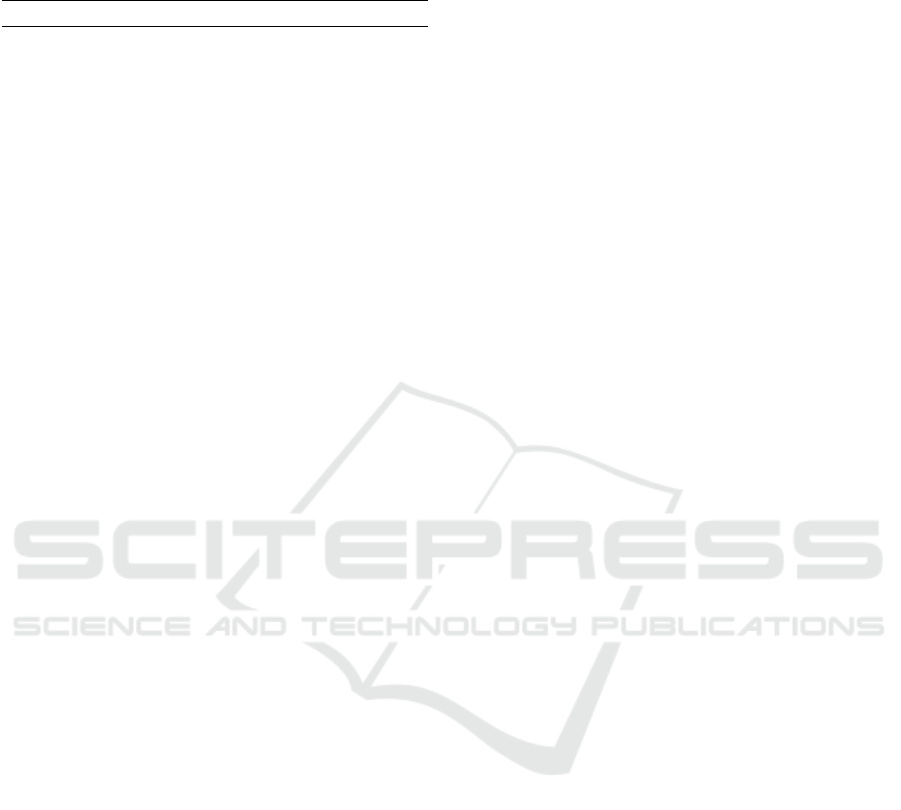
Table 3: Results on Middlebury dataset. The numbers for
the previous work are taken from (Yang et al., 2018; Engin
et al., 2018).
Method ↑PSNR ↑SSIM
DCP (He et al., 2010) 12.0234 0.6902
CycleGAN (Cai et al., 2016) 11.3037 0.3367
Cycle-Dehaze (Engin et al., 2018) 15.6016 0.8532
DDN (Yang et al., 2018) 14.9539 0.7741
DehazeNet (Cai et al., 2016) 13.5959 0.7502
MSCNN (Ren et al., 2016) 13.5501 0.7365
Ours 15.8747 0.8601
chitecture with residual blocks and skip links to re-
move haze effectively. It also leverages different loss
functions to generate realistic clean images. Using
two benchmark test datasets, we showed the effec-
tiveness of the proposed method. Our method out-
performs other methods in terms of PSNR and SSIM.
ACKNOWLEDGMENTS
We would like to thank the VISAPP’21 anonymous
reviewers for their valuable feedback. This work is
partially supported by National Science Foundation
grant IIS-1565328. Any opinions, findings, and con-
clusions or recommendations expressed in this publi-
cation are those of the authors, and do not necessarily
reflect the views of the National Science Foundation.
REFERENCES
Ancuti, C., Ancuti, C. O., De Vleeschouwer, C., and Bovik,
A. C. (2016). Night-time dehazing by fusion. In 2016
IEEE International Conference on Image Processing
(ICIP), pages 2256–2260. IEEE.
Ancuti, C. O., Ancuti, C., Hermans, C., and Bekaert, P.
(2010). A fast semi-inverse approach to detect and
remove the haze from a single image. In Asian Con-
ference on Computer Vision, pages 501–514. Springer.
