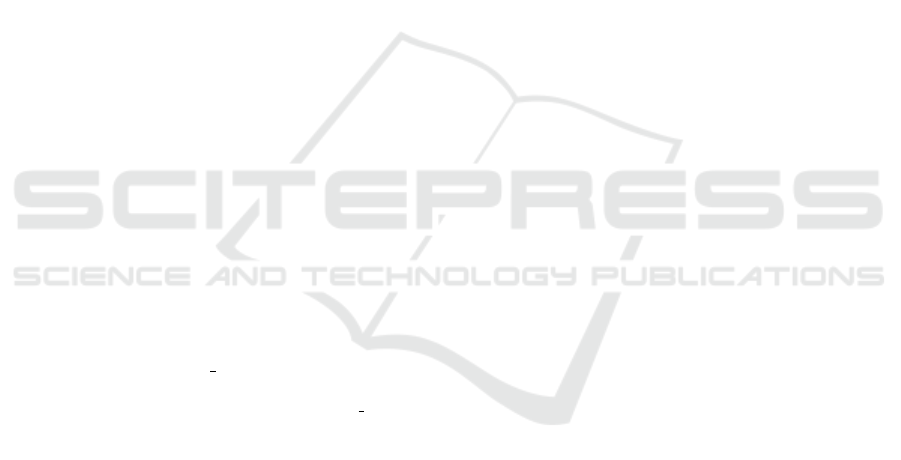
Craik, A., He, Y., and Contreras-Vidal, J. L. (2019). Deep
learning for electroencephalogram (EEG) classifica-
tion tasks:a review. Journal of Neural Engineering,
16(3):031001.
Elephant authors and contributors (2020). Ele-
phant - electrophysiology analysis toolkit.
https://elephant.readthedocs.io/en/latest/, last ac-
cessed on 2020-04-19.
Garcia, S. and Gill, J.(2019). ephyviewer 1.3.2.dev.
https://ephyviewer.readthedocs.io/en/latest/, last ac-
cessed on 2020-04-19.
Garcia, S., Guarino, D., Jaillet, F., Jennings, T., Pr
¨
opper, R.,
Rautenberg, P., Rodgers, C., Sobolev, A., Wachtler,
T., Yger, P., and Davison, A. (2014). Neo: an object
model for handling electrophysiology data in multiple
formats. Frontiers in Neuroinformatics, 8:10.
Gorgolewski, K., Auer, T., Calhoun, V., Craddock, R., Das,
S., Duff, E., et al.(2016). The brain imaging data
structure, a format for organizing and describing out-
puts of neuroimaging experiments. Scientific Data, 3.
Gramfort, A., Luessi, M., Larson, E., Engemann,
D., Strohmeier, D., Brodbeck, C., Goj, R., Jas,
M., Brooks, T., Parkkonen, L., and H
¨
am
¨
al
¨
ainen,
M.(2013). Meg and eeg data analysis with mne-
python. Frontiers in Neuroscience, 7:267.
Herger, B. (2020). keras-pandas 3.1.0. https://pypi.org/
project/keras-pandas/, last accessed on 2020-09-30.
International Neuroinformatics Coordinating Facility
(2020). https://www.incf.org/, last accessed on
2020-08-17.
Kemp, B. and Olivan, J. (2003). European data format
‘plus’ (edf+), an edf alike standard format for the ex-
change of physiological data. Clinical Neurophysiol-
ogy, 114(9):1755 – 1761.
Kothe, C. and Makeig, S. (2013). Bcilab: A platform for
brain–computer interface development. Journal of
neural engineering, 10:056014.
Kupil
´
ık, F. (2020). MNE ML - EEG data processing
pipeline using the MNE, Keras and the scikit-learn
libraries. https://github.com/fkupilik/MNE ML, last
accessed on 2020-10-27.
Mons, B., Neylon, C., Velterop, J., Dumontier, M.,
Da Silva Santos, L., and Wilkinson, M. (2017).
Cloudy, increasingly fair; revisiting the fair data guid-
ing principles for the european open science cloud. In-
formation Services and Use, 37(1):49–56.
Mou
ˇ
cek, R., Va
ˇ
reka, L., Prokop, T.,
ˇ
St
ˇ
ebet
´
ak, J., and Br
˚
uha,
P. (2017). Event-related potential data from a guess
the number brain-computer interface experiment on
school children. Scientific Data, 4 cited By 5.
Pernet, C., Appelhoff, S., Gorgolewski, K., Flandin, G.,
Phillips, C., Delorme, A., et al. (2019). Eeg-bids, an
extension to the brain imaging data structure for elec-
troencephalography. Scientific Data, 6(1).
Pr
¨
opper, R. and Obermayer, K. (2013). Spyke viewer:
a flexible and extensible platform for electrophysio-
logical data analysis. Frontiers in Neuroinformatics,
7:26.
Roy, Y., Banville, H., Albuquerque, I., Gramfort, A., Falk,
T. H., and Faubert, J. (2019). Deep learning-based
electroencephalography analysis: a systematic review.
Journal of Neural Engineering, 16(5):051001.
R
¨
ubel, O., Tritt, A., Dichter, B., Braun, T., Cain, N., Clack,
N., et al. (2019). Nwb:n 2.0: An accessible data stan-
dard for neurophysiology. bioRxiv.
Schirrmeister, R., Springenberg, J., Fiederer, L., Glasstet-
ter, M., Eggensperger, K., Tangermann, M., Hutter,
F., Burgard, W., and Ball, T. (2017). Deep learning
with convolutional neural networks for eeg decoding
and visualization: Convolutional neural networks in
eeg analysis. Human Brain Mapping, 38.
Teeters, J., Godfrey, K., Young, R., Dang, C., Friedsam, C.,
Wark, B., et al.(2015). Neurodata without borders:
Creating a common data format for neurophysiology.
Neuron, 88(4):629–634.
The Brainstorm team (2020). Brainstorm. https:// neuroim-
age.usc.edu/brainstorm/, last accessed on 2020-05-02.
The NeuralEnsemble Initiative (2020a). Pynn -
a python package for simulator-independent
specification of neuronal network models.
https://neuralensemble.org/PyNN/, last accessed
on 2020-04-19.
The NeuralEnsemble Initiative (2020b). Spykeviewer.
https://neuralensemble.org/SpykeViewer/, last ac-
cessed on 2020-05-03.
The pandas development team (2020). pandas - data analy-
sis and manipulation tool. https://pandas.pydata.org/,
last accessed on 2020-09-30.
Va
ˇ
reka, L. (2020). Evaluation of convolutional neu-
ral networks using a large multi-subject p300
dataset. Biomedical Signal Processing and Control,
58:101837.
van Gerven, M., Bahramisharif, A., Farquhar, J., and
Heskes, T. (2011). Donders machine learning tool-
box. https://github.com/distrep/DMLT, last accessed
on 2020-05-02.
Wilkinson, M., Dumontier, M., Aalbersberg, I., Appleton,
G., Axton, M., Baak, A., et al.(2016). The fair guiding
principles for scientific data management and steward-
ship. Scientific Data, 3.
Zehl, L., Jaillet, F., Stoewer, A., Grewe, J., Sobolev, A.,
Wachtler, T., et al.(2016). Handling metadata in a neu-
rophysiology laboratory. Frontiers in Neuroinformat-
ics, 10:26.
HEALTHINF 2021 - 14th International Conference on Health Informatics
676