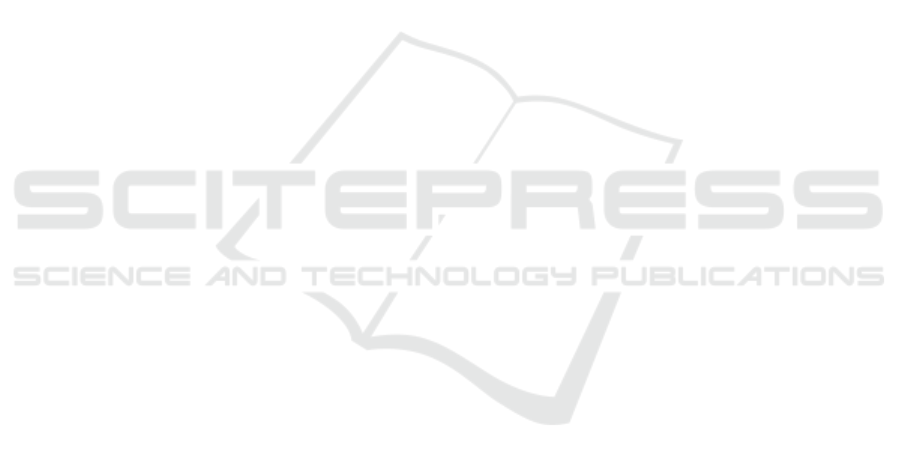
Dansana, D., Kumar, R., et al. (2020). Early diagnosis of
covid-19-affected patients based on x-ray and com-
puted tomography images using deep learning algo-
rithm. Soft Computing.
Demner-Fushman, D., Kohli, M., et al. (2015). Preparing
a collection of radiology examinations for distribution
and retrieval. JAMIA, 23.
Deng, J., Dong, W., et al. (2009). ImageNet: A Large-Scale
Hierarchical Image Database. In CVPR09.
Forthmann, P. and Pfleiderer, G. (2019). Augmented dis-
play device for use in a medical imaging laboratory.
US2018197337 Google Patents.
Gangavarapu, T., Krishnan, G. S., Kamath, S., and Je-
ganathan, J. (2020). Farsight: Long-term disease
prediction using unstructured clinical nursing notes.
IEEE Transactions on Emerging Topics in Computing.
Ghoshal, B. and Tucker, A. (2020). Estimating un-
certainty and interpretability in deep learning for
coronavirus (COVID-19) detection. arXiv preprint
arXiv:2003.10769.
Gonzalez, R. C. and Woods, R. E. (2008). Digital image
processing. Prentice Hall, Upper Saddle River, N.J.
Hall, L. O., Paul, R., Goldgof, D. B., and Goldgof, G. M.
(2020). Finding covid-19 from chest x-rays using
deep learning on a small dataset. arXiv e-prints, page
arXiv:2004.02060.
He, K., Zhang, X., et al. (2015). Deep residual learning for
image recognition.
Hemdan, E.-D., Shouman, M., and Karar, M. E. (2020).
Covidx-net: A framework of deep learning classi-
fiers to diagnose covid-19 in x-ray images. arXiv
preprint:2003.11055.
Isola, P., Zhu, J.-Y., Zhou, T., and Efros, A. A. (2018).
Image-to-image translation with conditional adversar-
ial networks.
Krizhevsky, A. (2012). Convolutional deep belief networks
on cifar-10.
Max Roser, Hannah Ritchie, E. O.-O. and Hasell, J. (2020).
Coronavirus pandemic (COVID-19). Our World in
Data.
Mei, X., Lee, H.-C., et al. (2020). Artificial intelli-
gence–enabled rapid diagnosis of patients with covid-
19. Nature Medicine, 26:1–5.
Mikolov, T., Chen, K., Corrado, G., and Dean, J. (2013).
Efficient Estimation of Word Representations in Vec-
tor Space. arXiv e-prints, page arXiv:1301.3781.
Mishra, A., Das, S., et al. (2020). Identifying covid19
from chest ct images: A deep convolutional neural
networks based approach. Journal of Healthcare En-
gineering, 2020:1–7.
Narin, A., Kaya, C., and Pamuk, Z. (2020). Automatic de-
tection of coronavirus disease (COVID-19) using x-
ray images and deep convolutional neural networks.
arxiv preprint:2003.10849.
Ozturk, T., Talo, M., et al. (2020). Automated detection
of COVID-19 cases using deep neural networks with
x-ray images. Computers in Biology and Medicine.
Paszke, A., Gross, S., Massa, F., et al. (2019). Pytorch:
An imperative style, high-performance deep learning
library. In Advances in Neural Information Processing
Systems 32.
Radiological Society of North America (2018). Rsna pneu-
monia detection challenge. https://www.kaggle.com/
c/rsna-pneumonia-detection-challenge. Accessed:
2020-08-11.
Rahman, T. (2020). COVID-19 radiography database.
ˇ
Reh˚u
ˇ
rek, R. and Sojka, P. (2010). Software Framework for
Topic Modelling with Large Corpora. In LREC 2010
Workshop on New Challenges for NLP Frameworks.
ELRA.
Reza, A. (2004). Realization of the contrast limited adap-
tive histogram equalization (clahe) for real-time image
enhancement. VLSI Signal Processing, 38:35–44.
Ronneberger, O., Fischer, P., and Brox, T. (2015). U-
net: Convolutional networks for biomedical im-
age segmentation. In Medical Image Computing
and Computer-Assisted Intervention – MICCAI 2015,
pages 234–241. Springer.
Scheib, S. (2009). Dosimetric end-to-end verification
devices, systems, and methods. US 20150085993
Google Patents.
Selvaraju, R., Cogswell, M., et al. (2017). Grad-cam: Vi-
sual explanations from deep networks via gradient-
based localization. In IEEE international conference
on computer vision, pages 618–626.
Son, J., Park, S. J., and Jung, K.-H. (2017). Retinal Ves-
sel Segmentation in Fundoscopic Images with Gen-
erative Adversarial Networks. arXiv e-prints, page
arXiv:1706.09318.
Tushaar, G., Jayasimha, A., Krishnan, G. S., and Kamath,
S. (2020). Predicting icd-9 code groups with fuzzy
similarity based supervised multi-label classification
of unstructured clinical nursing notes. Knowledge-
Based Systems, 190:105321.
Zijdenbos, A., Dawant, B., Margolin, R., and Palmer, A. C.
(1994). Morphometric analysis of white matter lesions
in mr images: method and validation. IEEE transac-
tions on medical imaging, 13 4:716–24.
HEALTHINF 2021 - 14th International Conference on Health Informatics
666