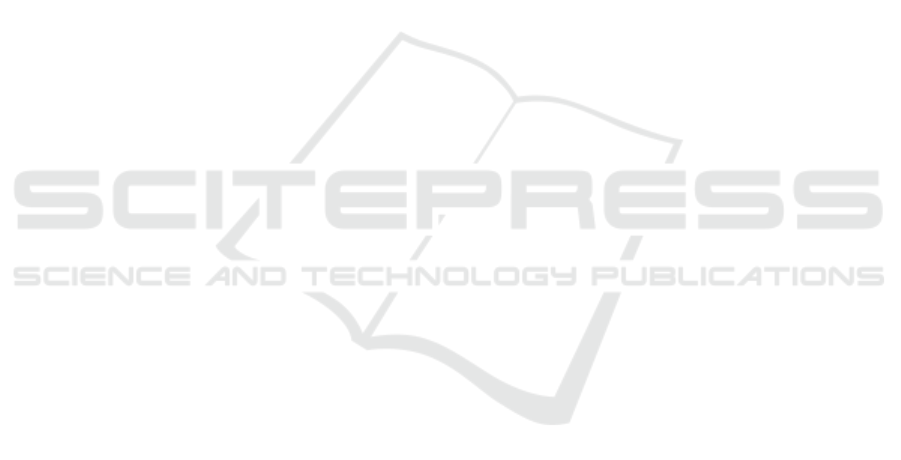
flow. In 2019 IEEE 15th International Scientific Con-
ference on Informatics, pages 469–476.
Chovanec, M., Bachrat
´
y, H., Jasen
ˇ
c
´
akov
´
a, K., and
Bachrat
´
a, K. (2019). Convolutional neural networks
for red blood cell trajectory prediction in simulation
of blood flow. In International Work-Conference on
Bioinformatics and Biomedical Engineering, pages
284–296. Springer.
Cimr
´
ak, I., Gusenbauer, M., and Jan
ˇ
cigov
´
a, I. (2014).
An espresso implementation of elastic objects im-
mersed in a fluid. Computer Physics Communications,
185(3):900–907.
Cimr
´
ak, I., Gusenbauer, M., and Schrefl, T. (2012). Mod-
elling and simulation of processes in microfluidic
devices for biomedical applications. Computers &
Mathematics with Applications, 64(3):278–288.
Cimr
´
ak, I. and Jan
ˇ
cigov
´
a, I. (2018). Computational Blood
Cell Mechanics: Road Towards Models and Biomedi-
cal Applications. CRC Press.
Clevert, D.-A., Unterthiner, T., and Hochreiter, S. (2015).
Fast and accurate deep network learning by exponen-
tial linear units (elus). Under Review of ICLR2016
(1997).
Exl, L., Mauser, N., Schrefl, T., and Suess, D. (2019).
Learning time-stepping by nonlinear dimensionality
reduction to predict magnetization dynamics.
Glorot, X. and Bengio, Y. (2010). Understanding the dif-
ficulty of training deep feedforward neural networks.
Journal of Machine Learning Research - Proceedings
Track, 9:249–256.
Guo, Q., Duffy, S., Matthews, K., Islamzada, E., and Ma,
H. (2017). Deformability based cell sorting using mi-
crofluidic ratchets enabling phenotypic separation of
leukocytes directly from whole blood. Scientific Re-
ports, 7.
Gusenbauer, M., Oezelt, H., Fischbacher, J., Kovacs, A.,
Zhao, P., Woodcock, T., and Schrefl, T. (2020). Ex-
tracting local nucleation fields in permanent magnets
using machine learning. npj Computational Materi-
als, 6:1–10.
Huang, G., Liu, Z., Van Der Maaten, L., and Weinberger,
K. Q. (2017). Densely connected convolutional net-
works. In 2017 IEEE Conference on Computer Vision
and Pattern Recognition (CVPR), pages 2261–2269.
Kingma, D. P. and Ba, J. (2015). Adam: A method for
stochastic optimization. In Bengio, Y. and LeCun,
Y., editors, 3rd International Conference on Learn-
ing Representations, ICLR 2015, San Diego, CA, USA,
May 7-9, 2015, Conference Track Proceedings.
Koval
ˇ
c
´
ıkov
´
a, K., Cimr
´
ak, I., Bachrat
´
a, K., and Bachrat
´
y, H.
(2019). Comparison of Numerical and Laboratory Ex-
periment Examining Deformation of Red Blood Cell,
pages 75–86. Springer International Publishing.
Li, M., Zhang, T., Chen, Y., and Smola, A. J. (2014). Ef-
ficient mini-batch training for stochastic optimization.
In KDD, pages 661–670.
T
´
othov
´
a, R., Jan
ˇ
cigov
´
a, I., and Bu
ˇ
s
´
ık, M. (2015). Calibra-
tion of elastic coefficients for spring-network model
of red blood cell. In 2015 International Conference
on Information and Digital Technologies, pages 376–
380. IEEE.
Tsai, C.-H., Tanaka, J., Kaneko, M., Horade, M., Ito, H.,
Taniguchi, T., Ohtani, T., and Sakata, Y. (2016). An
on-chip rbc deformability checker significantly im-
proves velocity-deformation correlation. Microma-
chines, 7.
Vaswani, A., Shazeer, N., Parmar, N., Uszkoreit, J., Jones,
L., Gomez, A. N., Kaiser, L. u., and Polosukhin, I.
(2017). Attention is all you need. In Guyon, I.,
Luxburg, U. V., Bengio, S., Wallach, H., Fergus, R.,
Vishwanathan, S., and Garnett, R., editors, Advances
in Neural Information Processing Systems 30, pages
5998–6008. Curran Associates, Inc.
Possibilities of using Neural Networks to Blood Flow Modelling
147