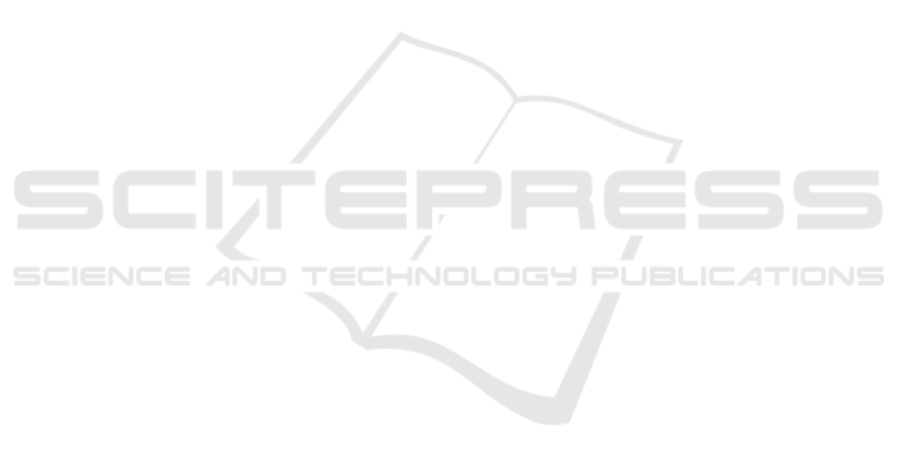
5 CONCLUSIONS
In this paper we were able to collect important aspects
for the approach to the topic of pHRI in the care do-
main. There is a general need for physical relief in
care. For this relief through robotic assistance, how-
ever, a force application directly or indirectly on hu-
mans is necessary. Safety standards with values for
force limits depending on the body part do already ex-
ist but these were not created with the intention of pro-
viding relief in care and are currently only means to an
end. It requires a systematic creation of care-related
force limits. When testing care-relevant robotic sup-
port movements, initial experiments using humans is
not desirable and one should switch to patient sim-
ulators for this particular task. In the present paper,
however, it could be shown that there is a mismatch
between patient simulators and humans, which must
either be taken into account or developments in this
field must take place so that simulators become more
similar to humans with a suitable weight, material and
mobility. Finally, we were able to show that even col-
laborative lightweight robots can apply enough force
to independently move an 80 kg patient simulator in
bed and are thus also suitable for nursing activities.
6 FUTURE WORK
The presented work should serve as a basis for the
field of pHRI for nursing care and should also show
that despite existing gaps in the framework condi-
tions, there is a potential for force relief of caregivers
by collaborative robots. In future work, we will fo-
cus on directly supporting caregivers using robots and
on measuring and comparing the degree of potential
physical relief. For this, the three main difficulties in
this complex project mentioned at the beginning have
to be considered more intensively in follow-up work.
On the one hand, an additional assessment of physi-
cal properties may possibly provide an advantage in
the transmission of force. On the other hand, nursing
activities at the bed are such highly complex activi-
ties that this problem should perhaps be handled by a
robot controller learned through reinforcement learn-
ing rather than using a handcrafted controller. Over-
all, there are still many areas where the present system
can be further improved and used for research. We
envision a system which ensures significant physical
relief through human-robot interaction and coopera-
tion while maintaining safety standards with regard
to maximum force limits dependent on the patient’s
condition.
ACKNOWLEDGEMENTS
This work was funded by the German Ministry
for Education and Research (BMBF) within the re-
search project Nursing Care Innovation Center (grant
16SV7819K).
REFERENCES
Akhtar, R., Sherratt, M. J., Cruickshank, J. K., and Derby,
B. (2011). Characterizing the elastic properties of tis-
sues. Materials Today, 14(3):96–105.
Byravan, A. and Fox, D. (2017). Se3-nets: Learning rigid
body motion using deep neural networks. In 2017
IEEE International Conference on Robotics and Au-
tomation (ICRA), pages 173–180. IEEE.
De Santis, A., Siciliano, B., De Luca, A., and Bicchi, A.
(2008). An atlas of physical human–robot interaction.
Mechanism and Machine Theory, 43(3):253–270.
Dogar, M. and Srinivasa, S. (2011). A framework for push-
grasping in clutter. Robotics: Science and systems VII,
1.
Ehrentraut, O., Hackmann, T., Krämer, L., and Schmutz,
S. (2015). Zukunft der Pflegepolitik: Perspek-
tiven, Handlungsoptionen und Politikempfehlungen.
Friedrich-Ebert-Stiftung, Abteilung Wirtschafts-und
Sozialpolitik.
Ehrhardt, S., Monszpart, A., Mitra, N. J., and Vedaldi, A.
(2017). Learning a physical long-term predictor. arXiv
preprint arXiv:1703.00247.
Erickson, Z., Clever, H. M., Gangaram, V., Turk, G.,
Liu, C. K., and Kemp, C. C. (2019a). Multidimen-
sional capacitive sensing for robot-assisted dressing
and bathing. In 2019 IEEE 16th International Confer-
ence on Rehabilitation Robotics (ICORR), pages 224–
231. IEEE.
Erickson, Z., Gangaram, V., Kapusta, A., Liu, C. K., and
Kemp, C. C. (2019b). Assistive gym: A physics simu-
lation framework for assistive robotics. arXiv preprint
arXiv:1910.04700.
Fifelski, C., Brinkmann, A., Ortmann, S. M., Isken, M., and
Hein, A. (2018). Multi depth camera system for 3d
data recording for training and education of nurses. In
2018 International Conference on Computational Sci-
ence and Computational Intelligence (CSCI), pages
679–684. IEEE.
Golovin, V., Arkhipov, M., and Zhuravlev, V. (2014). Force
training for position/force control of massage robots.
In New trends in medical and service robots, pages
95–107. Springer.
Haddadin, S., Albu-Schaffer, A., De Luca, A., and
Hirzinger, G. (2008). Collision detection and reac-
tion: A contribution to safe physical human-robot in-
teraction. In 2008 IEEE/RSJ International Conference
on Intelligent Robots and Systems, pages 3356–3363.
IEEE.
Handling Comparison between a Human and a Patient Simulator for Nursing Care Related Physical Human-robot Interaction
611