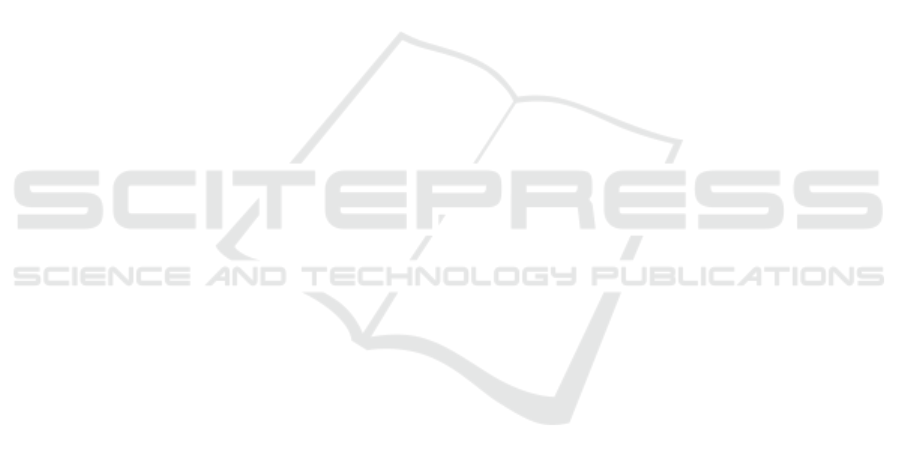
game, trying to mimic pro-players cortical activity.
If both the PGTE and the NFT obtain positive re-
sults, this tools could be used by professional and am-
ateurs eSports teams as a valuable development re-
source.
Next steps of this project will be related to estab-
lishing a link between complementary physiological
signals and performance, using like eye tracking or
electro-dermal activity (EDA).
6 FUNDING
This project has been funded (2020 and 2021) by
the Regional Government, Generalitat Valenciana,
through the Conselleria de Innovaci
´
on, Universi-
dades, Ciencia y Sociedad Digital department, under
the Emergent Research Groups (ERG) program.
7 AUTHOR CONTRIBUTIONS
All authors listed have made a substantial, direct and
intellectual contribution to the work, and approved it
for publication.
REFERENCES
Abernethy, B. (1990). Expertise, visual search, and infor-
mation pick-up in squash. Perception, 19(1):63–77.
Araujo, D., Davids, K., and Hristovski, R. (2006). The eco-
logical dynamics of decision making in sport. Psy-
chology of sport and exercise, 7(6):653–676.
Baker, S. N. (2007). Oscillatory interactions between sen-
sorimotor cortex and the periphery. Current opinion
in neurobiology, 17(6):649–655.
Beauchamp, M. K., Harvey, R. H., and Beauchamp, P. H.
(2012). An integrated biofeedback and psychologi-
cal skills training program for canada’s olympic short-
track speedskating team. Journal of clinical sport psy-
chology, 6(1):67–84.
Behncke, L. (2004). Mental skills training for sports: A
brief review. Online J Sport Psychol, 6(1).
Bryce, J. and Rutter, J. (2002). Killing like a girl: Gendered
gaming and girl gamers’ visibility. In CGDC Conf.
BusinessTelegraph (2020). Esports viewership vs tradi-
tional sports: Revenue and statistics for investors.
Chamberlain, C. J. and Coelho, A. J. (1993). The perceptual
side of action: Decision-making in sport. In Advances
in psychology, volume 102, pages 135–157. Elsevier.
Chase, W. G. and Simon, H. A. (1973). The mind’s eye in
chess. In Visual information processing, pages 215–
281. Elsevier.
Cheng, M.-Y., Wang, K.-P., Hung, C.-L., Tu, Y.-L., Huang,
C.-J., Koester, D., Schack, T., and Hung, T.-M.
(2017). Higher power of sensorimotor rhythm is as-
sociated with better performance in skilled air-pistol
shooters. Psychology of Sport and Exercise, 32:47–
53.
Craig, C. and Watson, G. (2011). An affordance based ap-
proach to decision making in sport: discussing a novel
methodological framework. Revista de psicolog
´
ıa del
deporte, 20(2):689–708.
De Groot, A. D. (2014). Thought and choice in chess, vol-
ume 4. Walter de Gruyter GmbH & Co KG.
Del Percio, C., Babiloni, C., Bertollo, M., Marzano, N.,
Iacoboni, M., Infarinato, F., Lizio, R., Stocchi, M.,
Robazza, C., Cibelli, G., et al. (2009). Visuo-
attentional and sensorimotor alpha rhythms are related
to visuo-motor performance in athletes. Human brain
mapping, 30(11):3527–3540.
Emotive (2019). Performance metrics.
Fajardo, A. and Guzm
´
an, A. L. (2016). Neurofeedback,
aplicaciones y eficacia. Interdisciplinaria, 33(1):81–
93.
Gruzelier, J., Inoue, A., Smart, R., Steed, A., and Steffert, T.
(2010). Acting performance and flow state enhanced
with sensory-motor rhythm neurofeedback comparing
ecologically valid immersive vr and training screen
scenarios. Neuroscience letters, 480(2):112–116.
Guttman, A. (1992). From ritual to record. Sport and reli-
gion, pages 143–152.
Hammond, D. C. (2011). What is neurofeedback: An up-
date. Journal of Neurotherapy, 15(4):305–336.
Hatfield, B. D., Haufler, A. J., Hung, T.-M., and Spald-
ing, T. W. (2004). Electroencephalographic studies of
skilled psychomotor performance. Journal of Clinical
Neurophysiology, 21(3):144–156.
Hilvoorde, I. v. and Pot, N. (2016). Embodiment and fun-
damental motor skills in esports. Sport, Ethics and
Philosophy, 10(1):14–27.
Hung, T.-M., Haufler, A. J., Lo, L.-C., Mayer-Kress, G.,
and Hatfield, B. D. (2008). Visuomotor expertise and
dimensional complexity of cerebral cortical activity.
Medicine & Science in Sports & Exercise, 40(4):752–
759.
Kerick, S. E., McDowell, K., Hung, T.-M., Santa Maria,
D. L., Spalding, T. W., and Hatfield, B. D. (2001).
The role of the left temporal region under the cogni-
tive motor demands of shooting in skilled marksmen.
Biological psychology, 58(3):263–277.
Kontos, A. P. (2004). Perceived risk, risk taking, estimation
of ability and injury among adolescent sport partic-
ipants. Journal of pediatric psychology, 29(6):447–
455.
Pain, M. T. and Pain, M. A. (2005). Essay: risk taking in
sport. The lancet, 366:S33–S34.
Park, J. L., Fairweather, M. M., and Donaldson, D. I.
(2015). Making the case for mobile cognition: Eeg
and sports performance. Neuroscience & Biobehav-
ioral Reviews, 52:117–130.
GRAPP 2021 - 16th International Conference on Computer Graphics Theory and Applications
282