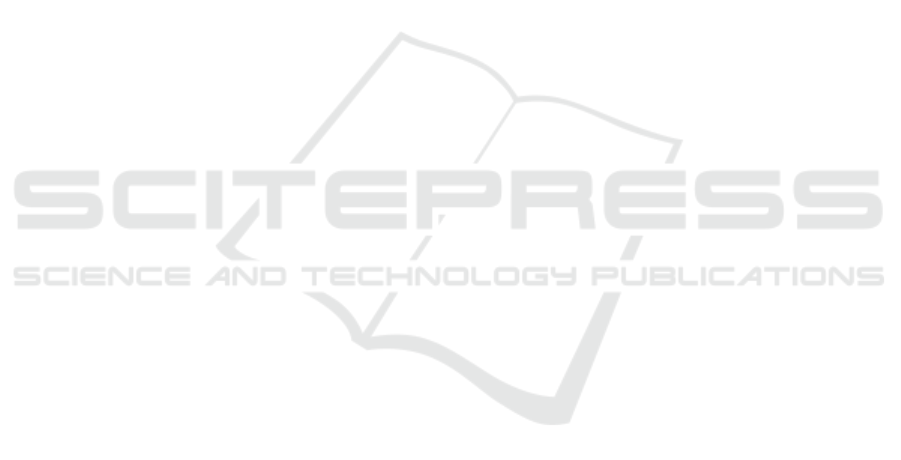
REFERENCES
Chen, Y., Shi, F., Christodoulou, A. G., Xie, Y., Zhou, Z.,
and Li, D. (2018). Efficient and accurate mri super-
resolution using a generative adversarial network and
3d multi-level densely connected network. In In-
ternational Conference on Medical Image Comput-
ing and Computer-Assisted Intervention, pages 91–
99. Springer.
Damkat, C. (2011). Single image super-resolution using
self-examples and texture synthesis. Signal, Image
and Video Processing, 5(3):343–352.
Faber, V. and Dougherty, R. L. (2007). Method for loss-
less encoding of image data by approximating linear
transforms and preserving selected properties for im-
age processing. US Patent 7,218,789.
Hatzigiorgaki, M. and Skodras, A. N. (2003). Compressed
domain image retrieval: a comparative study of simi-
larity metrics. In Visual Communications and Image
Processing 2003, volume 5150, pages 439–448. Inter-
national Society for Optics and Photonics.
Jacquin, A. E. et al. (1992). Image coding based on a fractal
theory of iterated contractive image transformations.
IEEE Transactions on image processing, 1(1):18–30.
Jiang, K., Wang, Z., Yi, P., Wang, G., Lu, T., and Jiang, J.
(2019). Edge-enhanced gan for remote sensing image
superresolution. IEEE Transactions on Geoscience
and Remote Sensing, 57(8):5799–5812.
Khatami, A., Khosravi, A., Nguyen, T., Lim, C. P., and
Nahavandi, S. (2017). Medical image analysis using
wavelet transform and deep belief networks. Expert
Systems with Applications, 86:190–198.
Ledig, C., Theis, L., Husz
´
ar, F., Caballero, J., Cunningham,
A., Acosta, A., Aitken, A., Tejani, A., Totz, J., Wang,
Z., et al. (2017). Photo-realistic single image super-
resolution using a generative adversarial network. In
Proceedings of the IEEE conference on computer vi-
sion and pattern recognition, pages 4681–4690.
Lee, D., Sim, A., Choi, J., and Wu, K. (2016). Novel data
reduction based on statistical similarity. In Proceed-
ings of the 28th International Conference on Scientific
and Statistical Database Management, pages 1–12.
Lee, D., Sim, A., Choi, J., and Wu, K. (2019). Idealem: Sta-
tistical similarity based data reduction. arXiv preprint
arXiv:1911.06980.
Liu, B. and Ling, Q. (2020). Edge-guided depth im-
age super-resolution based on ksvd. IEEE Access,
8:41108–41115.
