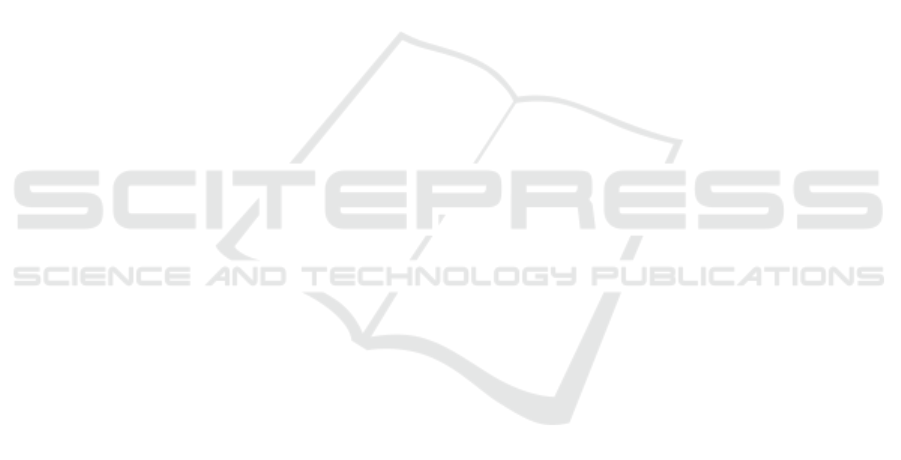
puter vision and pattern recognition, pages 248–255.
Ieee.
Ebert, F., Finn, C., Lee, A. X., and Levine, S. (2017). Self-
supervised visual planning with temporal skip connec-
tions. arXiv preprint arXiv:1710.05268.
Faktor, A. and Irani, M. (2014). Video segmentation by
non-local consensus voting. In BMVC, page 8.
Grundmann, M., Kwatra, V., Han, M., and Essa, I. (2010).
Efficient hierarchical graph-based video segmenta-
tion. In 2010 ieee computer society conference on
computer vision and pattern recognition, pages 2141–
2148. IEEE.
Hayder, Z., He, X., and Salzmann, M. (2017). Boundary-
aware instance segmentation. In Proceedings of the
IEEE Conference on Computer Vision and Pattern
Recognition, pages 5696–5704.
He, K., Gkioxari, G., Doll
´
ar, P., and Girshick, R. (2017).
Mask r-cnn. In Proceedings of the IEEE international
conference on computer vision, pages 2961–2969.
He, K., Zhang, X., Ren, S., and Sun, J. (2016). Deep resid-
ual learning for image recognition. In Proceedings of
the IEEE conference on computer vision and pattern
recognition, pages 770–778.
Ilg, E., Mayer, N., Saikia, T., Keuper, M., Dosovitskiy, A.,
and Brox, T. (2017). Flownet 2.0: Evolution of optical
flow estimation with deep networks. In Proceedings of
the IEEE conference on computer vision and pattern
recognition, pages 2462–2470.
Jain, S. D. and Grauman, K. (2014). Supervoxel-consistent
foreground propagation in video. In European confer-
ence on computer vision, pages 656–671. Springer.
Johnander, J., Danelljan, M., Brissman, E., Khan, F. S., and
Felsberg, M. (2019). A generative appearance model
for end-to-end video object segmentation. In Proceed-
ings of the IEEE Conference on Computer Vision and
Pattern Recognition, pages 8953–8962.
Kingma, D. P. and Ba, J. (2014). Adam: A
method for stochastic optimization. arXiv preprint
arXiv:1412.6980.
Krizhevsky, A., Sutskever, I., and Hinton, G. E. (2012). Im-
agenet classification with deep convolutional neural
networks. In Advances in neural information process-
ing systems, pages 1097–1105.
Li, X., Qi, Y., Wang, Z., Chen, K., Liu, Z., Shi, J., Luo,
P., Tang, X., and Loy, C. C. (2017). Video object
segmentation with re-identification. arXiv preprint
arXiv:1708.00197.
