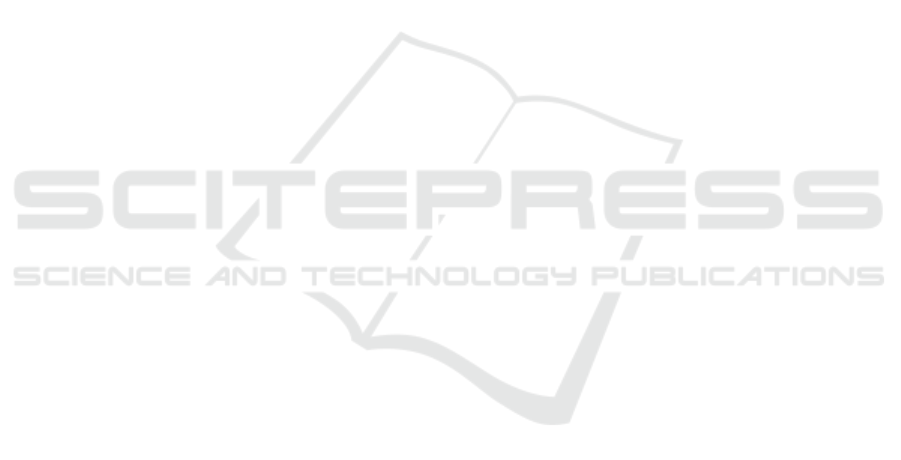
frastructure for: (i) advancements in machine learn-
ing algorithms for multi-view and multi-domain oph-
thalmic applications, (ii) improvements in generaliz-
ability and translations into clinical settings, and (iii)
enhanced understanding of variations in ophthalmic
disease prognosis.
As a research databank with a unique infrastruc-
ture, I-ODA will continue to grow in imaging and pa-
tient metadata. While the limitations in annotations
are understandable, machine learning applications de-
veloped on data from I-ODA will allow new discov-
eries in computer vision, specifically in the medical
imaging field, and in new applications for classifi-
cation and progression of ophthalmic diseases. Ad-
ditionally, I-ODA can also serve with multiple ef-
forts in validating current algorithms that have shown
promise in more controlled datasets with less diverse
domains and patient population.
ACKNOWLEDGEMENT
This work is supported in part by NSF under grants
III-1763325, III-1909323, SaTC-1930941, BrightFo-
cus Foundation Grant M2019155, and Core Grant for
Vision Research (2P30 EY001792 41), Department
of Ophthalmology and Visual Sciences, University of
Illinois at Chicago.
REFERENCES
Almazroa, A., Alodhayb, S., Osman, E., Ramadan, E.,
Hummadi, M., Dlaim, M., Alkatee, M., Raahemi-
far, K., and Lakshminarayanan, V. (2018). Reti-
nal fundus images for glaucoma analysis: the riga
dataset. In Medical Imaging 2018: Imaging Informat-
ics for Healthcare, Research, and Applications, vol-
ume 10579, page 105790B. International Society for
Optics and Photonics.
Burlina, P. M., Joshi, N., Pacheco, K. D., Freund, D. E.,
Kong, J., and Bressler, N. M. (2018). Use of deep
learning for detailed severity characterization and es-
timation of 5-year risk among patients with age-
related macular degeneration. JAMA ophthalmology,
136(12):1359–1366.
Burlina, P. M., Joshi, N., Pekala, M., Pacheco, K. D., Fre-
und, D. E., and Bressler, N. M. (2017). Automated
grading of age-related macular degeneration from
color fundus images using deep convolutional neural
networks. JAMA ophthalmology, 135(11):1170–1176.
Decenci
`
ere, E., Zhang, X., Cazuguel, G., Lay, B., Coch-
ener, B., Trone, C., Gain, P., Ordonez, R., Massin, P.,
Erginay, A., et al. (2014). Feedback on a publicly dis-
tributed image database: the messidor database. Im-
age Analysis & Stereology, 33(3):231–234.
