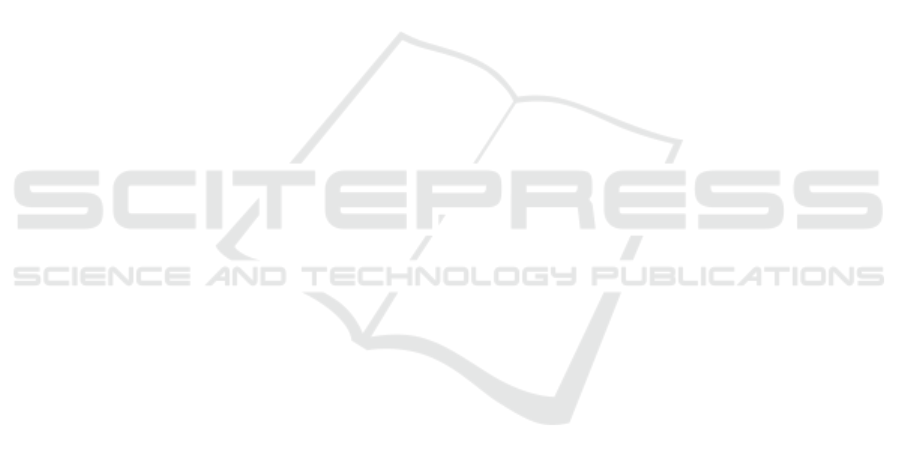
the IEEE Conference on Computer Vision and Pattern
Recognition, pages 6016–6025.
Farneb
¨
ack, G. (2003). Two-frame motion estimation based
on polynomial expansion. In Scandinavian conference
on Image analysis, pages 363–370. Springer.
Gao, Y., Beijbom, O., Zhang, N., and Darrell, T. (2016).
Compact bilinear pooling. In Proceedings of the IEEE
Conference on Computer Vision and Pattern Recogni-
tion, pages 317–326.
Girdhar, R. and Ramanan, D. (2017). Attentional pooling for
action recognition. In Advances in Neural Information
Processing Systems, pages 34–45.
Girdhar, R., Ramanan, D., Gupta, A., Sivic, J., and Russell,
B. (2017). Actionvlad: Learning spatio-temporal ag-
gregation for action classification. In Proceedings of
the IEEE Conference on Computer Vision and Pattern
Recognition, pages 971–980.
He, K., Zhang, X., Ren, S., and Sun, J. (2016). Deep resid-
ual learning for image recognition. In Proceedings of
the IEEE Conference on Computer Vision and Pattern
Recognition, pages 770–778.
Hershey, S., Chaudhuri, S., Ellis, D. P., Gemmeke, J. F.,
Jansen, A., Moore, R. C., Plakal, M., Platt, D., Saurous,
R. A., Seybold, B., et al. (2017). CNN architectures for
large-scale audio classification. In Acoustics, Speech
and Signal Processing (ICASSP), 2017 IEEE Interna-
tional Conference on, pages 131–135. IEEE.
Hu, J.-F., Zheng, W.-S., Pan, J., Lai, J., and Zhang, J. (2018).
Deep bilinear learning for RGB-D action recognition.
In Proceedings of the European Conference on Com-
puter Vision (ECCV), pages 335–351.
Kar, P. and Karnick, H. (2012). Random feature maps for dot
product kernels. In Artificial Intelligence and Statistics,
pages 583–591.
Karpathy, A., Toderici, G., Shetty, S., Leung, T., Sukthankar,
R., and Fei-Fei, L. (2014). Large-scale video classifi-
cation with convolutional neural networks. In Proceed-
ings of the IEEE Conference on Computer Vision and
Pattern Recognition, pages 1725–1732.
Lin, T.-Y., RoyChowdhury, A., and Maji, S. (2015). Bilinear
CNN models for fine-grained visual recognition. In
Proceedings of the IEEE International Conference on
Computer Vision, pages 1449–1457.
Liu, J., Yuan, Z., and Wang, C. (2018). Towards good prac-
tices for multi-modal fusion in large-scale video classi-
fication. In Proceedings of the European Conference
on Computer Vision (ECCV), pages 0–0.
