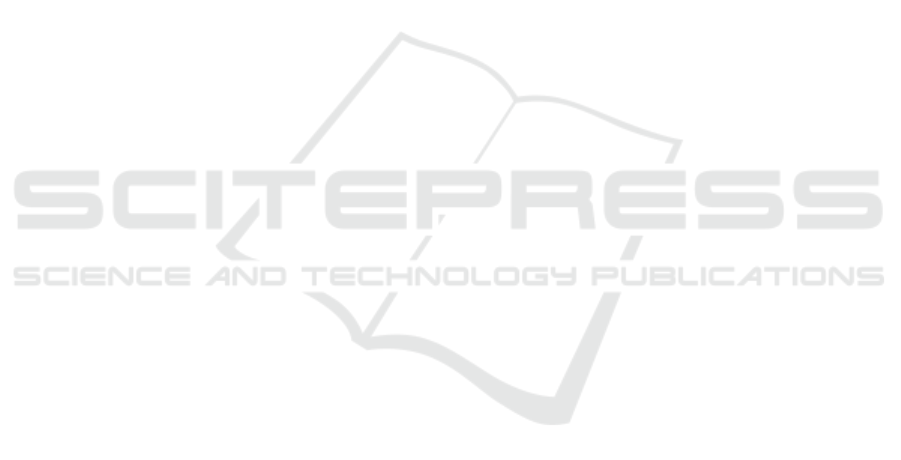
REFERENCES
Badrinarayanan, V., Kendall, A., and Cipolla, R. (2017).
Segnet: A deep convolutional encoder-decoder ar-
chitecture for image segmentation. IEEE Transac-
tions on Pattern Analysis and Machine Intelligence,
39(12):2481–2495.
Bay, H., Tuytelaars, T., and Van Gool, L. (2006). Surf:
Speeded up robust features. In Leonardis, A., Bischof,
H., and Pinz, A., editors, Computer Vision – ECCV
2006, pages 404–417, Berlin, Heidelberg. Springer
Berlin Heidelberg.
Chaudhary, V., Dave, I., and Upla, K. (2017). Automatic vi-
sual inspection of printed circuit board for defect de-
tection and classification. pages 732–737.
Chen, L., Papandreou, G., Schroff, F., and Adam, H.
(2017). Rethinking atrous convolution for semantic
image segmentation. CoRR, abs/1706.05587.
Chen, L.-C., Papandreou, G., Kokkinos, I., Murphy, K., and
Yuille, A. L. (2014). Semantic image segmentation
with deep convolutional nets and fully connected crfs.
arXiv preprint arXiv:1412.7062.
Cheng, Y. (1995). Mean shift, mode seeking, and clus-
tering. IEEE Trans. Pattern Anal. Mach. Intell.,
17(8):790–799.
d. S. Silva, L. H., d. A. Azevedo, G. O., Fernandes, B. J. T.,
Bezerra, B. L. D., Lima, E. B., and Oliveira, S. C.
(2019). Automatic optical inspection for defective pcb
detection using transfer learning. In 2019 IEEE Latin
American Conference on Computational Intelligence
(LA-CCI), pages 1–6.
Erhan, D., Manzagol, P.-A., Bengio, Y., Bengio, S., and
Vincent, P. (2009). The difficulty of training deep ar-
chitectures and the effect of unsupervised pre-training.
Journal of Machine Learning Research - Proceedings
Track, 5:153–160.
Ester, M., Kriegel, H.-P., Sander, J., and Xu, X. (1996).
A density-based algorithm for discovering clusters in
large spatial databases with noise. pages 226–231.
AAAI Press.
Howard, A. G., Zhu, M., Chen, B., Kalenichenko, D.,
Wang, W., Weyand, T., Andreetto, M., and Adam,
H. (2017). Mobilenets: Efficient convolutional neu-
ral networks for mobile vision applications. CoRR,
abs/1704.04861.
Huang, W. and Wei, P. (2019). A pcb dataset for defects
detection and classification.
