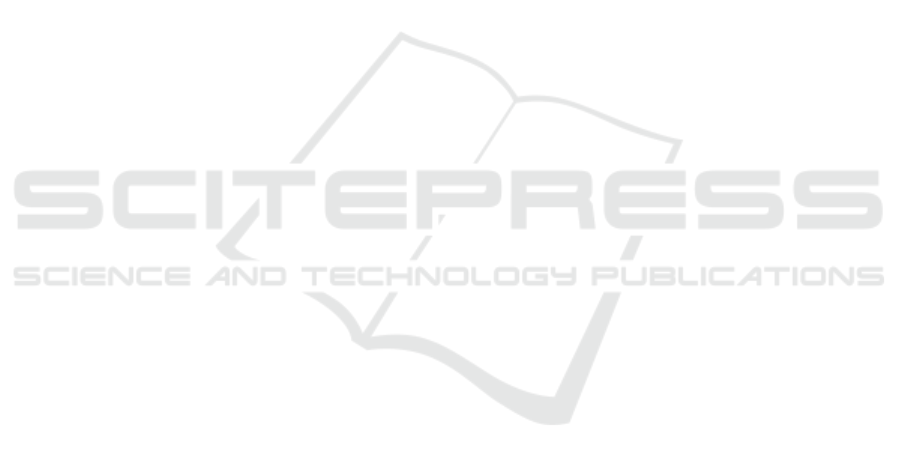
5 CONCLUSIONS AND FUTURE
WORK
In this paper we presented an extension to our pre-
vious off-road pedestrian detection dataset OPEDD,
which adds full image semantic segmentation an-
notations to 203 images. To this end we defined
19 semantic classes: grass, trees, sky, drivable and
non-drivable dirt, obstacles, crops, building, per-
son, bush, wall, drivable and non-drivable pavement,
held/carried object, truck, car, excavator, guard rail
and camper. The chosen images were selected in a
way that retains the wide range of outdoor environ-
ments and special human poses that were depicted in
OPEDD. For future work, we intend to completely se-
mantically annotate some of the video sequences the
images were taken from, to allow for the use of the
dataset in semantic SLAM and tracking tasks.
ACKNOWLEDGEMENTS
We would like to thank Ahmed Elsherif and Mitesh
Mittal for their help in annotating and reviewing the
quality of the annotations.
REFERENCES
Computer Vision Annotation Tool (2020). URL: https://
software.intel.com/content/www/us/ens/develop/
articles/computer-vision-annotation-tool-a-universal-
approach-to-data-annotation. html (visited on
12/18/2020).
Cordts, M., Omran, M., Ramos, S., Rehfeld, T., Enzweiler,
M., Benenson, R., Franke, U., Roth, S., and Schiele,
B. (2016). The Cityscapes Dataset for Semantic Urban
Scene Understanding. Proceedings of the IEEE Com-
puter Society Conference on Computer Vision and
Pattern Recognition, pages 3213–3223.
Geiger, A., Lenz, P., and Urtasun, R. (2012). Are we
ready for Autonomous Driving? The KITTI Vision
Benchmark Suite. Proceedings of the IEEE Computer
Society Conference on Computer Vision and Pattern
Recognition, pages 3354–3361.
Geyer, J., Kassahun, Y., Mahmudi, M., Ricou, X., Durgesh,
R., Chung, A. S., Hauswald, L., Pham, V. H.,
M
¨
uhlegg, M., Dorn, S., Fernandez, T., J
¨
anicke, M.,
Mirashi, S., Savani, C., Sturm, M., Vorobiov, O.,
Oelker, M., Garreis, S., and Schuberth, P. (2020).
A2D2: Audi Autonomous Driving Dataset.
Halevy, A., Norvig, P., and Pereira, F. (2009). The un-
reasonable effectiveness of data. Intelligent Systems.
IEEE.
Huang, X., Wang, P., Cheng, X., Zhou, D., Geng, Q., and
Yang, R. (2020). The ApolloScape Open Dataset
for Autonomous Driving and Its Application. IEEE
Transactions on Pattern Analysis and Machine Intel-
ligence, 42(10):2702–2719.
Neigel, P., Ameli, M., Katrolia, J., Feld, H., Wasenm
¨
uller,
O., and Stricker, D. (2020). OPEDD: Off-road pedes-
trian detection dataset. Journal of WSCG, 28(1-
2):207–212.
Neuhold, G., Ollmann, T., Bulo, S. R., and Kontschieder,
P. (2017). The Mapillary Vistas Dataset for Semantic
Understanding of Street Scenes. Proceedings of the
IEEE International Conference on Computer Vision,
2017-October:5000–5009.
Pezzementi, Z., Tabor, T., Hu, P., Chang, J. K., Ramanan,
D., Wellington, C., Wisely Babu, B. P., and Herman,
H. (2018). Comparing apples and oranges: Off-road
pedestrian detection on the National Robotics Engi-
neering Center agricultural person-detection dataset.
Journal of Field Robotics, 35(4):545–563.
Tabor, T., Pezzementi, Z., Vallespi, C., and Wellington, C.
(2015). People in the weeds: Pedestrian detection
goes off-road. In 2015 IEEE International Sympo-
sium on Safety, Security, and Rescue Robotics (SSRR),
pages 1–7.
Xiang, Y., Wang, H., Su, T., Li, R., and Geimer, M. (2020).
KIT MOMA: A Mobile Machines Dataset. ArXiv
Preprint.
Yu, F., Chen, H., Wang, X., Xian, W., Chen, Y., Liu, F.,
Madhavan, V., and Darrell, T. (2020). BDD100K: A
Diverse Driving Dataset for Heterogeneous Multitask
Learning.
ZED (2020). URL: https://www.stereolabs.com/zed/ (vis-
ited on 12/18/2020).
Zhu, X., Vondrick, C., Fowlkes, C. C., and Ramanan, D.
(2015). Do We Need More Training Data? Interna-
tional Journal of Computer Vision (IJCV), pages 1–
17.
OFFSED: Off-Road Semantic Segmentation Dataset
557