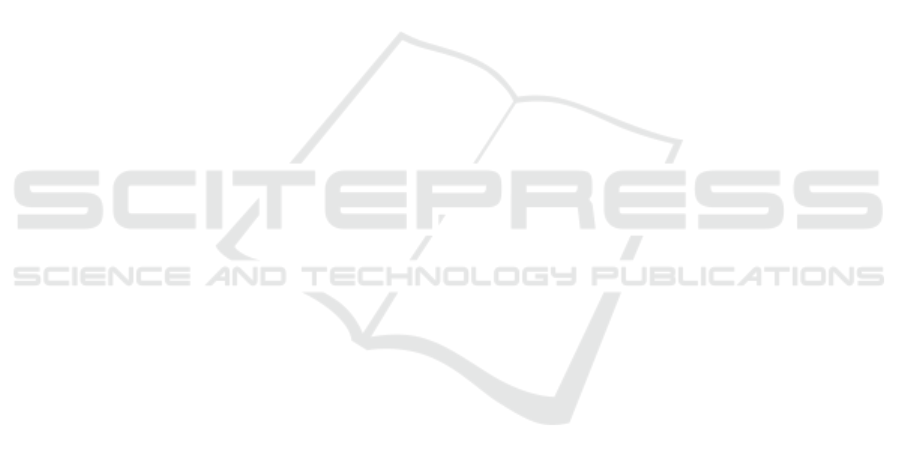
Schmidt, T. G., and Chen, G.-H., editors, Medical
Imaging 2018: Physics of Medical Imaging, volume
10573, pages 1333 – 1340. International Society for
Optics and Photonics, SPIE.
Caldwell, C. B. and Yaffe, M. J. (1990). Development of an
anthropomorphic breast phantom. Medical physics,
17(2):273–280.
Carton, A.-K., Bakic, P., Ullberg, C., Derand, H., and Maid-
ment, A. D. (2011). Development of a physical 3d
anthropomorphic breast phantom. Medical physics,
38(2):891–896.
Chatfield, K., Simonyan, K., Vedaldi, A., and Zisserman,
A. (2014). Return of the devil in the details: Delving
deep into convolutional nets. CoRR, abs/1405.3531.
Cheng, H.-D., Shi, X., Min, R., Hu, L., Cai, X., and Du, H.
(2006). Approaches for automated detection and clas-
sification of masses in mammograms. Pattern recog-
nition, 39(4):646–668.
Chui, J. H., Pokrajac, D. D., Maidment, A. D. A., and Ba-
kic, P. R. (2012). Roadmap for efficient paralleliza-
tion of breast anatomy simulation. In Pelc, N. J.,
Nishikawa, R. M., and Whiting, B. R., editors, Med-
ical Imaging 2012: Physics of Medical Imaging, vol-
ume 8313, pages 1369 – 1378. International Society
for Optics and Photonics, SPIE.
Elangovan, P., Mackenzie, A., Dance, D. R., Young, K. C.,
Cooke, V., Wilkinson, L., Given-Wilson, R. M., Wal-
lis, M. G., and Wells, K. (2017). Design and validation
of realistic breast models for use in multiple alterna-
tive forced choice virtual clinical trials. Physics in
Medicine & Biology, 62(7):2778.
Feng, S. S. J. and Sechopoulos, I. (2012). Clinical digital
breast tomosynthesis system: dosimetric characteriza-
tion. Radiology, 263(1):35–42.
Hamarneh, G. and Jassi, P. (2010). Vascusynth: Simu-
lating vascular trees for generating volumetric image
data with ground-truth segmentation and tree anal-
ysis. Computerized medical imaging and graphics,
34(8):605–616.
Hubbell, J. H. and Seltzer, S. M. (1995). Tables of x-
ray mass attenuation coefficients and mass energy-
absorption coefficients 1 kev to 20 mev for elements
z= 1 to 92 and 48 additional substances of dosimetric
interest. Technical report, National Inst. of Standards
and Technology-PL, Gaithersburg, MD (United . . . .
