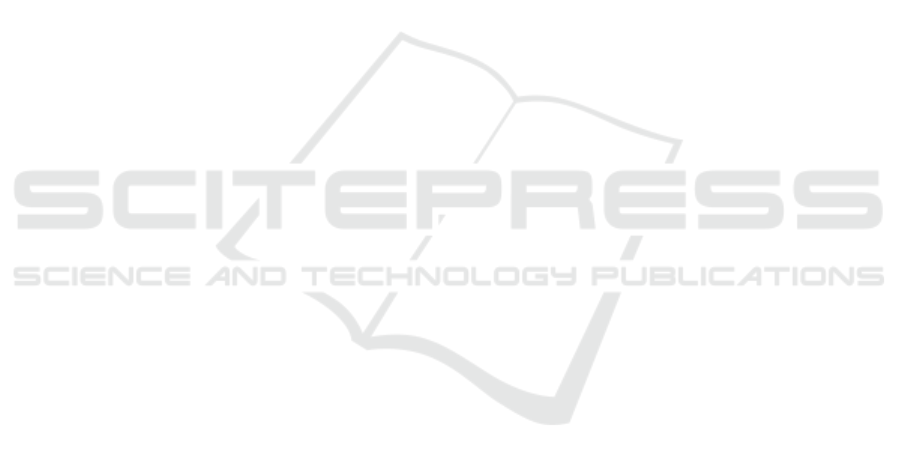
IEEE Conference on Computer Vision and Pattern
Recognition, pages 580–587.
Duan, K., Parikh, D., Crandall, D., and Grauman, K.
(2012). Discovering localized attributes for fine-
grained recognition. In 2012 IEEE Conference on
Computer Vision and Pattern Recognition, pages
3474–3481.
Fong, R. C. and Vedaldi, A. (2017). Interpretable explana-
tions of black boxes by meaningful perturbation. In
2017 IEEE International Conference on Computer Vi-
sion, pages 3429–3437.
Fukui, H., Hirakawa, T., Yamashita, T., and Fujiyoshi, H.
(2019). Attention branch network: Learning of atten-
tion mechanism for visual explanation. In 2019 IEEE
Conference on Computer Vision and Pattern Recogni-
tion, pages 10705–10714.
He, K., Zhang, X., Ren, S., and Sun, J. (2016). Deep resid-
ual learning for image recognition. In 2016 IEEE Con-
ference on Computer Vision and Pattern Recognition,
pages 770–778.
Hu, J., Shen, L., and Sun, G. (2018). Squeeze-and-
excitation networks. In 2018 IEEE Conference
on Computer Vision and Pattern Recognition, pages
7132–7141.
Jeffrey, De, F., Joseph, R., L., Bernardino, R.-P., Stanislav,
N., Nenad, T., Sam, B., Harry, A., Xavier, G., Bren-
dan, O., Daniel, V., George, van, d. D., Balaji, L.,
Clemens, M., Faith, M., Simon, B., Kareem, A.,
Reena, C., Dominic, K., Alan, K., C
´
ıan, O., H., Ros-
alind, R., Julian, H., Dawn, A., S., Catherine, E., Ad-
nan, T., Hugh, M., Demis, H., Geraint, R., Trevor, B.,
Peng, T., K., Mustafa, S., Julien, C., Pearse, A., K.,
and Olaf, R. (2018). Clinically applicable deep learn-
ing for diagnosis and referral in retinal disease. Nature
Medicine, (24):1342–1350.
Jetley, S., Lord, N. A., Lee, N., and Torr, P. (2018). Learn to
pay attention. In International Conference on Learn-
ing Representations.
Kelvin, X., Jimmy, B., Ryan, K., Kyunghyun, C., Aaron, C.,
Ruslan, S., Rich, Z., and Yoshua, B. (2015). Show, at-
tend and tell: Neural image caption generation with
visual attention. In International Conference on Ma-
chine Learning, pages 2048–2057.
LeCun, Y., Boser, B., Denker, J. S., Henderson, D., Howard,
R. E., Hubbard, W., and Jackel, L. D. (1989). Back-
propagation applied to handwritten zip code recogni-
tion. Neural Computation, 1(4):541–551.