Ibrahim, Z. and Al-Attas, S. (2005). Wavelet-based
printed circuit board inspection algorithm. Integrated
Computer-Aided Engineering, 12:201–213.
Iglovikov, V. and Shvets, A. (2018). Ternausnet: U-net with
vgg11 encoder pre-trained on imagenet for image seg-
mentation.
Indera Putera, S. H. and Ibrahim, Z. (2010). Printed cir-
cuit board defect detection using mathematical mor-
phology and matlab image processing tools. In 2010
2nd International Conference on Education Technol-
ogy and Computer, volume 5, pages V5–359–V5–
363.
Khalilian, S., Hallaj, Y., Balouchestani, A., Karshenas, H.,
and Mohammadi, A. (2020). Pcb defect detection us-
ing denoising convolutional autoencoders. In 2020 In-
ternational Conference on Machine Vision and Image
Processing (MVIP), pages 1–5.
Long, J., Shelhamer, E., and Darrell, T. (2014). Fully
convolutional networks for semantic segmentation.
CoRR, abs/1411.4038.
Ma, N., Zhang, X., Zheng, H., and Sun, J. (2018). Shuf-
flenet V2: practical guidelines for efficient CNN ar-
chitecture design. CoRR, abs/1807.11164.
Otsu, N. (1979). A threshold selection method from gray-
level histograms. IEEE Transactions on Systems,
Man, and Cybernetics, 9(1):62–66.
Perez, L. and Wang, J. (2017). The effectiveness of data
augmentation in image classification using deep learn-
ing. CoRR, abs/1712.04621.
Ren, S., He, K., Girshick, R. B., and Sun, J. (2015). Faster
R-CNN: towards real-time object detection with re-
gion proposal networks. CoRR, abs/1506.01497.
Ronneberger, O., Fischer, P., and Brox, T. (2015). U-net:
Convolutional networks for biomedical image seg-
mentation. CoRR, abs/1505.04597.
Sandler, M., Howard, A. G., Zhu, M., Zhmoginov, A., and
Chen, L. (2018). Inverted residuals and linear bottle-
necks: Mobile networks for classification, detection
and segmentation. CoRR, abs/1801.04381.
Santoyo, J., Pedraza Ortega, J. C., Mej
´
ıa, L., and Santoyo,
A. (2007). Pcb inspection using image processing and
wavelet transform. volume 4827, pages 634–639.
Srimani, P. and Prathiba, V. (2016). Adaptive data mining
approach for pcb defect detection and classification.
Indian journal of science and technology, 9.
Tang, S., He, F., Huang, X., and Yang, J. (2019). Online
pcb defect detector on a new pcb defect dataset.
Vafeiadis, T., Dimitriou, N., Ioannidis, D., Wotherspoon, T.,
Tinker, G., and Tzovaras, D. (2018). A framework for
inspection of dies attachment on pcb utilizing machine
learning techniques. Journal of Management Analyt-
ics, 5:1–14.
Wei, P., Liu, C., Liu, M., Gao, Y., and Liu, H. (2018).
Cnn-based reference comparison method for classi-
fying bare pcb defects. The Journal of Engineering,
2018(16):1528–1533.
Yosinski, J., Clune, J., Bengio, Y., and Lipson, H. (2014).
How transferable are features in deep neural net-
works? CoRR, abs/1411.1792.
Zhang, L., Jin, Y., Yang, X., Li, X., Duan, X., Sun, Y., and
Liu, H. (2018). Convolutional neural network-based
multi-label classification of pcb defects. The Journal
of Engineering, 2018(16):1612–1616.
Zhang, X., Zhou, X., Lin, M., and Sun, J. (2017). Shuf-
flenet: An extremely efficient convolutional neural
network for mobile devices. CoRR, abs/1707.01083.
Zhao, H., Shi, J., Qi, X., Wang, X., and Jia, J. (2016). Pyra-
mid scene parsing network. CoRR, abs/1612.01105.
U-Net based Zero-hour Defect Inspection of Electronic Components and Semiconductors
601