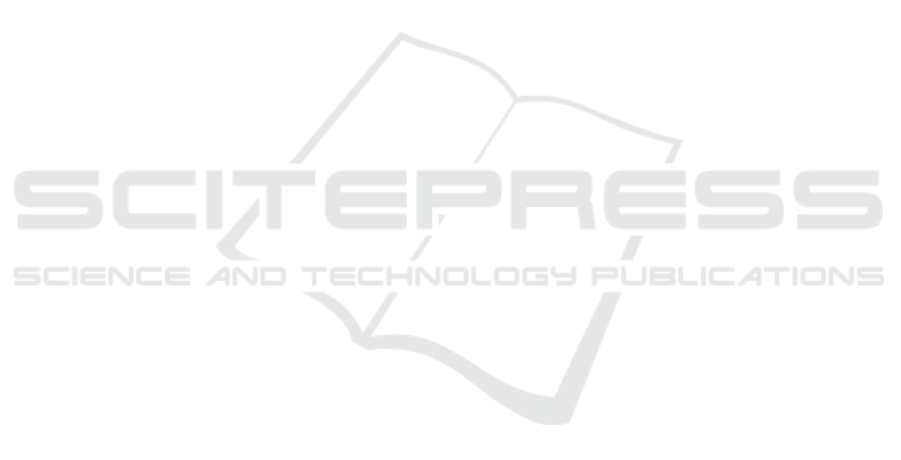
conference on computer vision and pattern recogni-
tion, pages 5297–5307.
Bromley, J., Guyon, I., LeCun, Y., S
¨
ackinger, E., and Shah,
R. (1994). Signature verification using a” siamese”
time delay neural network. In Advances in neural in-
formation processing systems, pages 737–744.
Chen, W., Chen, X., Zhang, J., and Huang, K. (2017). Be-
yond triplet loss: a deep quadruplet network for per-
son re-identification. In Proceedings of the IEEE Con-
ference on Computer Vision and Pattern Recognition,
pages 403–412.
Choy, C., Gwak, J., and Savarese, S. (2019a). 4d spatio-
temporal convnets: Minkowski convolutional neural
networks. In Proceedings of the IEEE Conference
on Computer Vision and Pattern Recognition, pages
3075–3084.
Choy, C., Park, J., and Koltun, V. (2019b). Fully convolu-
tional geometric features. In Proceedings of the IEEE
International Conference on Computer Vision, pages
8958–8966.
Dai, A., Chang, A. X., Savva, M., Halber, M., Funkhouser,
T., and Nießner, M. (2017). Scannet: Richly-
annotated 3d reconstructions of indoor scenes. In
Proc. Computer Vision and Pattern Recognition
(CVPR), IEEE.
Hermans, A., Beyer, L., and Leibe, B. (2017). In defense
of the triplet loss for person re-identification. arXiv
preprint arXiv:1703.07737.
Komorowski, J. (2020). Minkloc3d: Point cloud
based large-scale place recognition. arXiv preprint
arXiv:2011.04530.
Lee, J.-E., Jin, R., and Jain, A. K. (2008). Rank-based
distance metric learning: An application to image re-
trieval. In 2008 IEEE Conference on Computer Vision
and Pattern Recognition, pages 1–8. IEEE.
Lin, T.-Y., Doll
´
ar, P., Girshick, R., He, K., Hariharan, B.,
and Belongie, S. (2017). Feature pyramid networks
for object detection. In Proceedings of the IEEE con-
ference on computer vision and pattern recognition,
pages 2117–2125.
Liu, Z., Zhou, S., Suo, C., Yin, P., Chen, W., Wang, H.,
Li, H., and Liu, Y.-H. (2019). Lpd-net: 3d point cloud
learning for large-scale place recognition and environ-
ment analysis. In Proceedings of the IEEE Interna-
tional Conference on Computer Vision, pages 2831–
2840.
Lu, J., Hu, J., and Zhou, J. (2017). Deep metric learning
for visual understanding: An overview of recent ad-
vances. IEEE Signal Processing Magazine, 34(6):76–
84.
Maturana, D. and Scherer, S. (2015). Voxnet: A 3d con-
volutional neural network for real-time object recog-
nition. In 2015 IEEE/RSJ International Conference
on Intelligent Robots and Systems (IROS), pages 922–
928. IEEE.
Qi, C. R., Su, H., Mo, K., and Guibas, L. J. (2017a). Point-
net: Deep learning on point sets for 3d classification
and segmentation. In Proceedings of the IEEE con-
ference on computer vision and pattern recognition,
pages 652–660.
Qi, C. R., Yi, L., Su, H., and Guibas, L. J. (2017b). Point-
net++: Deep hierarchical feature learning on point sets
in a metric space. In Advances in neural information
processing systems, pages 5099–5108.
Su, H., Maji, S., Kalogerakis, E., and Learned-Miller, E.
(2015). Multi-view convolutional neural networks for
3d shape recognition. In Proceedings of the IEEE
international conference on computer vision, pages
945–953.
Wang, Z. and Jia, K. (2019). Frustum convnet: Slid-
ing frustums to aggregate local point-wise features
for amodal. In 2019 IEEE/RSJ International Confer-
ence on Intelligent Robots and Systems (IROS), pages
1742–1749. IEEE.
Wu, C.-Y., Manmatha, R., Smola, A. J., and Krahenbuhl, P.
(2017). Sampling matters in deep embedding learn-
ing. In Proceedings of the IEEE International Confer-
ence on Computer Vision, pages 2840–2848.
Zeng, A., Song, S., Nießner, M., Fisher, M., Xiao, J., and
Funkhouser, T. (2017). 3dmatch: Learning the match-
ing of local 3d geometry in range scans. In CVPR,
volume 1.
Zhang, W. and Xiao, C. (2019). Pcan: 3d attention map
learning using contextual information for point cloud
based retrieval. In Proceedings of the IEEE Confer-
ence on Computer Vision and Pattern Recognition,
pages 12436–12445.
Zhong, Z., Zheng, L., Kang, G., Li, S., and Yang, Y. (2017).
Random erasing data augmentation. arXiv preprint
arXiv:1708.04896.
VISAPP 2021 - 16th International Conference on Computer Vision Theory and Applications
224