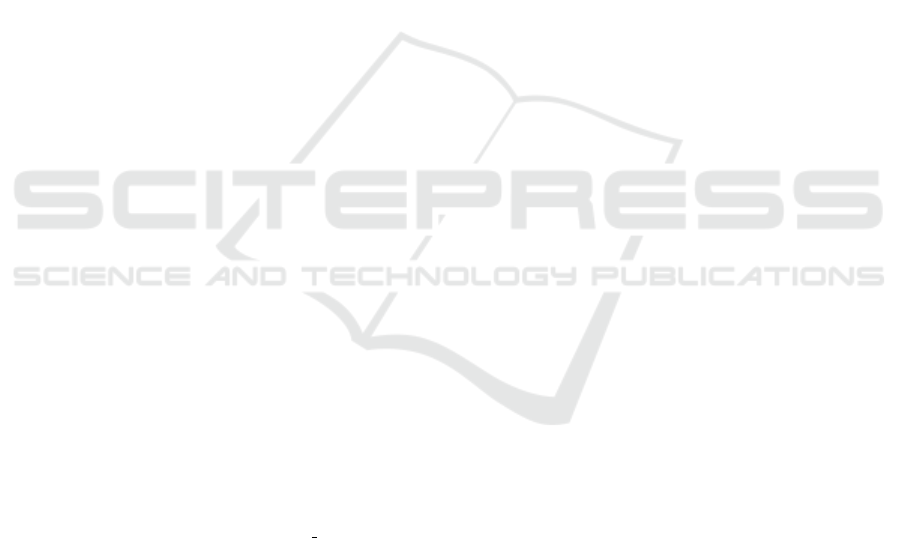
can be compared to robust methods at the level of
precision, given the results obtained in the identifica-
tion of all strides for the datasets used. This method,
also presents an ideal configuration for a possible in-
tegration in a real-time system, which is a prospective
breakthrough in this work.
It was presented a complete algorithm that allows
gait metrics to be calculated using data from iner-
tial sensors in patients with motor difficulties, geri-
atric patients. This system presents adequate results
to make the specific gait evaluation for the right and
left foot. Although this system present less accurate
results than the analogue study (Barth et al., 2015), is
considered adequate for the scope of gait physiother-
apy. In the future, we intend to calculate other gait
metrics such as double support period, stride width,
swing width and gait speed, which allow a more de-
tailed analysis for people with Parkinson’s, and also
integrate it in a real-time system that allows feedback
to patient whenever the algorithm evaluates a risky
gait pattern, based on spatial parameters.
ACKNOWLEDGEMENTS
Supported by project Indoor Activity Notification for
Vigilance Services (AAL-2018-5-116), funded under
the AAL JP and co-funded by the European Commis-
sion and the National Funding Authorities of Portu-
gal, Belgium, and Switzerland.
REFERENCES
Barth, J., Oberndorfer, C., Pasluosta, C., Sch
¨
ulein, S.,
Gassner, H., Reinfelder, S., Kugler, P., Schuld-
haus, D., Winkler, J., Klucken, J., and Eskofier,
B. M. (2015). Stride segmentation during free walk
movements using multi-dimensional subsequence dy-
namic time warping on inertial sensor data. Sensors,
15(3):6419–6440.
Community, T. S. (2020). scipy.signal.find peaks — SciPy
v1.5.3 Reference Guide.
FAU (2015). Digital Biobank - eGaIT (embedded
Gait analysis using Intelligent Technologies).
https://www.mad.tf.fau.de/research/activitynet/digital-
biobank/.
Ferrari, A., Ginis, P., Hardegger, M., Casamassima, F., Roc-
chi, L., and Chiari, L. (2016). A mobile Kalman-filter
based solution for the real-time estimation of spatio-
temporal gait parameters. IEEE Trans. Neural Syst.
Rehabil. Eng., 24(7):764–773.
Fourati, H. (2015). Heterogeneous data fusion algorithm for
pedestrian navigation via foot-mounted inertial mea-
surement unit and complementary filter. IEEE Trans.
Instrum. Meas., 64(1):221–229.
Guo, H., Uradzinski, M., Yin, H., and Yu, M. (2015). Indoor
positioning based on foot-mounted IMU. Bulletin of
the Polish Academy of Sciences: Technical Sciences,
63(3):629–634.
Haji Ghassemi, N., Hannink, J., Martindale, C. F., Gaßner,
H., M
¨
uller, M., Klucken, J., and Eskofier, B. M.
(2018). Segmentation of gait sequences in sensor-
based movement analysis: A comparison of methods
in Parkinson’s disease. Sensors, 18(1):1–15.
Kalilani, L., Asgharnejad, M., Palokangas, T., and Durgin,
T. (2016). Comparing the incidence of falls/fractures
in Parkinson’s disease patients in the US population.
PLoS ONE, 11(9):1–11.
Keus, S. H., Munneke, M., Nijkrake, M. J., Kwakkel, G.,
and Bloem, B. R. (2009). Physical therapy in Parkin-
son’s disease: Evolution and future challenges. Move-
ment Disorders, 24(1):1–14.
Mariani, B., Jim
´
enez, M. C., Vingerhoets, F. J., and
Aminian, K. (2013). On-shoe wearable sensors for
gait and turning assessment of patients with parkin-
son’s disease. IEEE. Trans. Biomed. Eng., 60(1):155–
158.
Moore, D. J., West, A. B., Dawson, V. L., and Dawson,
T. M. (2005). Molecular Pathophysiology of Parkin-
son ’ s Disease. Annual Review of Neuroscience,
26(1):57–87.
Nelson, A. J., Zwick, D., Brody, S., Doran, C., Pulver,
L., Rooz, G., Sadownick, M., Nelson, R., and Roth-
man, J. (2002). The validity of the GaitRite and the
functional ambulation performance scoring system in
the analysis of Parkinson gait. NeuroRehabilitation,
17(3):255–262.
Rampp, A., Barth, J., Sch
¨
ulein, S., Gaßmann, K. G.,
Klucken, J., and Eskofier, B. M. (2015). Inertial
Sensor-Based Stride Parameter Calculation From Gait
Sequences in Geriatric Patients. IEEE. Trans. Biomed.
Eng., 62(4):1089–1097.
Tomlinson, C. L., Patel, S., Meek, C., Herd, C. P., Clarke,
C. E., Stowe, R., Shah, L., Sackley, C. M., Deane,
K. H., Wheatley, K., and Ives, N. (2014). Physio-
therapy for Parkinson’s disease: a comparison of tech-
niques. CDSR, pages 1–108.
Tunca, C., Pehlivan, N., Ak, N., Arnrich, B., Salur, G.,
and Ersoy, C. (2017). Inertial sensor-based robust gait
analysis in non-hospital settings for neurological dis-
orders. Sensors, 17(4):1–29.
Tunca, C., Salur, G., and Ersoy, C. (2020). Deep Learn-
ing for Fall Risk Assessment with Inertial Sensors:
Utilizing Domain Knowledge in Spatio-Temporal
Gait Parameters. IEEE J. Biomed. Health Inform.,
24(7):1994–2005.
Wang, Z., Zhao, H., Qiu, S., and Gao, Q. (2015). Stance-
Phase Detection for ZUPT-Aided Foot-Mounted
Pedestrian Navigation System. IEEE/ASME Trans.
Mechatronics, 20(6):3170–3181.
BIOSIGNALS 2021 - 14th International Conference on Bio-inspired Systems and Signal Processing
334