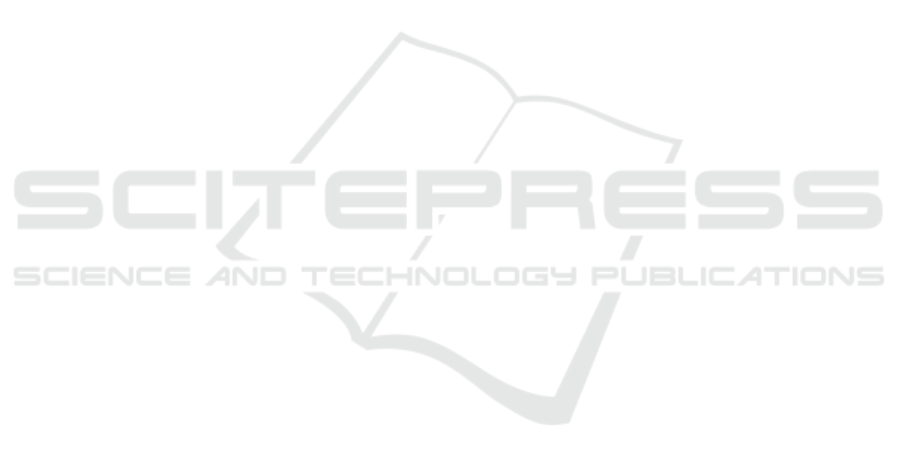
indeed be applied to embeddings. In our test setup
we have used some 3000 article abstracts from the
IEEE conferences and embedded them in five differ-
ent ways using paragraph-sized text embedding tech-
nologies. Performing all-to-all similarity calculations
and verifying the results with regards to a small, man-
ually labeled, ground-truth set indicates that an en-
semble approach yields better quality than any of the
single embeddings taken by themselves (Witschard
et al., 2020). However, much work remains to be done
to fully understand the general rules behind this pro-
cess. Nevertheless, these promising first results, to-
gether with the generalizability of the approach to any
embedding type, encourage us to use this as a foun-
dation for attempting a general framework for MVN
embedding.
REFERENCES
Cui, P., Wang, X., Pei, J., and Zhu, W. (2019). A survey on
network embedding. IEEE Transactions on Knowl-
edge and Data Engineering, 31(5):833–852.
Dong, X., Yu, Z., Cao, W., Shi, Y., and Ma, Q. (2020). A
survey on ensemble learning. Frontiers of Computer
Science, 14(2):241–258.
Goyal, P. and Ferrara, E. (2017). Graph embedding tech-
niques, applications, and performance: A survey.
arXiv:1705.02801.
Hamilton, W. L., Ying, R., and Leskovec, J. (2018). Rep-
resentation learning on graphs: Methods and applica-
tions. arXiv:1709.05584.
Kerren, A., Purchase, H. C., and Ward, M. O. (2014). Multi-
variate Network Visualization. Springer International
Publisher.
Lerique, S., Abitbol, J. L., and Karsai, M. (2020). Joint
embedding of structure and features via graph convo-
lutional networks. Applied Network Science.
Martins, R. M., Kruiger, J. F., Minghim, R., Telea, A. C.,
and Kerren, A. (2017). MVN-Reduce: Dimensional-
ity Reduction for the Visual Analysis of Multivariate
Networks. In Kozlikova, B., Schreck, T., and Wis-
chgoll, T., editors, EuroVis 2017 - Short Papers. The
Eurographics Association.
Ngatchou, P., Zarei, A., and El-Sharkawi, A. (2005). Pareto
multi objective optimization. In Proceedings of the
13th International Conference on, Intelligent Systems
Application to Power Systems, pages 84–91. IEEE.
Nobre, C., Meyer, M., Streit, M., and Lex, A. (2019). The
state of the art in visualizing multivariate networks.
EUROVIS, Volume 38, Number 3.
Opitz, D. and Maclin, R. (1999). Popular ensemble meth-
ods: An empirical study. Journal of Artificial Intelli-
gence Research.
Sun, X., Guo, J., Ding, X., and Liu, T. (2016). A general
framework for content-enhanced network representa-
tion learning. arXiv:1610.02906.
Toshevska, M., Stojanovska, F., and Kalajdjieski, J. (2020).
Comparative analysis of word embeddings for captur-
ing word similarities. arXiv:2005.03812.
Witschard, D., Jusufi, I., Martins, R. M., and Kerren, A.
(2020). Multiple embeddings for multivariate net-
work analysis. Poster abstract presented at 6th an-
nual Big Data Conference at Linnaeus University, in
V
¨
axj
¨
o, Sweden.
A Statement Report on the Use of Multiple Embeddings for Visual Analytics of Multivariate Networks
223