Pham, N. and Pagh, R. (2013). Fast and scalable polynomial
kernels via explicit feature maps. In Proceedings of
the 19th ACM SIGKDD International Conference on
Knowledge Discovery and Data Mining, pages 239–
247. ACM.
Piergiovanni, A. and Ryoo, M. S. (2019). Representation
flow for action recognition. In Proceedings of the IEEE
Conference on Computer Vision and Pattern Recogni-
tion, pages 9945–9953.
Redmon, J. and Farhadi, A. (2017). Yolo9000: better, faster,
stronger. arXiv preprint.
S
´
anchez, J., Perronnin, F., Mensink, T., and Verbeek, J.
(2013). Image classification with the fisher vector:
Theory and practice. International journal of computer
vision, 105(3):222–245.
Simonyan, K. and Zisserman, A. (2014). Two-stream convo-
lutional networks for action recognition in videos. In
Advances in Neural Information Processing Systems,
pages 568–576.
Soomro, K., Zamir, A. R., and Shah, M. (2012). UCF101:
A dataset of 101 human actions classes from videos in
the wild. arXiv preprint arXiv:1212.0402.
Sun, C., Shetty, S., Sukthankar, R., and Nevatia, R. (2015).
Temporal localization of fine-grained actions in videos
by domain transfer from web images. In Proceedings of
the 23rd ACM International Conference on Multimedia,
pages 371–380. ACM.
Szegedy, C., Vanhoucke, V., Ioffe, S., Shlens, J., and Wojna,
Z. (2016). Rethinking the inception architecture for
computer vision. In Proceedings of the IEEE Con-
ference on Computer Vision and Pattern Recognition,
pages 2818–2826.
Tenenbaum, J. B. and Freeman, W. T. (2000). Separating
style and content with bilinear models. Neural compu-
tation, 12(6):1247–1283.
Tran, D., Bourdev, L., Fergus, R., Torresani, L., and Paluri,
M. (2015). Learning spatiotemporal features with 3d
convolutional networks. In Proceedings of the IEEE
International Conference on Computer Vision, pages
4489–4497.
Tran, D., Ray, J., Shou, Z., Chang, S.-F., and Paluri, M.
(2017). Convnet architecture search for spatiotemporal
feature learning. arXiv preprint arXiv:1708.05038.
Varol, G., Laptev, I., and Schmid, C. (2018). Long-term
temporal convolutions for action recognition. IEEE
Transactions on Pattern Analysis and Machine Intelli-
gence, 40(6):1510–1517.
Wang, L., Xiong, Y., Wang, Z., Qiao, Y., Lin, D., Tang, X.,
and Van Gool, L. (2016). Temporal segment networks:
Towards good practices for deep action recognition.
In European Conference on Computer Vision, pages
20–36. Springer.
Wu, C.-Y., Zaheer, M., Hu, H., Manmatha, R., Smola, A. J.,
and Kr
¨
ahenb
¨
uhl, P. (2018). Compressed video action
recognition. In Proceedings of the IEEE Conference
on Computer Vision and Pattern Recognition, pages
6026–6035.
Yu, C., Zhao, X., Zheng, Q., Zhang, P., and You, X. (2018).
Hierarchical bilinear pooling for fine-grained visual
recognition. In European Conference on Computer
Vision, pages 595–610. Springer.
Yue-Hei Ng, J., Hausknecht, M., Vijayanarasimhan, S.,
Vinyals, O., Monga, R., and Toderici, G. (2015). Be-
yond short snippets: Deep networks for video classi-
fication. In Proceedings of the IEEE Conference on
Computer Vision and Pattern Recognition, pages 4694–
4702.
Zhang, Y., Tang, S., Muandet, K., Jarvers, C., and Neumann,
H. (2019). Local temporal bilinear pooling for fine-
grained action parsing. In The IEEE Conference on
Computer Vision and Pattern Recognition (CVPR).
VISAPP 2021 - 16th International Conference on Computer Vision Theory and Applications
644