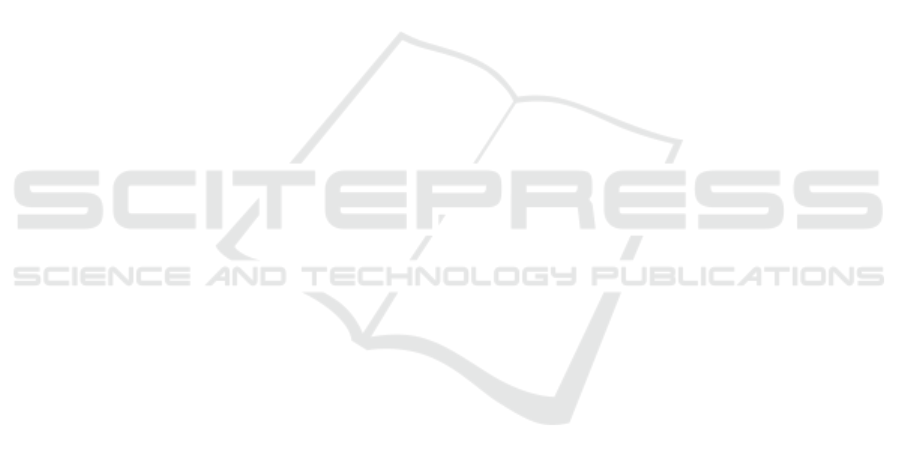
Kays, R., Crofoot, M. C., Jetz, W., and Wikelski, M. (2015).
Terrestrial animal tracking as an eye on life and planet.
Science, 348(6240).
Kemelmacher-Shlizerman, I. (2016). Transfiguring por-
traits. ACM Trans. Graph., 35:94:1–94:8.
Khirodkar, R., Yoo, D., and Kitani, K. M. (2018). Do-
main randomization for scene-specific car detection
and pose estimation. CoRR, abs/1811.05939.
Kingma, D. P. and Ba, J. (2015). Adam: A method for
stochastic optimization. In 3rd International Confer-
ence on Learning Representations, ICLR 2015, San
Diego, CA, USA, May 7-9, 2015.
Lin, T.-Y., Maire, M., Belongie, S., Hays, J., Perona, P.,
Ramanan, D., Doll
´
ar, P., and Zitnick, C. L. (2014).
Microsoft coco: Common objects in context. In Euro-
pean conference on computer vision, pages 740–755.
Springer.
Martinez, J., Hossain, R., Romero, J., and Little, J. J.
(2017). A simple yet effective baseline for 3d human
pose estimation. 2017 IEEE International Conference
on Computer Vision (ICCV), pages 2659–2668.
Mehta, D., Sridhar, S., Sotnychenko, O., Rhodin, H.,
Shafiei, M., Seidel, H.-P., Xu, W., Casas, D., and
Theobalt, C. (2017). Vnect: Real-time 3d human
pose estimation with a single rgb camera. ACM Trans.
Graph., 36(4):44:1–44:14.
Mu, J., Qiu, W., Hager, G., and Yuille, A. (2019). Learning
from synthetic animals.
Newell, A., Yang, K., and Deng, J. (2016). Stacked hour-
glass networks for human pose estimation. In ECCV.
Pavlakos, G., Zhou, X., Derpanis, K. G., and Daniilidis,
K. (2016). Coarse-to-fine volumetric prediction for
single-image 3d human pose.
Pavllo, D., Feichtenhofer, C., Grangier, D., and Auli, M.
(2019). 3d human pose estimation in video with tem-
poral convolutions and semi-supervised training. In
Conference on Computer Vision and Pattern Recogni-
tion (CVPR).
Pereira, T., Aldarondo, D. E., Willmore, L., Kislin, M.,
Wang, S. S.-H., Murthy, M., and Shaevitz, J. W.
(2018). Fast animal pose estimation using deep neural
networks. bioRxiv.
Perlin, K. (2002). Improving noise. ACM Trans. Graph.,
21(3):681–682.
Prakash, A., Boochoon, S., Brophy, M., Acuna, D., Cam-
eracci, E., State, G., Shapira, O., and Birchfield, S.
(2019). Structured domain randomization: Bridging
the reality gap by context-aware synthetic data. In In-
ternational Conference on Robotics and Automation,
ICRA 2019, Montreal, QC, Canada, May 20-24, 2019,
pages 7249–7255.
Rogge, L., Klose, F., Stengel, M., Eisemann, M., and Mag-
nor, M. (2014). Garment replacement in monocular
video sequences. ACM Trans. Graph., 34(1):6:1–6:10.
Simonyan, K. and Zisserman, A. (2015). Very deep con-
volutional networks for large-scale image recognition.
In International Conference on Learning Representa-
tions.
Sutherland, I. E. (1968). A head-mounted three dimensional
display. In AFIPS Fall Joint Computing Conference,
pages 757–764.
Tobin, J., Fong, R., Ray, A., Schneider, J., Zaremba, W., and
Abbeel, P. (2017). Domain randomization for transfer-
ring deep neural networks from simulation to the real
world. CoRR, abs/1703.06907.
Tom
`
e, D., Russell, C., and Agapito, L. (2017). Lifting from
the deep: Convolutional 3d pose estimation from a
single image. 2017 IEEE Conference on Computer
Vision and Pattern Recognition (CVPR), pages 5689–
5698.
Tremblay, J., Prakash, A., Acuna, D., Brophy, M., Jam-
pani, V., Anil, C., To, T., Cameracci, E., Boochoon,
S., and Birchfield, S. (2018). Training deep networks
with synthetic data: Bridging the reality gap by do-
main randomization. CoRR, abs/1804.06516.
Varol, G., Romero, J., Martin, X., Mahmood, N., Black,
M. J., Laptev, I., and Schmid, C. (2017). Learning
from synthetic humans. 2017 IEEE Conference on
Computer Vision and Pattern Recognition (CVPR).
Wei, S.-E., Ramakrishna, V., Kanade, T., and Sheikh, Y.
(2016). Convolutional pose machines. In CVPR.
Xiao, B., Wu, H., and Wei, Y. (2018). Simple baselines
for human pose estimation and tracking. In European
Conference on Computer Vision (ECCV).
Xu, W., Chatterjee, A., Zollh
¨
ofer, M., Rhodin, H., Mehta,
D., Seidel, H.-P., and Theobalt, C. (2018). Monop-
erfcap: Human performance capture from monocular
video. ACM Trans. Graph., 37(2).
Xu, Y., Zhu, S.-C., and Tung, T. (2019). Denserac: Joint
3d pose and shape estimation by dense render-and-
compare. In The IEEE International Conference on
Computer Vision (ICCV).
Yang, S., Ambert, T., Pan, Z., Wang, K., Yu, L., Berg, T. L.,
and Lin, M. C. (2016). Detailed garment recovery
from a single-view image. CoRR, abs/1608.01250.
Zuffi, S., Kanazawa, A., Berger-Wolf, T., and Black, M. J.
(2019). Three-d safari: Learning to estimate zebra
pose, shape, and texture from images ”in the wild”. In
International Conference on Computer Vision.
Zuffi, S., Kanazawa, A., and Black, M. J. (2018). Lions and
tigers and bears: Capturing non-rigid, 3D, articulated
shape from images. In IEEE Conference on Computer
Vision and Pattern Recognition (CVPR). IEEE Com-
puter Society.
Zuffi, S., Kanazawa, A., Jacobs, D., and Black, M. J.
(2016). 3d menagerie: Modeling the 3d shape and
pose of animals.
GRAPP 2021 - 16th International Conference on Computer Graphics Theory and Applications
132