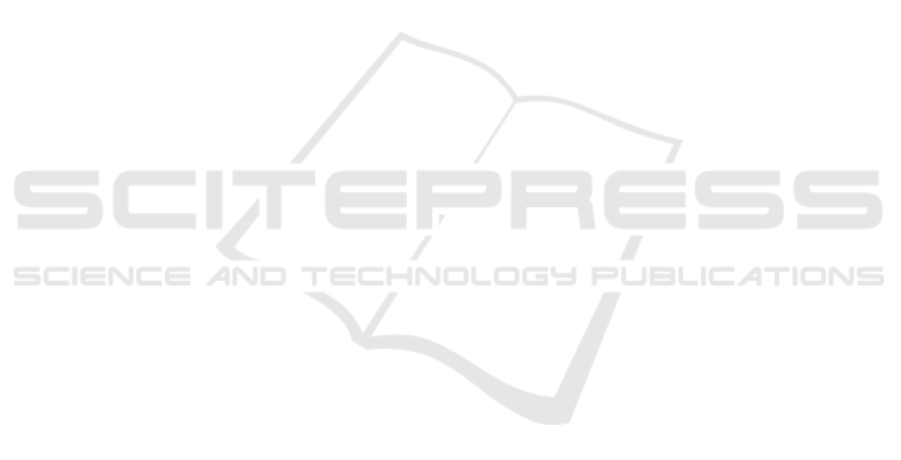
Budai, A., Bock, R., Maier, A., Hornegger, J., and Michel-
son, G. (2013). Robust vessel segmentation in fundus
images. International journal of biomedical imaging,
2013:154860.
Ding, A., Chen, Q., Cao, Y., and Liu, B. (2020). Retinopa-
thy of prematurity stage diagnosis using object seg-
mentation and convolutional neural networks. arXiv
preprint arXiv:2004.01582.
Dogra, M. R., Katoch, D., and Dogra, M. (2017). An up-
date on retinopathy of prematurity (rop). The Indian
Journal of Pediatrics, 84(12):930–936.
Fraz, M. M., Remagnino, P., Hoppe, A., Uyyanonvara, B.,
Rudnicka, A. R., Owen, C. G., and Barman, S. A.
(2012). Blood vessel segmentation methodologies in
retinal images–a survey. Computer methods and pro-
grams in biomedicine, 108(1):407–433.
Guo, X., Kikuchi, Y., Wang, G., Yi, J., Zou, Q., and Zhou,
R. (2020). Early detection of retinopathy of prema-
turity (rop) in retinal fundus images via convolutional
neural networks. arXiv preprint arXiv:2006.06968.
Hoover, A. D., Kouznetsova, V., and Goldbaum, M. (2000).
Locating blood vessels in retinal images by piecewise
threshold probing of a matched filter response. IEEE
Transactions on Medical Imaging, 19(3):203–210.
Islam, M., Poly, T. N., Walther, B. A., Yang, H. C., Li,
Y.-C. J., et al. (2020). Artificial intelligence in oph-
thalmology: A meta-analysis of deep learning models
for retinal vessels segmentation. Journal of clinical
medicine, 9(4):1018.
Islam, M. M., Poly, T. N., and Li, Y.-C. J. (2019). Retinal
vessels detection using convolutional neural networks
in fundus images. bioRxiv, page 737668.
Jocher, G., Stoken, A., Borovec, J., NanoCode012, Christo-
pherSTAN, Changyu, L., Laughing, Hogan, A., loren-
zomammana, tkianai, yxNONG, AlexWang1900, Di-
aconu, L., Marc, wanghaoyang0106, ml5ah, Doug,
Hatovix, Poznanski, J., L. Y., changyu98, Rai, P., Fer-
riday, R., Sullivan, T., Xinyu, W., YuriRibeiro, Clara-
munt, E. R., hopesala, pritul dave, and yzchen (2020).
ultralytics/yolov5: v3.0.
Luo, Y., Chen, K., Mao, J., Shen, L., and Sun, M. (2020).
A fusion deep convolutional neural network based on
pathological features for diagnosing plus disease in
retinopathy of prematurity. Investigative Ophthalmol-
ogy & Visual Science, 61(7):2017–2017.
Organization, W. H. et al. (2019). World report on vision.
Technical report, Geneva: World Health Organization.
Ravichandran, C. and Raja, J. B. (2014). A fast enhance-
ment/thresholding based blood vessel segmentation
for retinal image using contrast limited adaptive his-
togram equalization. Journal of Medical Imaging and
Health Informatics, 4(4):567–575.
Redd, T. K., Campbell, J. P., Brown, J. M., Kim, S. J.,
Ostmo, S., Chan, R. V. P., Dy, J., Erdogmus, D., Ioan-
nidis, S., Kalpathy-Cramer, J., et al. (2019). Evalua-
tion of a deep learning image assessment system for
detecting severe retinopathy of prematurity. British
Journal of Ophthalmology, 103(5):580–584.
Staal, J., Abramoff, M., Niemeijer, M., Viergever, M., and
van Ginneken, B. (2004). Ridge based vessel segmen-
tation in color images of the retina. IEEE Transactions
on Medical Imaging, 23(4):501–509.
Tan, Z., Simkin, S., Lai, C., and Dai, S. (2019). Deep learn-
ing algorithm for automated diagnosis of retinopathy
of prematurity plus disease. Translational Vision Sci-
ence & Technology, 8(6):23–23.
Ting, D. S., Peng, L., Varadarajan, A. V., Keane, P. A.,
Burlina, P., Chiang, M. F., Schmetterer, L., Pasquale,
L. R., Bressler, N. M., Webster, D. R., et al. (2019a).
Deep learning in ophthalmology: the technical and
clinical considerations. Progress in retinal and eye
research.
Ting, D. S. W., Pasquale, L. R., Peng, L., Campbell, J. P.,
Lee, A. Y., Raman, R., Tan, G. S. W., Schmetterer, L.,
Keane, P. A., and Wong, T. Y. (2019b). Artificial in-
telligence and deep learning in ophthalmology. British
Journal of Ophthalmology, 103(2):167–175.
Wang, J., Ju, R., Chen, Y., Zhang, L., Hu, J., Wu, Y., Dong,
W., Zhong, J., and Yi, Z. (2018). Automated retinopa-
thy of prematurity screening using deep neural net-
works. EBioMedicine, 35:361–368.
Wang, X., Jiang, X., and Ren, J. (2019). Blood vessel seg-
mentation from fundus image by a cascade classifica-
tion framework. Pattern Recognition, 88:331 – 341.
Yavuz, Z. and K
¨
ose, C. (2017). Blood vessel extraction
in color retinal fundus images with enhancement fil-
tering and unsupervised classification. Journal of
Healthcare Engineering, 2017:1–12.
Yildiz, V. M., Tian, P., Yildiz, I., Brown, J. M., Kalpathy-
Cramer, J., Dy, J., Ioannidis, S., Erdogmus, D.,
Ostmo, S., Kim, S. J., et al. (2020). Plus disease in
retinopathy of prematurity: Convolutional neural net-
work performance using a combined neural network
and feature extraction approach. Translational Vision
Science & Technology, 9(2):10–10.
Zhang, Y., Wang, L., Wu, Z., Zeng, J., Chen, Y., Tian, R.,
Zhao, J., and Zhang, G. (2018). Development of an
automated screening system for retinopathy of prema-
turity using a deep neural network for wide-angle reti-
nal images. IEEE Access, 7:10232–10241.
Deep Learning Assisted Retinopathy of Prematurity Screening Technique
243