Lashgari, E., Liang, D., and Maoz, U. (2020). Data aug-
mentation for deep-learning-based electroencephalog-
raphy. Journal of Neuroscience Methods, page
108885.
Li, C. M., Segars, W. P., Tourassi, G. D., Boone, J. M., and
Dobbins III, J. T. (2009). Methodology for generat-
ing a 3d computerized breast phantom from empirical
data. Medical physics, 36(7):3122–3131.
Maidment, A. D. (2014). Virtual clinical trials for the as-
sessment of novel breast screening modalities. In
International Workshop on Digital Mammography,
pages 1–8. Springer.
Norman, B., Pedoia, V., and Majumdar, S. (2018). Use of
2d u-net convolutional neural networks for automated
cartilage and meniscus segmentation of knee mr imag-
ing data to determine relaxometry and morphometry.
Radiology, 288(1):177–185.
Oliveira, W. d. S. (2017). Consenso de segmentac¸
˜
oes de
imagens usando classificac¸
˜
ao de padr
˜
oes. PhD thesis,
Universidade Federal de Pernambuco.
Paul, S. (2018). Learn how to train u-net on your
dataset. https://medium.com/coinmonks/learn-how-
to-train-u-net-on-your-dataset-8e3f89fbd623.
Pokrajac, D. D., Maidment, A. D., and Bakic, P. R. (2012).
Optimized generation of high resolution breast an-
thropomorphic software phantoms. Medical physics,
39(4):2290–2302.
Ronneberger, O., Fischer, P., and Brox, T. (2015). U-net:
Convolutional networks for biomedical image seg-
mentation. In International Conference on Medical
image computing and computer-assisted intervention,
pages 234–241. Springer.
Rui, T., Zou, J., Zhou, Y., Fei, J., and Yang, C. (2018).
Convolutional neural network feature maps selection
based on lda. Multimedia Tools and Applications,
77(9):10635–10649.
Sevastopolsky, A. (2017). Optic disc and cup segmentation
methods for glaucoma detection with modification of
u-net convolutional neural network. Pattern Recogni-
tion and Image Analysis, 27(3):618–624.
Siddon, R. L. (1985). Fast calculation of the exact radio-
logical path for a three-dimensional ct array. Medical
physics, 12(2):252–255.
Tice, J. and Feldman, M. (2008). Full-field digital mam-
mography compared with screen-film mammography
in the detection of breast cancer: Rays of light through
dmist or more fog? Breast cancer research and treat-
ment, 107:157–65.
Tunc¸ay, A. H. and Akduman, I. (2014). Realistic microwave
breast models through t1-weighted 3-d mri data. IEEE
Transactions on Biomedical Engineering, 62(2):688–
698.
Valverde, S., Cabezas, M., Roura, E., Gonz
´
alez-Vill
`
a,
S., Pareto, D., Vilanova, J. C., Rami
´
o-Torrent
`
a, L.,
Rovira, A., Oliver, A., and Llad
´
o, X. (2017). Improv-
ing automated multiple sclerosis lesion segmentation
with a cascaded 3d convolutional neural network ap-
proach. CoRR, abs/1702.04869.
Vedantham, S., Karellas, A., Vijayaraghavan, G. R., and
Kopans, D. B. (2015). Digital breast tomosynthesis:
State of the art. Radiology, 277(3):663–684. PMID:
26599926.
Zhang, C., Bakic, P., and Maidment, A. (2008). Develop-
ment of an anthropomorphic breast software phantom
based on region growing algorithm - art. no. 69180v.
Proc SPIE, 6918.
Zhixuhao (2016). Implementation of deep
learning framework – unet, using keras.
https://github.com/zhixuhao/unet.
Automatic Segmentation of Mammary Tissue using Computer Simulations of Breast Phantoms and Deep-learning Techniques
259