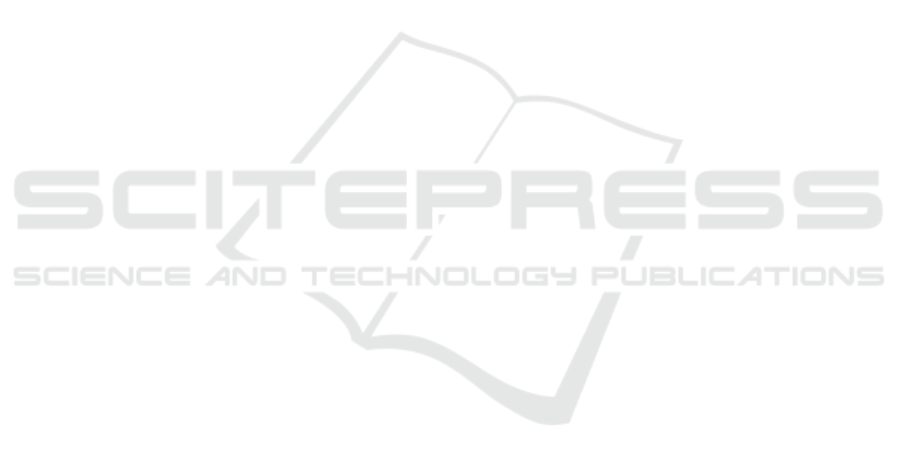
ACKNOWLEDGEMENTS
This work has been supported by the European So-
cial Fund (ESF) and the Ministry of Education, Sci-
ence and Culture of Mecklenburg-Vorpommern, Ger-
many under the project ”DigIT!” (ESF/14-BM-A55-
0015/19).
REFERENCES
Barrowclough, G., Cracraft, J., Klicka, J., and Zink, R.
(2016). How many kinds of birds are there and why
does it matter? PLoS ONE, 11.
Bendale, A. and Boult, T. E. (2016). Towards open set deep
networks. In Proceedings of the IEEE conference on
computer vision and pattern recognition, pages 1563–
1572.
Berg, T. and Belhumeur, P. N. (2013). Poof: Part-based one-
vs.-one features for fine-grained categorization, face
verification, and attribute estimation. In Proceedings
of the IEEE Conference on Computer Vision and Pat-
tern Recognition, pages 955–962.
Chen, X., Lan, X., Sun, F., and Zheng, N. (2020). A bound-
ary based out-of-distribution classifier for generalized
zero-shot learning. In Proceedings of the European
Conference on Computer Vision (ECCV).
Fu, J., Zheng, H., and Mei, T. (2017). Look closer to see
better: Recurrent attention convolutional neural net-
work for fine-grained image recognition. 2017 IEEE
Conference on Computer Vision and Pattern Recogni-
tion (CVPR), pages 4476–4484.
Geifman, Y. and El-Yaniv, R. (2019). Selectivenet: A deep
neural network with an integrated reject option. arXiv
preprint arXiv:1901.09192.
Goodfellow, I. J., Shlens, J., and Szegedy, C. (2014). Ex-
plaining and harnessing adversarial examples. arXiv
preprint arXiv:1412.6572.
Guo, C., Pleiss, G., Sun, Y., and Weinberger, K. Q. (2017).
On calibration of modern neural networks. ArXiv,
abs/1706.04599.
He, K., Zhang, X., Ren, S., and Sun, J. (2016). Deep resid-
ual learning for image recognition. In Proceedings of
the IEEE Conference on Computer Vision and Pattern
Recognition (CVPR).
Hendrycks, D. and Gimpel, K. (2017). A baseline for de-
tecting misclassified and out-of-distribution examples
in neural networks. ICLR, abs/1610.02136.
Hendrycks, D., Mazeika, M., and Dietterich, T. (2018).
Deep anomaly detection with outlier exposure. In In-
ternational Conference on Learning Representations.
Khosla, A., Jayadevaprakash, N., Yao, B., and Fei-Fei, L.
(2011). Novel dataset for fine-grained image catego-
rization. In First Workshop on Fine-Grained Visual
Categorization, IEEE Conference on Computer Vision
and Pattern Recognition, Colorado Springs, CO.
Krause, J., Stark, M., Deng, J., and Fei-Fei, L. (2013). 3d
object representations for fine-grained categorization.
In 4th International IEEE Workshop on 3D Represen-
tation and Recognition (3dRR-13), Sydney, Australia.
Lee, K., Lee, K., Lee, H., and Shin, J. (2018). A simple uni-
fied framework for detecting out-of-distribution sam-
ples and adversarial attacks. In Advances in Neural
Information Processing Systems, pages 7167–7177.
Liang, S., Li, Y., and Srikant, R. (2018). Enhancing the reli-
ability of out-of-distribution image detection in neural
networks. In International Conference on Learning
Representations.
Lin, D., Shen, X., Lu, C., and Jia, J. (2015). Deep lac:
Deep localization, alignment and classification for
fine-grained recognition. In Proceedings of the IEEE
conference on computer vision and pattern recogni-
tion, pages 1666–1674.
Maji, S., Kannala, J., Rahtu, E., Blaschko, M., and Vedaldi,
A. (2013). Fine-grained visual classification of air-
craft. Technical report.
Nguyen, A., Yosinski, J., and Clune, J. (2015). Deep neural
networks are easily fooled: High confidence predic-
tions for unrecognizable images. In Proceedings of
the IEEE conference on computer vision and pattern
recognition, pages 427–436.
Nilsback, M.-E. and Zisserman, A. (2008). Automated
flower classification over a large number of classes.
In Proceedings of the Indian Conference on Computer
Vision, Graphics and Image Processing.
Parkhi, O. M., Vedaldi, A., Zisserman, A., and Jawahar,
C. V. (2012). Cats and dogs. In IEEE Conference
on Computer Vision and Pattern Recognition.
Ren, J., Liu, P. J., Fertig, E., Snoek, J., Poplin, R., De-
Pristo, M. A., Dillon, J. V., and Lakshminarayanan,
B. (2019). Likelihood ratios for out-of-distribution de-
tection. In NeurIPS.
Ren, S., He, K., Girshick, R., and Sun, J. (2015). Faster
r-cnn: Towards real-time object detection with region
proposal networks. In Advances in neural information
processing systems, pages 91–99.
Roady, R., Hayes, T. L., Kemker, R., Gonzales, A., and
Kanan, C. (2019). Are out-of-distribution detection
methods effective on large-scale datasets? arXiv
preprint arXiv:1910.14034.
Russakovsky, O., Deng, J., Su, H., Krause, J., Satheesh,
S., Ma, S., Huang, Z., Karpathy, A., Khosla, A.,
Bernstein, M., Berg, A. C., and Fei-Fei, L. (2015).
ImageNet Large Scale Visual Recognition Challenge.
International Journal of Computer Vision (IJCV),
115(3):211–252.
Van Horn, G., Branson, S., Farrell, R., Haber, S., Barry,
J., Ipeirotis, P., Perona, P., and Belongie, S. (2015).
Building a bird recognition app and large scale dataset
with citizen scientists: The fine print in fine-grained
dataset collection. In Proceedings of the IEEE Con-
ference on Computer Vision and Pattern Recognition
(CVPR).
Van Horn, G., Mac Aodha, O., Song, Y., Cui, Y., Sun, C.,
Shepard, A., Adam, H., Perona, P., and Belongie, S.
(2018). The inaturalist species classification and de-
tection dataset. In The IEEE Conference on Computer
Vision and Pattern Recognition (CVPR).
VISAPP 2021 - 16th International Conference on Computer Vision Theory and Applications
232