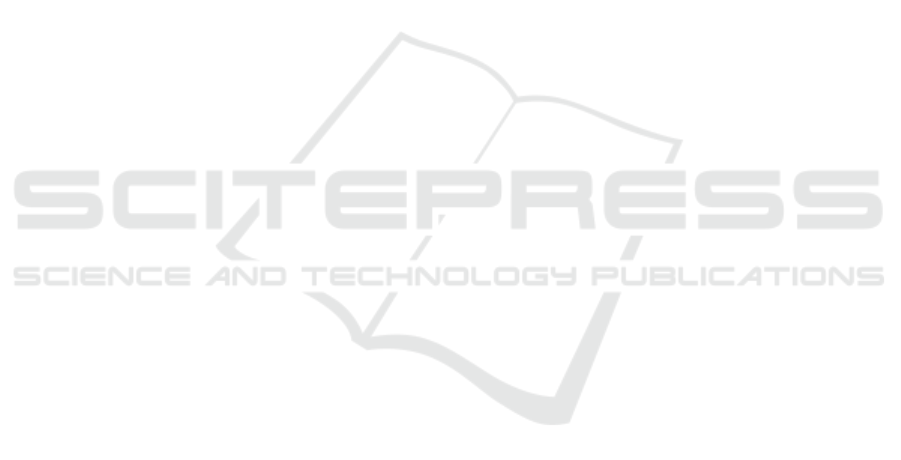
REFERENCES
Bailer, C., Taetz, B., and Stricker, D. (2015). Flow fields:
Dense correspondence fields for highly accurate large
displacement optical flow estimation. In Proceedings
of the IEEE international conference on computer vi-
sion, pages 4015–4023.
Brox, T. and Malik, J. (2010). Large displacement optical
flow: descriptor matching in variational motion esti-
mation. IEEE transactions on pattern analysis and
machine intelligence, 33(3):500–513.
Butler, D. J., Wulff, J., Stanley, G. B., and Black, M. J.
(2012). A naturalistic open source movie for optical
flow evaluation. In A. Fitzgibbon et al. (Eds.), editor,
European Conf. on Computer Vision (ECCV), Part IV,
LNCS 7577, pages 611–625. Springer-Verlag.
Cho, K., Van Merri
¨
enboer, B., Gulcehre, C., Bahdanau, D.,
Bougares, F., Schwenk, H., and Bengio, Y. (2014).
Learning phrase representations using rnn encoder-
decoder for statistical machine translation. arXiv
preprint arXiv:1406.1078.
Eigen, D., Puhrsch, C., and Fergus, R. (2014). Depth map
prediction from a single image using a multi-scale
deep network. In Advances in neural information pro-
cessing systems, pages 2366–2374.
Eldesokey, A., Felsberg, M., Holmquist, K., and Persson,
M. (2020). Uncertainty-aware cnns for depth comple-
tion: Uncertainty from beginning to end. In Proceed-
ings of the IEEE/CVF Conference on Computer Vision
and Pattern Recognition, pages 12014–12023.
Eldesokey, A., Felsberg, M., and Khan, F. S. (2018). Prop-
agating confidences through cnns for sparse data re-
gression. In The British Machine Vision Conference
(BMVC), Northumbria University, Newcastle upon
Tyne, England, UK, 3-6 September, 2018.
Eldesokey, A., Felsberg, M., and Khan, F. S. (2019). Con-
fidence propagation through cnns for guided sparse
depth regression. IEEE transactions on pattern anal-
ysis and machine intelligence.
Fischer, P., Dosovitskiy, A., Ilg, E., H
¨
ausser, P., Hazırbas¸,
C., Golkov, V., Van der Smagt, P., Cremers, D.,
and Brox, T. (2015). Flownet: Learning optical
flow with convolutional networks. arXiv preprint
arXiv:1504.06852.
Horn, B. K. and Schunck, B. G. (1981). Determining op-
tical flow. In Techniques and Applications of Image
Understanding, volume 281, pages 319–331. Interna-
tional Society for Optics and Photonics.
