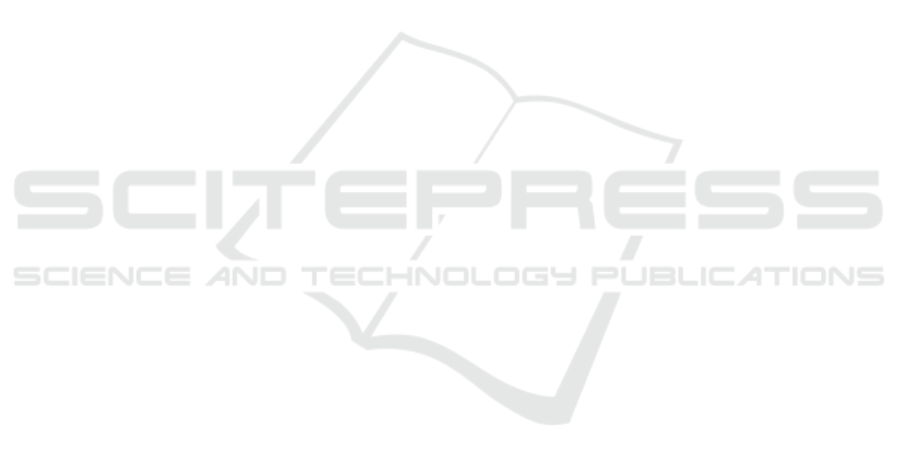
younger populations. IEEE Transactions on Neu-
ral Systems and Rehabilitation Engineering, 27(4).
https://doi.org/10.1109/TNSRE.2019.2890968.
Irimia, D. C., Ortner, R., Poboroniuc, M. S., Ignat,
B. E., and Guger, C. (2018). High classifica-
tion accuracy of a motor imagery based brain-
computer interface for stroke rehabilitation train-
ing. Frontier in Robotics and AI, 5(130):9.
https://doi.org/10.3389/frobt.2018.00130.
Khan, M. A., Das, R., Iversen, H. K., and Puthussery-
pady, S. (2020). Review on motor imagery
based bci systems for upper limb post-stroke
neurorehabilitation: From designing to applica-
tion. Computers in Biology and Medicine, 123.
https://doi.org/10.1016/j.compbiomed.2020.103843.
Le
´
on, J., Escobar, J. J., Ortiz, A., Ortega, J., Gonz
´
alez, J.,
Mart
´
ın-Smith, P., Gan, J. Q., and Damas, M. (2020).
Deep learning for eeg-based motor imagery classifi-
cation: Accuracy-cost trade-off. Plos One, 15(6).
https://doi.org/10.1371/journal.pone.0234178.
Lotte, F., Bougrain, L., Cichocki, A., Clerc, M.,
Congedo, M., Rakotomamonjy, A., and Yger, F.
(2018). A review of classification algorithms for
eeg-based brain–computer interfaces: a 10 year up-
date. Journal of Neural Engineering, 15(3):28.
https://doi.org/10.1088/1741-2552/aab2f2.
M
¨
uller-Gerking, J., Pfurtscheller, G., and Flyvbjerg,
H. (1999). Designing optimal spatial filters
for single-trial eeg classification in a movement
task. Clincial Neurophysiology, 5(1):787–798.
https://doi.org/10.1016/S1388-2457(98)00038-8.
Mubin, O., Alnajjar, F., Mahmud, A. A., Jishtu, N., and
Alsinglawi, B. (2020). Exploring serious games for
stroke rehabilitation: a scoping review. Disability
and Rehabilitation: Assistive Technology, pages 1–7.
https://doi.org/10.1080/17483107.2020.1768309.
Oldfield, R. C. (1971). The assessment and analysis of
handedness: The edinburgh inventory. Neuropsy-
chologia, 9(1):97–113. https://doi.org/10.1016/0028-
3932(71)90067-4.
Ortner, R., Scharinger, J., Lechner, A., and Guger, C.
(2015). How many people can control a motor im-
agery based bci using common spatial patterns? In
2015 7th International IEEE/EMBS Conference on
Neural Engineering (NER), pages 202–205, Montpel-
lier. IEEE. https://doi.org/10.1109/ner.2015.7146595.
