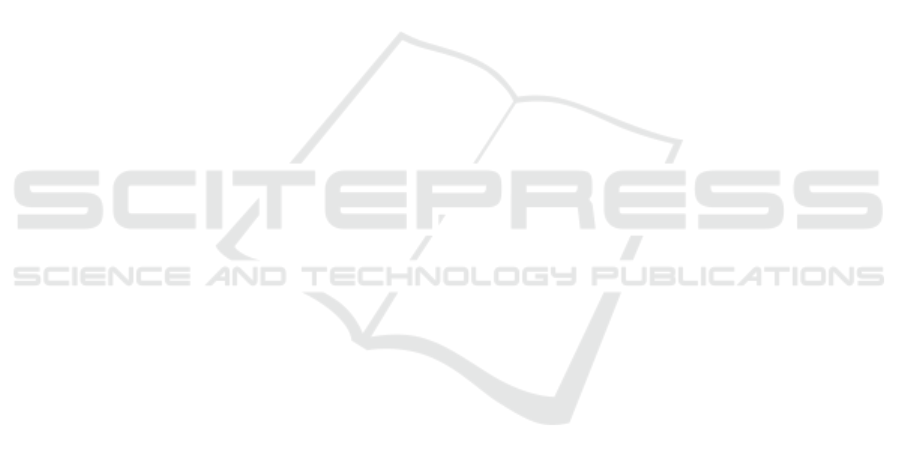
between ET and NCT/NET or NCT/NET and ED be-
cause all classes do not have the apparent shape or
have indefinite boundaries. The proposed method is
a promising direction for studying the feasibility of
generative networks with Bayesian active learning to
measure uncertainty in segmentation applications. In
the future, we aim to investigate the potential of the
proposed method to estimate uncertainty in other ap-
plications.
REFERENCES
Bakas, S., Akbari, H., Sotiras, A., Bilello, M., Rozycki,
M., Kirby, J. S., Freymann, J. B., Farahani, K., and
Davatzikos, C. (2017). Advancing the cancer genome
atlas glioma MRI collections with expert segmentation
labels and radiomic features. Scientific Data.
Eaton-Rosen, Z., Bragman, F., Bisdas, S., Ourselin, S., and
Cardoso, M. J. (2018). Towards safe deep learning:
accurately quantifying biomarker uncertainty in neural
network predictions. In Medical Image Computing and
Computer Assisted Intervention.
Gal, Y., Islam, R., and Ghahramani, Z. (2017). Deep
bayesian active learning with image data. In Proceed-
ings of the 34th International Conference on Machine
Learning, volume 70, pages 1183–1192.
Giacomello, E., Loiacono, D., and Mainardi, L. (2019).
Brain MRI tumor segmentation with adversarial net-
works. arXiv:1910.02717.
Goodfellow, I., Pouget-Abadie, J., Mirza, M., Xu, B., Warde-
Farley, D., Ozair, S., Courville, A., and Bengio, Y.
(2014). Generative adversarial nets. In Advances
in Neural Information Processing Systems 27, pages
2672–2680.
He, K., Zhang, X., Ren, S., and Sun, J. (2016). Deep residual
learning for image recognition. In IEEE Conference
on Computer Vision and Pattern Recognition, pages
770–778.
Isensee, F., Kickingereder, P., Wick, W., Bendszus, M., and
Maier-Hein, K. H. (2017). Brain tumor segmentation
and radiomics survival prediction: contribution to the
BRATS 2017 challenge. In 3rd International Work-
shop, BrainLes, Held in Conjunction with Medical
Image Computing for Computer Assisted Intervention,
volume 10670, page 287–297.
Isola, P., Zhu, J., Zhou, T., and Efros, A. A. (2017). Image-
to-image translation with conditional adversarial net-
works. In IEEE Conference on Computer Vision and
Pattern Recognition, pages 5967–5976.
Jungo, A., Meier, R., Ermis, E., Herrmann, E., and Reyes, M.
(2018). Uncertainty-driven sanity check: application to
postoperative brain tumor cavity segmentation. In 1st
Conference on Medical Imaging with Deep Learning.
Kendall, A. and Gal, Y. (2017). What uncertainties do we
need in bayesian deep learning for computer vision?
In Advances in Neural Information Processing Systems
30, pages 5574–5584.
Mazumdar, I. (2020). Automated brain tumour segmen-
tation using deep fully residual convolutional neural
networks. arXiv:1908.04250.
Menze, B., Jakab, A., Bauer, S., Kalpathy-Cramer, J., Fara-
haniy, K., Kirby, J., Burren, Y., Porz, N., Slotboomy, J.,
Wiest, R., Lancziy, L., Gerstnery, E., Webery, M.-A.,
Arbel, T., Avants, B., Ayache, N., Buendia, P., Collins,
L., Cordier, N., and Van Leemput, K. (2015). The mul-
timodal brain tumor image segmentation benchmark
(BRATS). IEEE Transactions on Medical Imaging,
34(10):1993–2024.
Myronenko, A. (2018). 3D MRI brain tumor segmentation
using autoencoder regularization. In 4rd International
Workshop on Brainlesion, BrainLes, Held in Conjunc-
tion with Medical Image Computing for Computer As-
sisted Intervention, volume 11384, pages 311–320.
Sauwen, N., Acou, M., Sima, D. M., Veraart, J., Maes, F.,
Himmelreich, U., Achten, E., and Huffel, S. V. (2017).
Semi-automated brain tumor segmentation on multi-
parametric MRI using regularized non-negative matrix
factorization. BMC Medical Imaging.
Sutherland, D. J., Tung, H., Strathmann, H., De, S., Ramdas,
A., Smola, A. J., and Gretton, A. (2017). Generative
models and model criticism via optimized maximum
mean discrepancy. In 5th International Conference on
Learning Representations.
Wang, G., Li, W., Aertsen, M., Deprest, J., Ourselin, S.,
and Vercauteren, T. (2019a). Aleatoric uncertainty
estimation with test-time augmentation for medical im-
age segmentation with convolutional neural networks.
Neurocomputing, 338:34 – 45.
Wang, G., Li, W., Ourselin, S., and Vercauteren, T. (2019b).
Automatic brain tumor segmentation based on cas-
caded convolutional neural networks with uncertainty
estimation. Frontiers in Computational Neuroscience,
13(56).
Wang, K., Zhang, D., Li, Y., Zhang, R., and Lin, L. (2017).
Cost-effective active learning for deep image classifi-
cation. IEEE Transactions on Circuits and Systems for
Video Technology, 27(12):2591–2600.
Xue, Y., Xu, T., Zhang, H., Long, L. R., and Huang, X.
(2018). SegAN: adversarial network with multi-scale
l1 loss for medical image segmentation. Neuroinfor-
matics, 16:383–392.
Quantification of Uncertainty in Brain Tumor Segmentation using Generative Network and Bayesian Active Learning
709