Hui, T.-W., Tang, X., and Change Loy, C. (2018). Lite-
flownet: A lightweight convolutional neural network
for optical flow estimation. In Proceedings of the
IEEE conference on computer vision and pattern
recognition, pages 8981–8989.
Hur, J. and Roth, S. (2019). Iterative residual refinement for
joint optical flow and occlusion estimation. In Pro-
ceedings of the IEEE Conference on Computer Vision
and Pattern Recognition, pages 5754–5763.
Ilg, E., Mayer, N., Saikia, T., Keuper, M., Dosovitskiy, A.,
and Brox, T. (2017). Flownet 2.0: Evolution of optical
flow estimation with deep networks. In Proceedings of
the IEEE conference on computer vision and pattern
recognition, pages 2462–2470.
Kondermann, D., Nair, R., Honauer, K., Krispin, K., An-
drulis, J., Brock, A., Gussefeld, B., Rahimimoghad-
dam, M., Hofmann, S., Brenner, C., et al. (2016). The
hci benchmark suite: Stereo and flow ground truth
with uncertainties for urban autonomous driving. In
Proceedings of the IEEE Conference on Computer Vi-
sion and Pattern Recognition Workshops, pages 19–
28.
Li, Y., Huang, J.-B., Ahuja, N., and Yang, M.-H. (2019).
Joint image filtering with deep convolutional net-
works. IEEE transactions on pattern analysis and ma-
chine intelligence, 41(8):1909–1923.
Mayer, N., Ilg, E., H
¨
ausser, P., Fischer, P., Cremers, D.,
Dosovitskiy, A., and Brox, T. (2016). A large dataset
to train convolutional networks for disparity, optical
flow, and scene flow estimation. In IEEE International
Conference on Computer Vision and Pattern Recogni-
tion (CVPR). arXiv:1512.02134.
Menze, M., Heipke, C., and Geiger, A. (2018). Object scene
flow. ISPRS Journal of Photogrammetry and Remote
Sensing (JPRS).
Su, H., Jampani, V., Sun, D., Gallo, O., Learned-Miller,
E., and Kautz, J. (2019). Pixel-adaptive convolutional
neural networks. In Proceedings of the IEEE Con-
ference on Computer Vision and Pattern Recognition,
pages 11166–11175.
Sun, D., Yang, X., Liu, M.-Y., and Kautz, J. (2018). Pwc-
net: Cnns for optical flow using pyramid, warping,
and cost volume. In Proceedings of the IEEE con-
ference on computer vision and pattern recognition,
pages 8934–8943.
Sun, D., Yang, X., Liu, M.-Y., and Kautz, J. (2019). Models
matter, so does training: An empirical study of cnns
for optical flow estimation. IEEE transactions on pat-
tern analysis and machine intelligence, 42(6):1408–
1423.
Teed, Z. and Deng, J. (2020). Raft: Recurrent all-pairs
field transforms for optical flow. arXiv preprint
arXiv:2003.12039.
Wannenwetsch, A. S. and Roth, S. (2020). Probabilistic
pixel-adaptive refinement networks. In Proceedings
of the IEEE/CVF Conference on Computer Vision and
Pattern Recognition (CVPR).
Wu, H., Zheng, S., Zhang, J., and Huang, K. (2018). Fast
end-to-end trainable guided filter. In Proceedings of
the IEEE Conference on Computer Vision and Pattern
Recognition, pages 1838–1847.
Xu, J., Ranftl, R., and Koltun, V. (2017). Accurate optical
flow via direct cost volume processing. In Proceed-
ings of the IEEE Conference on Computer Vision and
Pattern Recognition, pages 1289–1297.
Yang, G. and Ramanan, D. (2019). Volumetric correspon-
dence networks for optical flow. In Advances in neural
information processing systems, pages 794–805.
Yang, Q., Yang, R., Davis, J., and Nist
´
er, D. (2007). Spatial-
depth super resolution for range images. In 2007 IEEE
Conference on Computer Vision and Pattern Recogni-
tion, pages 1–8. IEEE.
Normalized Convolution Upsampling for Refined Optical Flow Estimation
751