Anvari, Z. and Athitsos, V. (2019). A pipeline for auto-
mated face dataset creation from unlabeled images. In
Proceedings of the 12th ACM International Confer-
ence on PErvasive Technologies Related to Assistive
Environments, pages 227–235.
Anvari, Z. and Athitsos, V. (2020). Evaluating single image
dehazing methods under realistic sunlight haze. arXiv
preprint arXiv:2008.13377.
Cai, B., Xu, X., Jia, K., Qing, C., and Tao, D. (2016). De-
hazenet: An end-to-end system for single image haze
removal. IEEE Transactions on Image Processing,
25(11):5187–5198.
Chen, J., Chen, J., Chao, H., and Yang, M. (2018). Image
blind denoising with generative adversarial network
based noise modeling. In Proceedings of the IEEE
Conference on Computer Vision and Pattern Recogni-
tion, pages 3155–3164.
Emberton, S., Chittka, L., and Cavallaro, A. (2015). Hier-
archical rank-based veiling light estimation for under-
water dehazing.
Engin, D., Genc¸, A., and Kemal Ekenel, H. (2018). Cycle-
dehaze: Enhanced cyclegan for single image dehaz-
ing. In Proceedings of the IEEE Conference on
Computer Vision and Pattern Recognition Workshops,
pages 825–833.
He, K., Sun, J., and Tang, X. (2010). Single image haze
removal using dark channel prior. IEEE transac-
tions on pattern analysis and machine intelligence,
33(12):2341–2353.
Jiang, Y., Gong, X., Liu, D., Cheng, Y., Fang, C., Shen, X.,
Yang, J., Zhou, P., and Wang, Z. (2019). Enlighten-
gan: Deep light enhancement without paired supervi-
sion. arXiv preprint arXiv:1906.06972.
Kumar, R. and Moyal, V. (2013). Visual image quality as-
sessment technique using fsim. International Journal
of Computer Applications Technology and Research,
2(3):250–254.
Kupyn, O., Budzan, V., Mykhailych, M., Mishkin, D., and
Matas, J. (2018). Deblurgan: Blind motion deblurring
using conditional adversarial networks. In Proceed-
ings of the IEEE Conference on Computer Vision and
Pattern Recognition, pages 8183–8192.
Ledig, C., Theis, L., Husz
´
ar, F., Caballero, J., Cunningham,
A., Acosta, A., Aitken, A., Tejani, A., Totz, J., Wang,
Z., et al. (2017). Photo-realistic single image super-
resolution using a generative adversarial network. In
Proceedings of the IEEE conference on computer vi-
sion and pattern recognition, pages 4681–4690.
Li, B., Peng, X., Wang, Z., Xu, J., and Feng, D. (2017).
Aod-net: All-in-one dehazing network. In Proceed-
ings of the IEEE International Conference on Com-
puter Vision, pages 4770–4778.
Lin, W.-A., Chen, J.-C., Castillo, C. D., and Chellappa,
R. (2018). Deep density clustering of unconstrained
faces. In Proceedings of the IEEE Conference
on Computer Vision and Pattern Recognition, pages
8128–8137.
Lin, W.-A., Chen, J.-C., and Chellappa, R. (2017). A
proximity-aware hierarchical clustering of faces. In
2017 12th IEEE International Conference on Auto-
matic Face & Gesture Recognition (FG 2017), pages
294–301. IEEE.
Liu, W., Anguelov, D., Erhan, D., Szegedy, C., Reed, S.,
Fu, C.-Y., and Berg, A. C. (2016). Ssd: Single shot
multibox detector. In European conference on com-
puter vision, pages 21–37. Springer.
Long, J., Shelhamer, E., and Darrell, T. (2015). Fully con-
volutional networks for semantic segmentation. In
Proceedings of the IEEE conference on computer vi-
sion and pattern recognition, pages 3431–3440.
Luo, M. R., Cui, G., and Rigg, B. (2001). The devel-
opment of the cie 2000 colour-difference formula:
Ciede2000. Color Research & Application: Endorsed
by Inter-Society Color Council, The Colour Group
Enhanced CycleGAN Dehazing Network
201