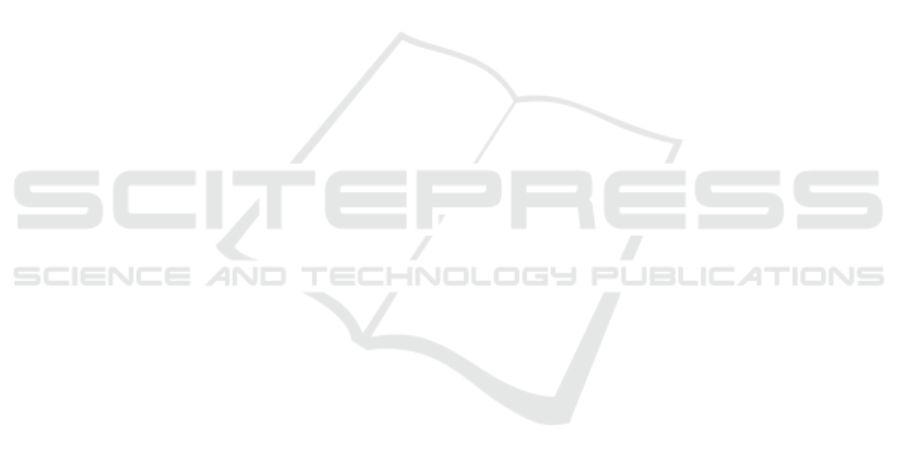
more, a flexible and easy to install monitoring sys-
tem for various shrimp production systems was pre-
sented. By means of the proposed automated moni-
toring approach, a more time and cost efficient shrimp
production can be achieved to increase the efficiency
of the shrimp farming process. Certainly, as men-
tioned in the previous section, there is room for im-
provement within all three mentioned use-cases. This
could be a subject for future research. Additionally,
it became clear that the designed system should cover
a significantly larger area than 40 × 20 cm. Approx-
imately 100 × 100 cm would be a better fit, which
would make the counting task more meaningful. In
addition to all the considered use-cases, an automated
detection of anomalies in the external appearance of
shrimp could be an interesting use-case for future
works.
ACKNOWLEDGEMENTS
Funding (code: B 730217000011) for ASV came
from the European Fisheries Fund (EFF) and the State
Mecklenburg-Vorpommern.
REFERENCES
Awalludin, E. A., Mat Yaziz, M. Y., Abdul Rahman, N. R.,
Yussof, W. N. J. H. W., Hitam, M. S., and T Ar-
sad, T. N. (2019). Combination of canny edge detec-
tion and blob processing techniques for shrimp larvae
counting. In 2019 IEEE International Conference on
Signal and Image Processing Applications (ICSIPA),
pages 308–313.
Beseres, J. J., Lawrence, A. L., and Feller, R. J. (2005).
Variation in fiber, protein and lipid content of shrimp
feed – effects on gut-passage time measured in the
field. Journal of Shellfish Research, 24(1):301–308.
Publisher: National Shellfisheries Association.
Boone, L. (1931). Anomuran, macruran Crustacea from
Panama and Canal Zone. Number 63 in Bulletin of
the American Museum of Natural History. American
Museum of Natural History.
Davie, P. J. F., Guinot, D., and Ng, P. K. L. (2015). Anatomy
and functional morphology of Brachyura. Treatise
on Zoology - Anatomy, Taxonomy, Biology. The Crus-
tacea, Volume 9 Part C (2 vols), pages 11–163. Pub-
lisher: Brill Section: Treatise on Zoology - Anatomy,
Taxonomy, Biology. The Crustacea, Volume 9 Part C
(2 vols).
FAO (2020). The State of World Fisheries and Aquaculture
2020: Sustainability in action. FAO, Rome.
Franceschini-Vicentini, I. B., Ribeiro, K., Papa, L. P., Mar-
ques Junior, J., Vicentini, C. A., and Valenti, P. M.
C. M. (2009). Histoarchitectural Features of the
Hepatopancreas of the Amazon River Prawn Macro-
brachium amazonicum. International Journal of Mor-
phology, 27(1):121–128.
Harbitz, A. (2007). Estimation of shrimp (Pandalus bore-
alis) carapace length by image analysis. ICES Journal
of Marine Science, 64(5):939–944.
He, K., Gkioxari, G., Doll
´
ar, P., and Girshick, R. (2017).
Mask r-cnn. In 2017 IEEE International Conference
on Computer Vision (ICCV), pages 2980–2988.
He, K., Zhang, X., Ren, S., and Sun, J. (2016). Deep resid-
ual learning for image recognition. In 2016 IEEE Con-
ference on Computer Vision and Pattern Recognition
(CVPR), pages 770–778.
Kaewchote, J., Janyong, S., and Limprasert, W. (2018).
Image recognition method using local binary pattern
and the random forest classifier to count post larvae
shrimp. Agriculture and Natural Resources, 52(4):371
– 376.
Khantuwan, W. and Khiripet, N. (2012). Live shrimp
larvae counting method using co-occurrence color
histogram. In 2012 9th International Confer-
ence on Electrical Engineering/Electronics, Com-
puter, Telecommunications and Information Technol-
ogy, pages 1–4.
Lin, T., Doll
´
ar, P., Girshick, R., He, K., Hariharan, B., and
Belongie, S. (2017). Feature pyramid networks for
object detection. In 2017 IEEE Conference on Com-
puter Vision and Pattern Recognition (CVPR), pages
936–944.
Nguyen, K., Nguyen, C., Wang, C., and Wang, J. (2020).
Two-phase instance segmentation for whiteleg shrimp
larvae counting. In 2020 IEEE International Confer-
ence on Consumer Electronics (ICCE), pages 1–3.
O’Mahony, N., Campbell, S., Carvalho, A., Harapanahalli,
S., Hernandez, G. V., Krpalkova, L., Riordan, D., and
Walsh, J. (2019). Deep learning vs. traditional com-
puter vision. In Science and Information Conference,
pages 128–144. Springer.
Ren, S., He, K., Girshick, R., and Sun, J. (2017). Faster
R-CNN: Towards real-time object detection with re-
gion proposal networks. IEEE Transactions on Pat-
tern Analysis and Machine Intelligence, 39(6):1137–
1149.
Roy, L. A., Davis, D. A., and Whitis, G. N. (2012). Ef-
fect of Feeding Rate and Pond Primary Productivity
on Growth of Litopenaeus vannamei Reared in In-
land Saline Waters of West Alabama. North American
Journal of Aquaculture, 74(1):20–26.
Solahudin, M., Slamet, W., and Dwi, A. S. (2018). Vaname
(litopenaeus vannamei) shrimp fry counting based on
image processing method. IOP Conference Series:
Earth and Environmental Science, 147:012014.
Yeh, C.-T. and Chen, M.-C. (2019). A combination of iot
and cloud application for automatic shrimp counting.
Microsystem Technologies, pages 1–8.
VISAPP 2021 - 16th International Conference on Computer Vision Theory and Applications
716