Fu, H., Cheng, J., Xu, Y., Zhang, C., Wong, D. W. K., Liu,
J., and Cao, X. (2018). Disc-aware ensemble network
for glaucoma screening from fundus image. IEEE
transactions on medical imaging, 37(11):2493–2501.
Fumero, F., Alay
´
on, S., Sanchez, J. L., Sigut, J., and
Gonzalez-Hernandez, M. (2011). Rim-one: An open
retinal image database for optic nerve evaluation.
In 2011 24th international symposium on computer-
based medical systems (CBMS), pages 1–6. IEEE.
Gargeya, R. and Leng, T. (2017). Automated identification
of diabetic retinopathy using deep learning. Ophthal-
mology, 124(7):962–969.
Grewal, P. S., Oloumi, F., Rubin, U., and Tennant, M. T.
(2018). Deep learning in ophthalmology: a review.
Canadian Journal of Ophthalmology, 53(4):309–313.
Gulshan, V., Peng, L., Coram, M., Stumpe, M. C., Wu,
D., Narayanaswamy, A., Venugopalan, S., Widner, K.,
Madams, T., Cuadros, J., et al. (2016). Development
and validation of a deep learning algorithm for de-
tection of diabetic retinopathy in retinal fundus pho-
tographs. Jama, 316(22):2402–2410.
Lu, W., Tong, Y., Yu, Y., Xing, Y., Chen, C., and Shen, Y.
(2018). Applications of artificial intelligence in oph-
thalmology: general overview. Journal of ophthalmol-
ogy, 2018.
Medeiros, F. A., Jammal, A. A., and Thompson, A. C.
(2019). From machine to machine: an oct-trained
deep learning algorithm for objective quantification of
glaucomatous damage in fundus photographs. Oph-
thalmology, 126(4):513–521.
Mojab, N., Noroozi, V., Yi, D., Nallabothula, M. P., Aleem,
A., Yu, P. S., and Hallak, J. A. (2020). Real-world
multi-domain data applications for generalizations to
clinical settings. arXiv preprint arXiv:2007.12672.
Mojab, N., Noroozi, V., Yu, P., and Hallak, J. (2019). Deep
multi-task learning for interpretable glaucoma detec-
tion. In 2019 IEEE 20th International Conference on
Information Reuse and Integration for Data Science
(IRI), pages 167–174. IEEE.
Schmidt-Erfurth, U., Sadeghipour, A., Gerendas, B. S.,
Waldstein, S. M., and Bogunovi
´
c, H. (2018). Artifi-
cial intelligence in retina. Progress in retinal and eye
research, 67:1–29.
Sivaswamy, J., Krishnadas, S., Joshi, G. D., Jain, M., and
Tabish, A. U. S. (2014). Drishti-gs: Retinal image
dataset for optic nerve head (onh) segmentation. In
2014 IEEE 11th international symposium on biomed-
ical imaging (ISBI), pages 53–56. IEEE.
Thompson, A. C., Jammal, A. A., and Medeiros, F. A.
(2019). A deep learning algorithm to quantify neu-
roretinal rim loss from optic disc photographs. Amer-
ican journal of ophthalmology, 201:9–18.
Ting, D. S. W., Pasquale, L. R., Peng, L., Campbell, J. P.,
Lee, A. Y., Raman, R., Tan, G. S. W., Schmetterer, L.,
Keane, P. A., and Wong, T. Y. (2019). Artificial intel-
ligence and deep learning in ophthalmology. British
Journal of Ophthalmology, 103(2):167–175.
Varadarajan, A. V., Poplin, R., Blumer, K., Angermueller,
C., Ledsam, J., Chopra, R., Keane, P. A., Corrado,
G. S., Peng, L., and Webster, D. R. (2018). Deep
learning for predicting refractive error from retinal
fundus images. Investigative ophthalmology & visual
science, 59(7):2861–2868.
HEALTHINF 2021 - 14th International Conference on Health Informatics
574