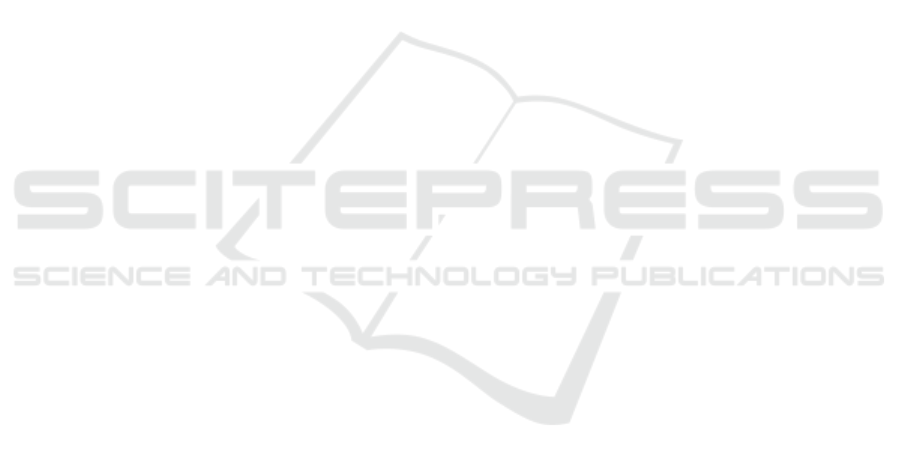
infrastructure for biological and medical imaging" of
the Ministry of Education, Youth and Sports of the
CR (No. CZ.02.1.01/0.0/0.0/16_013/0001775).
REFERENCES
Alaskar, H. (2019). Deep Learning-based Model Architec-
ture for Time-Frequency Images Analysis. January
2018.
Andén, J., & Mallat, S. (2014). Deep scattering spectrum.
IEEE Transactions on Signal Processing, 62(16),
4114–4128.
Bruna, J., & Mallat, S. (2013). Invariant scattering
convolution networks. IEEE Transactions on Pattern
Analysis and Machine Intelligence, 35(8), 1872–1886.
Chen, D., Wang, Z., Guo, D., Orekhov, V., & Qu, X.
(2020). Review and Prospect: Deep Learning in
Nuclear Magnetic Resonance Spectroscopy. Chemistry
- A European Journal, 26(46), 10391–10401.
Graveron-Demilly, D. (2014). Quantification in magnetic
resonance spectroscopy based on semi-parametric
approaches. In Magnetic Resonance Materials in
Physics, Biology and Medicine (Vol. 27, Issue 2, pp.
113–130). Springer Verlag.
Hatami, N., Lyon, B., & Etienne, U. (2018). Magnetic
Resonance Spectroscopy Quantification using Deep
Learning.
ISMRM. (2016). MRS Fitting Challenge (ismrm.org
/workshops/Spectroscopy16/mrs_fitting_challenge/).
Kreis, R., & Kyathanahally, S. P. (2018). Deep Learning
Approaches for Detection and Removal of Ghosting
Artifacts in MR Spectroscopy. 863, 851–863.
Lee, H. H., & Kim, H. (2019). Intact metabolite spectrum
mining by deep learning in proton magnetic resonance
spectroscopy of the brain. Magnetic Resonance in
Medicine, 82(1), 33–48.
Opstad, K. S., Bell, B. A., Griffiths, J. R., & Howe, F. A.
(2008). Toward accurate quantification of metabolites,
lipids, and macromolecules in HRMAS spectra of
human brain tumor biopsies using LCModel. Magnetic
Resonance in Medicine, 60(5).
Osorio-Garcia, M. I., Sima, D. M., Nielsen, F. U.,
Dresselaers, T., Van Leuven, F., Himmelreich, U., &
Van Huffel, S. (2011). Quantification of in vivo 1H
magnetic resonance spectroscopy signals with baseline
and lineshape estimation. Measurement Science and
Technology, 22(11), 1–17.
Poullet, J. B., Sima, D. M., Simonetti, A. W., De Neuter,
B., Vanhamme, L., Lemmerling, P., & Van Huffel, S.
(2007). An automated quantification of short echo time
MRS spectra in an open source software environment:
AQSES. NMR in Biomedicine, 20(5), 493–504.
Poullet, J. B., Sima, D. M., & Van Huffel, S. (2008). MRS
signal quantitation: A review of time- and frequency-
domain methods. Journal of Magnetic Resonance,
195(2), 134–144.
Robin A. de Graaf. (2019). In Vivo NMR Spectroscopy. In
In Vivo NMR Spectroscopy (pp. 1–42).
Stagg, C., & Rothman, D. (2014). Magnetic Resonance
Spectroscopy: Tools for Neuroscience Research.
Starčuk, Z., & Starčuková, J. (2017). Quantum-mechanical
simulations for in vivo MR spectroscopy: Principles
and possibilities demonstrated with the program
NMRScopeB. Analytical Biochemistry, 529.
Stefan, D., Cesare, F. Di, Andrasescu, A., Popa, E.,
Lazariev, A., Vescovo, E., Strbak, O., Williams, S.,
Starcuk, Z., Cabanas, M., Van Ormondt, D., &
Graveron-Demilly, D. (2009). Quantitation of magnetic
resonance spectroscopy signals: The jMRUI software
package. Measurement Science and Technology, 20(10).
Suvichakorn, A., Ratiney, H., Bucur, A., Cavassila, S., &
Antoine, J. P. (2008). Quantification method using the
Morlet wavelet for Magnetic Resonance Spectroscopic
signals with macromolecular contamination.
Proceedings of the 30th Annual International Conf. of
the IEEE Engineering in Medicine and Biology Society,
EMBS’08 - “Personalized Healthcare through
Technology,” 2681–2684.
Van Der Graaf, M. (2010). In vivo magnetic resonance
spectroscopy: Basic methodology and clinical
applications. European Biophysics Journal, 39(4),
527–540.
A Wavelet Scattering Convolutional Network for Magnetic Resonance Spectroscopy Signal Quantitation
275