Liu, H., Fu, Z., Han, J., Shao, L., Hou, S., and Chu, Y.
(2019). Single image super-resolution using multi-
scale deep encoder–decoder with phase congruency
edge map guidance. Information Sciences, 473:44–
58.
Massey Jr, F. J. (1951). The kolmogorov-smirnov test for
goodness of fit. Journal of the American statistical
Association, 46(253):68–78.
Mittal, A., Moorthy, A. K., and Bovik, A. C. (2012).
No-reference image quality assessment in the spatial
domain. IEEE Transactions on image processing,
21(12):4695–4708.
Sajjadi, M. S., Scholkopf, B., and Hirsch, M. (2017). En-
hancenet: Single image super-resolution through au-
tomated texture synthesis. In Proceedings of the IEEE
International Conference on Computer Vision, pages
4491–4500.
Sayood, K. (2012). Introduction to data compression.
Newnes.
Selinger, P. (2003). Potrace: a polygon-based tracing algo-
rithm, http://potrace.sourceforge.net/potrace.pdf.
Stasik, P. M. and Balcerek, J. (2017). Improvements in up-
scaling of pixel art. In 2017 Signal Processing: Al-
gorithms, Architectures, Arrangements, and Applica-
tions (SPA), pages 371–376. IEEE.
Sun, J., Xu, Z., and Shum, H.-Y. (2008). Image super-
resolution using gradient profile prior. In 2008 IEEE
Conference on Computer Vision and Pattern Recogni-
tion, pages 1–8. IEEE.
Venkatanath, N., Praneeth, D., Bh, M. C., Channappayya,
S. S., and Medasani, S. S. (2015). Blind image qual-
ity evaluation using perception based features. In
2015 Twenty First National Conference on Commu-
nications (NCC), pages 1–6. IEEE.
Wang, L., Xiang, S., Meng, G., Wu, H., and Pan, C.
(2013). Edge-directed single-image super-resolution
via adaptive gradient magnitude self-interpolation.
IEEE Transactions on Circuits and Systems for Video
Technology, 23(8):1289–1299.
Wang, Y., Su, F., and Qian, Y. (2019). Text-attentional
conditional generative adversarial network for super-
resolution of text images. In 2019 IEEE International
Conference on Multimedia and Expo (ICME), pages
1024–1029. IEEE.
Wohlberg, B. and De Jager, G. (1999). A review of the
fractal image coding literature. IEEE Transactions on
Image Processing, 8(12):1716–1729.
Xie, J., Feris, R. S., and Sun, M.-T. (2015). Edge-guided
single depth image super resolution. IEEE Transac-
tions on Image Processing, 25(1):428–438.
Yang, W., Feng, J., Yang, J., Zhao, F., Liu, J., Guo, Z., and
Yan, S. (2017). Deep edge guided recurrent residual
learning for image super-resolution. IEEE Transac-
tions on Image Processing, 26(12):5895–5907.
Yang, W., Zhang, X., Tian, Y., Wang, W., Xue, J.-H., and
Liao, Q. (2019). Deep learning for single image super-
resolution: A brief review. IEEE Transactions on Mul-
timedia, 21(12):3106–3121.
Zhang, K., Zuo, W., and Zhang, L. (2018). Learning a sin-
gle convolutional super-resolution network for multi-
ple degradations. In Proceedings of the IEEE Con-
ference on Computer Vision and Pattern Recognition,
pages 3262–3271.
Zhang, K., Zuo, W., and Zhang, L. (2019). Deep plug-
and-play super-resolution for arbitrary blur kernels. In
Proceedings of the IEEE Conference on Computer Vi-
sion and Pattern Recognition, pages 1671–1681.
Zhou, D., Shen, X., and Dong, W. (2012). Image zooming
using directional cubic convolution interpolation. IET
image processing, 6(6):627–634.
Single Image Super-resolution using Vectorization and Texture Synthesis
519