Luiten, J., Voigtlaender, P., and Leibe, B. (2018). Pre-
mvos: Proposal-generation, refinement and merging
for video object segmentation. In Asian Conference
on Computer Vision, pages 565–580. Springer.
Maninis, K.-K., Caelles, S., Chen, Y., Pont-Tuset, J., Leal-
Taix
´
e, L., Cremers, D., and Van Gool, L. (2018).
Video object segmentation without temporal informa-
tion. IEEE Transactions on Pattern Analysis and Ma-
chine Intelligence (TPAMI).
Maninis, K.-K., Pont-Tuset, J., Arbel
´
aez, P., and Van Gool,
L. (2016). Deep retinal image understanding. In In-
ternational conference on medical image computing
and computer-assisted intervention, pages 140–148.
Springer.
M
¨
arki, N., Perazzi, F., Wang, O., and Sorkine-Hornung, A.
(2016). Bilateral space video segmentation. In Pro-
ceedings of the IEEE Conference on Computer Vision
and Pattern Recognition, pages 743–751.
Oh, S. W., Lee, J.-Y., Xu, N., and Kim, S. J. (2019). Video
object segmentation using space-time memory net-
works. In Proceedings of the IEEE International Con-
ference on Computer Vision, pages 9226–9235.
Papazoglou, A. and Ferrari, V. (2013). Fast object segmen-
tation in unconstrained video. In Proceedings of the
IEEE International Conference on Computer Vision,
pages 1777–1784.
Peng, C., Zhang, X., Yu, G., Luo, G., and Sun, J. (2017).
Large kernel matters–improve semantic segmentation
by global convolutional network. In Proceedings of
the IEEE conference on computer vision and pattern
recognition, pages 4353–4361.
Perazzi, F., Khoreva, A., Benenson, R., Schiele, B., and
Sorkine-Hornung, A. (2017). Learning video object
segmentation from static images. In Proceedings of
the IEEE Conference on Computer Vision and Pattern
Recognition, pages 2663–2672.
Perazzi, F., Pont-Tuset, J., McWilliams, B., Van Gool, L.,
Gross, M., and Sorkine-Hornung, A. (2016a). A
benchmark dataset and evaluation methodology for
video object segmentation. In Computer Vision and
Pattern Recognition.
Perazzi, F., Pont-Tuset, J., McWilliams, B., Van Gool, L.,
Gross, M., and Sorkine-Hornung, A. (2016b). A
benchmark dataset and evaluation methodology for
video object segmentation. In Proceedings of the
IEEE Conference on Computer Vision and Pattern
Recognition, pages 724–732.
Pont-Tuset, J., Perazzi, F., Caelles, S., Arbel
´
aez, P.,
Sorkine-Hornung, A., and Van Gool, L. (2017). The
2017 davis challenge on video object segmentation.
arXiv preprint arXiv:1704.00675.
Ronneberger, O., Fischer, P., and Brox, T. (2015). U-net:
Convolutional networks for biomedical image seg-
mentation. In International Conference on Medical
image computing and computer-assisted intervention,
pages 234–241. Springer.
Shankar Nagaraja, N., Schmidt, F. R., and Brox, T. (2015).
Video segmentation with just a few strokes. In Pro-
ceedings of the IEEE International Conference on
Computer Vision, pages 3235–3243.
Simonyan, K. and Zisserman, A. (2014). Very deep con-
volutional networks for large-scale image recognition.
arXiv preprint arXiv:1409.1556.
Tokmakov, P., Alahari, K., and Schmid, C. (2017). Learn-
ing video object segmentation with visual memory. In
Proceedings of the IEEE International Conference on
Computer Vision, pages 4481–4490.
Ventura, C., Bellver, M., Girbau, A., Salvador, A., Mar-
ques, F., and Giro-i Nieto, X. (2019). Rvos: End-to-
end recurrent network for video object segmentation.
In Proceedings of the IEEE Conference on Computer
Vision and Pattern Recognition, pages 5277–5286.
Hybrid-S2S: Video Object Segmentation with Recurrent Networks and Correspondence Matching
191