Lin, M., Chen, Q., and Yan, S. (2014). Network in network.
In 2nd International Conference on Learning Rep-
resentations, Banff, AB, Canada, April 14-16, 2014,
Conference Track Proceedings.
Linsley, D., Shiebler, D., Eberhardt, S., and Serre, T. (2019).
Learning what and where to attend with humans in the
loop. In International Conference on Learning Repre-
sentations.
Luong, T., Pham, H., and Manning, C. D. (2015). Effective
approaches to attention-based neural machine transla-
tion. In Empirical Methods in Natural Language Pro-
cessing, pages 1412–1421.
Mnih, V., Heess, N., Graves, A., and kavukcuoglu, k.
(2014). Recurrent models of visual attention. In
Neural Information Processing Systems, pages 2204–
2212.
Montavon, G., Samek, W., and M
¨
uller, K.-R. (2018). Meth-
ods for interpreting and understanding deep neural
networks. Digital Signal Processing, 73:1–15.
Parikh, D. and Grauman, K. (2011). Interactively building
a discriminative vocabulary of nameable attributes. In
2011 IEEE Conference on Computer Vision and Pat-
tern Recognition, pages 1681–1688.
Parkash, A. and Parikh, D. (2012). Attributes for classi-
fier feedback. In European Conference on Computer
Vision, pages 354–368.
Petsiuk, V., Das, A., and Saenko, K. (2018). Rise: Ran-
domized input sampling for explanation of black-box
models. In British Machine Vision Conference.
Porwal, P., Pachade, S., Kamble, R., Kokare, M., Desh-
mukh, G., Sahasrabuddhe, V., and Meriaudeau, F.
(2018). Indian diabetic retinopathy image dataset
(idrid): A database for diabetic retinopathy screening
research. Data, 3:25.
Quattoni, A., Wang, S., Morency, L.-P., Collins, M., and
Darrell, T. (2007). Hidden conditional random fields.
IEEE Transactions on Pattern Analysis & Machine In-
telligence, (10):1848–1852.
Ramprasaath, R., S., Michael, C., Abhishek, D., Ramakr-
ishna, V., Devi, P., and Dhruv, B. (2017). Grad-CAM:
Visual explanations from deep networks via gradient-
based localization. In International Conference on
Computer Vision, pages 618–626.
Ribeiro, M. T., Singh, S., and Guestrin, C. (2016). Why
should I trust you?: Explaining the predictions of any
classifier. In 22nd ACM SIGKDD International Con-
ference on Knowledge Discovery and Data Mining,
pages 1135–1144.
Ryan, P., Avinash, V., V., Katy, B., Yun, L., Michael, V., M.,
Greg, S., C., Lily, P., and Dale, R., W. (2018). Pre-
diction of cardiovascular risk factors from retinal fun-
dus photographs via deep learning. Nature Biomedical
Engineering, (2):158–164.
Smilkov, D., Thorat, N., Kim, B., Vi
´
egas, F., and Watten-
berg, M. (2017). Smoothgrad: removing noise by
adding noise. arXiv preprint arXiv:1706.03825.
Springenberg, J. T., Dosovitskiy, A., Brox, T., and Ried-
miller, M. A. (2015). Striving for simplicity: The
all convolutional net. In International Conference on
Learning Representations.
Vaswani, A., Shazeer, N., Parmar, N., Uszkoreit, J., Jones,
L., Gomez, A. N., Kaiser, Ł., and Polosukhin, I.
(2017). Attention is all you need. In Advances in
Neural Information Processing Systems, pages 5998–
6008.
Wang, F., Jiang, M., Qian, C., Yang, S., Li, C., Zhang, H.,
Wang, X., and Tang, X. (2017). Residual attention
network for image classification. In 2017 IEEE Con-
ference on Computer Vision and Pattern Recognition,
pages 3156–3164.
Wang, X., Girshick, R., Gupta, A., and He, K. (2018).
Non-local neural networks. In 2018 IEEE Conference
Embedding Human Knowledge into Deep Neural Network via Attention Map
635