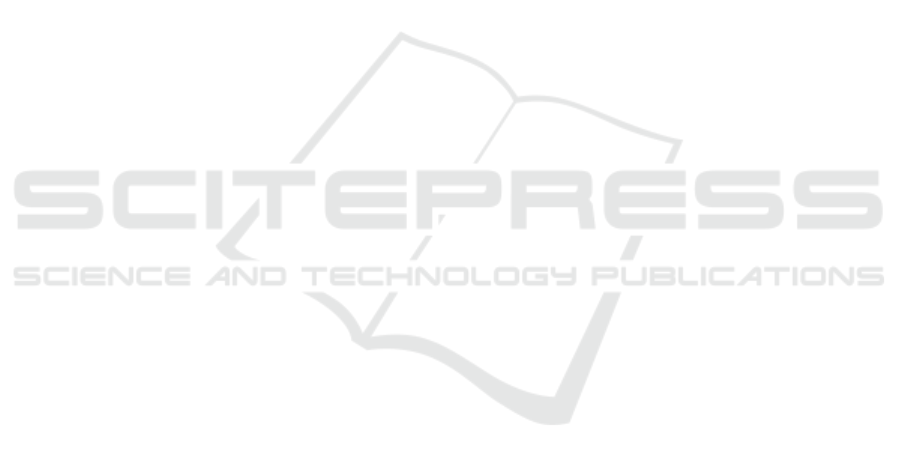
stitution of Mechanical Engineers, Part F: Journal of
Rail and Rapid Transit, 231(10):1174–1185.
G
´
ezero, L. and Antunes, C. (2019). Automated three-
dimensional linear elements extraction from mobile
lidar point clouds in railway environments. Infrastruc-
tures, 4(3):46.
Guan, H., Li, J., Cao, S., and Yu, Y. (2016). Use of mobile
lidar in road information inventory: A review. Interna-
tional Journal of Image and Data Fusion, 7(3):219–
242.
Hu, X. and Ye, L. (2013). A fast and simple method of
building detection from lidar data based on scan line
analysis. ISPRS Annals of the Photogrammetry, Re-
mote Sensing and Spatial Information Sciences, pages
7–13.
Navarro-Serment, L. E., Mertz, C., and Hebert, M.
(2010). Pedestrian detection and tracking using three-
dimensional ladar data. The International Journal of
Robotics Research, 29(12):1516–1528.
Oehler, B., Stueckler, J., Welle, J., Schulz, D., and Behnke,
S. (2011). Efficient multi-resolution plane segmenta-
tion of 3d point clouds. In International Conference
on Intelligent Robotics and Applications, pages 145–
156. Springer.
Qi, C. R., Yi, L., Su, H., and Guibas, L. J. (2017). Point-
net++: Deep hierarchical feature learning on point sets
in a metric space. In Advances in neural information
processing systems, pages 5099–5108.
Redmon, J. and Farhadi, A. (2018). Yolov3: An incremental
improvement. arXiv preprint arXiv:1804.02767.
Ronneberger, O., Fischer, P., and Brox, T. (2015). U-net:
Convolutional networks for biomedical image seg-
mentation. In International Conference on Medical
image computing and computer-assisted intervention,
pages 234–241. Springer.
Sanne, J. M. (2008). Framing risks in a safety-critical and
hazardous job: risk-taking as responsibility in railway
maintenance. Journal of Risk Research, 11:645 – 658.
Shang, L., Yang, Q., Wang, J., Li, S., and Lei, W. (2018).
Detection of rail surface defects based on cnn im-
age recognition and classification. 2018 20th Interna-
tional Conference on Advanced Communication Tech-
nology (ICACT), pages 45–51.
Stein, D., Spindler, M., Kuper, J., and Lauer, M. (2016).
Rail detection using lidar sensors. International
Journal of Sustainable Development and Planning,
11(1):65–78.
Taheriandani, M. (2016). The Application of Doppler LI-
DAR Technology for Rail Inspection and Track Geom-
etry Assessment. PhD thesis, Virginia Tech.
Wang, L., Meng, W., Xi, R., Zhang, Y., Ma, C., Lu, L., and
Zhang, X. (2019). 3d point cloud analysis and clas-
sification in large-scale scene based on deep learning.
IEEE Access, 7:55649–55658.
Wang, W., Sakurada, K., and Kawaguchi, N. (2016). In-
cremental and enhanced scanline-based segmentation
method for surface reconstruction of sparse lidar data.
Remote Sensing, 8(11):967.
Wirth, H. (2008). Der neue lichtraummesszug limez iii der
deutschen bahn ag. Zeitschrift f
¨
ur Geod
¨
asie, Geoin-
formation und Landmanagement, 133(3):180–186.
Wolf, J., Richter, R., Discher, S., and D
¨
ollner, J. (2019a).
Applicability of neural networks for image classifica-
tion on object detection in mobile mapping 3d point
clouds. International Archives of the Photogramme-
try, Remote Sensing & Spatial Information Sciences,
42(4/W15):111–115.
Wolf, J., Richter, R., and D
¨
ollner, J. (2019b). Techniques
for automated classification and segregation of mobile
mapping 3d point clouds. In Proceedings of the 14th
International Joint Conference on Computer Vision,
Imaging and Computer Graphics Theory and Appli-
cations, pages 201–208.
Yan, L., Liu, H., Tan, J., Li, Z., Xie, H., and Chen, C.
(2016). Scan line based road marking extraction from
mobile lidar point clouds. Sensors, 16(6):903.
Yanan, S., Hui, Z., Li, L., and Hang, Z. (2018). Rail surface
defect detection method based on yolov3 deep learn-
ing networks. In 2018 Chinese Automation Congress
(CAC), pages 1563–1568. IEEE.
Yang, Y., Zhang, W., He, Z., and Li, D. (2020). High-
speed rail pole number recognition through deep rep-
resentation and temporal redundancy. Neurocomput-
ing, 415:201–214.
Zhang, Z., Hua, B.-S., and Yeung, S.-K. (2019). Shellnet:
Efficient point cloud convolutional neural networks
using concentric shells statistics. 2019 IEEE/CVF In-
ternational Conference on Computer Vision (ICCV),
pages 1607–1616.
Zhang, Z., Liu, Q., and Wang, Y. (2018). Road extraction
by deep residual u-net. IEEE Geoscience and Remote
Sensing Letters, 15(5):749–753.
VISAPP 2021 - 16th International Conference on Computer Vision Theory and Applications
470