Padfield, N., Zabalza, J., Zhao, H., Masero, V., and Ren,
J. (2019). Eeg-based brain-computer interfaces using
motor-imagery: Techniques and challenges. Sensors,
19:1–34. https://doi.org/10.3390/s19061423.
Pfurtscheller, G. and Lopes, F. H. (1999). Event-related
eeg/meg synchronization and desynchronization: ba-
sic principles. Clinical Neurophysiology, 110:1842–
1857. https://doi.org/10.1016/S1388-2457(99)00141-
8.
Pfurtscheller, G., Neuper, C., Obermaier, B., Krausz,
G., Scherer, R., Graimann, B., , and Schrank,
C. (2003). Graz-bci: state of the art and clin-
ical applications. IEEE Transactions on Neural
Systems and Rehabilitation Engineering, 11(2):1–4.
https://doi.org/10.1109/tnsre.2003.814454.
Putze, F. (2019). Brain Art: Brain-Computer Inter-
faces for Artistic Expression, chapter Methods and
Tools for Using BCI with Augmented and Vir-
tual Reality, pages 433–446. Springer, Cham.
https://doi.org/10.1007/978-3-030-14323-7.
Ramos-Murguialday, A., Curado, M. R., Broetz, D., Yil-
maz, ., Brasil, F. L., Liberati, and G., . . . Birbaumer,
N. (2019). Brain-machine interface in chronic
stroke: Randomized trial long-term follow-up. Amer-
ican Society of Neurorehabilitation, 33(3):188–198.
https://doi.org/10.1177/1545968319827573.
Renard, Y., Lotte, F., Gilbert, G., Congedo, M., Maby,
E., Delannoy, V., Bertrand, O., and L
´
ecuyer, A.
(2010). Openvibe: An open-source software plat-
form to design, test and use brain-computer inter-
faces in real and virtual environments. Presence
Teleoperators and Virtual Environments, 19(1):35–53.
https://doi.org/10.1162/pres.19.1.35.
Ros, T., Baars, B. J., Lanius, R. A., and P.Vuilleumier
(2014). Tuning pathological brain oscillations
with neurofeedback: a systems neuroscience frame-
work. Frontiers in Human Neuroscience, 8:1008.
https://doi.org/10.3389/fnhum.2014.01008.
Suryotrisongko, H. and Samopa, F. (2015). Eval-
uating openbci spiderclaw v1 headwear’s
electrodes placements for brain-computer
interface (bci) motor imagery application.
Procedia Computer Science, 72:398–405.
https://doi.org/10.1016/j.procs.2015.12.155.
Vourvopoulos, A. (2018). Using Brain-Computer Interac-
tion and Multimodal Virtual-Reality for Augmenting
Stroke Neurorehabilitation. PhD thesis, Universidade
da Madeira. https://doi.org/10400.13/1992.
Vourvopoulos, A., Ferreira, A., and Bermudez, S.
(2016). Neurow: An immersive vr environment
for motor-imagery training with the use of brain-
computer interfaces and vibrotactile feedback. In
3rd International Conference on Physiological Com-
puting Systems, Lisbon, Portugal. SCITEPRESS.
https://doi.org/10.5220/0005939400430053.
Vourvopoulos, A., Jorge, C., Abreu, R., Figueiredo, P.,
Fernandes, J., and Bermudez, S. (2019). Effi-
cacy and brain imaging correlates of an immer-
sive motor imagery bci-driven vr system for upper
limb motor rehabilitation: A clinical case report.
Frontiers in Human Neuroscience, 13(244):567–577.
https://doi.org/10.3389/fnhum.2019.00244.
Wang, J., Feng, Z., Ren, X., Lu, N., Luo, J., and Sun,
L. (2020). Feature subset and time segment selec-
tion for the classification of eeg data based motor im-
agery. Biomedical Signal Processing and Control, 61.
https://doi.org/10.1016/j.bspc.2020.102026.
Finding the Optimal Time Window for Increased Classification Accuracy during Motor Imagery
151