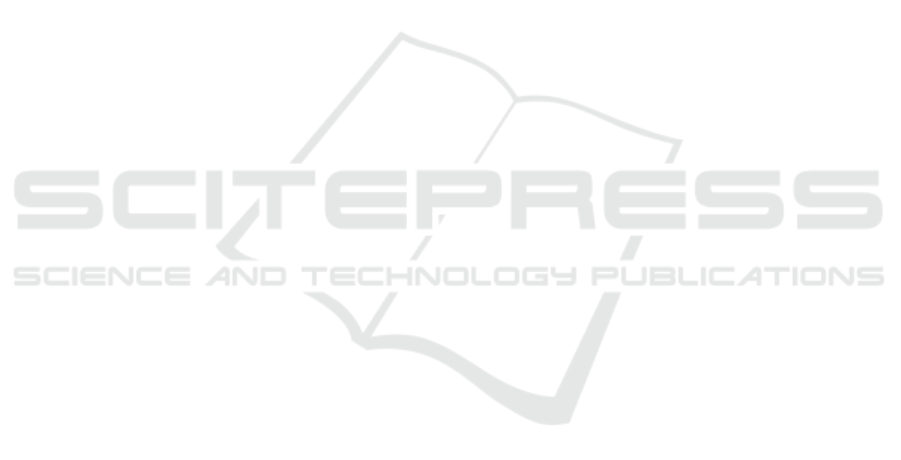
Giancola, S., Valenti, M., and Sala, R. (2018). A Survey
on 3D Cameras: Metrological Comparison of Time-
of-Flight, Structured-Light and Active Stereoscopy
Technologies. SpringerBriefs in Computer Science.
Springer International Publishing, Cham, 1 edition.
Hartley, R. and Zisserman, A. (2004). Multiple View Geom-
etry in Computer Vision. Cambridge University Press,
Cambridge, UK, 2 edition. https://doi.org/10.1017.
Hirschm
¨
uller, H. (2008). Stereo Processing by Semiglobal
Matching and Mutual Information. IEEE Transac-
tions on Pattern Analysis and Machine Intelligence,
30(2):328–341.
Kannala, J. and Brandt, S. S. (2006). A generic camera
model and calibration method for conventional, wide-
angle, and fish-eye lenses. IEEE Transactions on Pat-
tern Analysis and Machine Intelligence, 28(8):1335–
1340. Conference Name: IEEE Transactions on Pat-
tern Analysis and Machine Intelligence.
Kendall, A., Martirosyan, H., Dasgupta, S., Henry, P.,
Kennedy, R., Bachrach, A., and Bry, A. (2017). End-
to-End Learning of Geometry and Context for Deep
Stereo Regression. In 2017 IEEE International Con-
ference on Computer Vision (ICCV), pages 66–75,
Venice. IEEE.
Kingma, D. P. and Ba, J. (2015). Adam: A Method for
Stochastic Optimization. In Bengio, Y. and LeCun,
Y., editors, 3rd International Conference on Learn-
ing Representations, ICLR 2015, San Diego, CA, USA,
May 7-9, 2015, Conference Track Proceedings.
Li, S. (2006). Trinocular Spherical Stereo. In 2006
IEEE/RSJ International Conference on Intelligent
Robots and Systems, pages 4786–4791, Beijing,
China.
Liu, S., De Mello, S., Gu, J., Zhong, G., Yang, M.-H., and
Kautz, J. (2017). Learning Affinity via Spatial Propa-
gation Networks. In Guyon, I., Luxburg, U. V., Ben-
gio, S., Wallach, H., Fergus, R., Vishwanathan, S., and
Garnett, R., editors, Advances in Neural Information
Processing Systems 30, pages 1520–1530. Curran As-
sociates, Inc.
Loshchilov, I. and Hutter, F. (2017). SGDR: Stochastic Gra-
dient Descent with Warm Restarts. In 5th Interna-
tional Conference on Learning Representations, ICLR
2017, Toulon, France, April 24-26, 2017, Conference
Track Proceedings. OpenReview.net.
Luo, W., Schwing, A. G., and Urtasun, R. (2016). Efficient
Deep Learning for Stereo Matching. In 2016 IEEE
Conference on Computer Vision and Pattern Recogni-
tion (CVPR), pages 5695–5703, Las Vegas, NV, USA.
IEEE.
Mayer, N., Ilg, E., Hausser, P., Fischer, P., Cremers, D.,
Dosovitskiy, A., and Brox, T. (2016). A Large Dataset
to Train Convolutional Networks for Disparity, Opti-
cal Flow, and Scene Flow Estimation. In 2016 IEEE
Conference on Computer Vision and Pattern Recogni-
tion (CVPR), pages 4040–4048, Las Vegas, NV, USA.
IEEE.
Menze, M. and Geiger, A. (2015). Object scene flow for
autonomous vehicles. In 2015 IEEE Conference on
Computer Vision and Pattern Recognition (CVPR),
pages 3061–3070, Boston, MA, USA. IEEE.
Ronneberger, O., Fischer, P., and Brox, T. (2015). U-Net:
Convolutional Networks for Biomedical Image Seg-
mentation. pages 234–241. Springer, Cham.
Scharstein, D., Hirschm
¨
uller, H., Kitajima, Y., Krath-
wohl, G., Ne
ˇ
si
´
c, N., Wang, X., and Westling,
P. (2014). High-Resolution Stereo Datasets with
Subpixel-Accurate Ground Truth. volume 8753, pages
31–42, Cham. Springer. Series Title: Lecture Notes in
Computer Science.
Scharstein, D., Szeliski, R., and Zabih, R. (2001). A
taxonomy and evaluation of dense two-frame stereo
correspondence algorithms. In Proceedings IEEE
Workshop on Stereo and Multi-Baseline Vision (SMBV
2001), pages 131–140, Kauai, HI, USA. IEEE Com-
put. Soc.
Scheck, T., Seidel, R., and Hirtz, G. (2020). Learning from
theodore: A synthetic omnidirectional top-view in-
door dataset for deep transfer learning. In Proceedings
of the IEEE/CVF Winter Conference on Applications
of Computer Vision (WACV).
ˇ
Zbontar, J. and LeCun, Y. (2016). Stereo matching by train-
ing a convolutional neural network to compare image
patches. J. Mach. Learn. Res., 17(1):2287–2318.
Wang, Y., Lai, Z., Huang, G., Wang, B. H., van der Maaten,
L., Campbell, M., and Weinberger, K. Q. (2019). Any-
time Stereo Image Depth Estimation on Mobile De-
vices. In 2019 International Conference on Robotics
and Automation (ICRA), pages 5893–5900, Montreal,
QC, Canada. IEEE.
Won, C., Ryu, J., and Lim, J. (2019a). OmniMVS: End-to-
End Learning for Omnidirectional Stereo Matching.
In 2019 IEEE/CVF International Conference on Com-
puter Vision (ICCV), pages 8986–8995, Seoul, Korea
(South). IEEE.
Won, C., Ryu, J., and Lim, J. (2019b). SweepNet: Wide-
baseline Omnidirectional Depth Estimation. In 2019
International Conference on Robotics and Automation
(ICRA), pages 6073–6079, Montreal, QC, Canada.
IEEE.
Won, C., Ryu, J., and Lim, J. (2020). End-to-End Learn-
ing for Omnidirectional Stereo Matching with Uncer-
tainty Prior. IEEE Transactions on Pattern Analysis
and Machine Intelligence. Early Access.
VISAPP 2021 - 16th International Conference on Computer Vision Theory and Applications
816