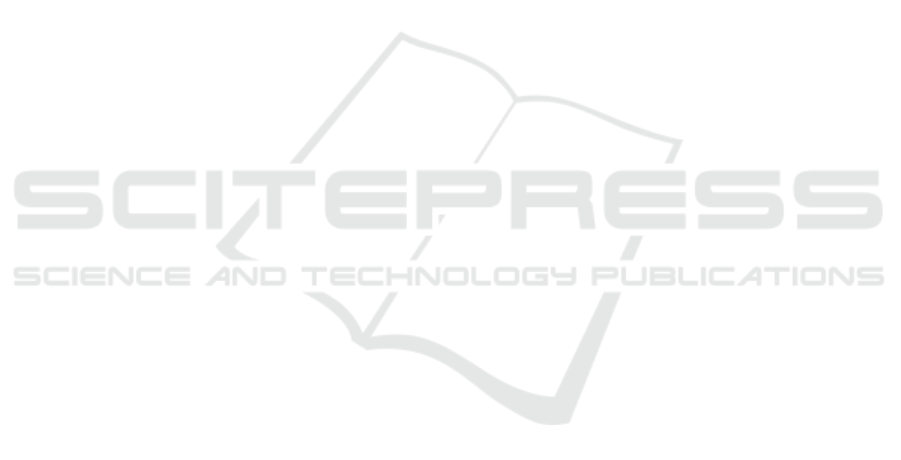
Barros, P., Parisi, G. I., Jirak, D., and Wermter, S. (2014).
Real-time gesture recognition using a humanoid robot
with a deep neural architecture. In 2014 IEEE-RAS
International Conference on Humanoid Robots, pages
646–651. IEEE.
Barros, P. and Wermter, S. (2016). Developing crossmodal
expression recognition based on a deep neural model.
Adaptive behavior, 24(5):373–396.
Breazeal, C. (2003). Emotion and sociable humanoid
robots. International journal of human-computer
studies, 59(1-2):119–155.
Chen, S., Tian, Y., Liu, Q., and Metaxas, D. N. (2013). Rec-
ognizing expressions from face and body gesture by
temporal normalized motion and appearance features.
Image and Vision Computing, 31(2):175–185.
Chollet, F. (2016). Xception: deep learning with depthwise
separable convolutions. corr abs/1610.02357 (2016).
arXiv preprint arXiv:1610.02357.
de Gelder, B., De Borst, A., and Watson, R. (2015). The
perception of emotion in body expressions. Wiley In-
terdisciplinary Reviews: Cognitive Science, 6(2):149–
158.
Ekman, P., Friesen, W. V., and Ellsworth, P. (2013). Emo-
tion in the human face: Guidelines for research and
an integration of findings, volume 11. Elsevier.
Fukui, A., Park, D. H., Yang, D., Rohrbach, A., Darrell, T.,
and Rohrbach, M. (2016). Multimodal compact bilin-
ear pooling for visual question answering and visual
grounding. arXiv preprint arXiv:1606.01847.
Glowinski, D., Dael, N., Camurri, A., Volpe, G., Mortillaro,
M., and Scherer, K. (2011). Toward a minimal repre-
sentation of affective gestures. IEEE Transactions on
Affective Computing, 2(2):106–118.
Gunes, H. and Piccardi, M. (2006). A bimodal face and
body gesture database for automatic analysis of hu-
man nonverbal affective behavior. In 18th Interna-
tional Conference on Pattern Recognition (ICPR’06),
volume 1, pages 1148–1153. IEEE.
Gunes, H. and Piccardi, M. (2007). Bi-modal emo-
tion recognition from expressive face and body ges-
tures. Journal of Network and Computer Applications,
30(4):1334–1345.
Gunes, H. and Piccardi, M. (2008). Automatic tempo-
ral segment detection and affect recognition from
face and body display. IEEE Transactions on Sys-
tems, Man, and Cybernetics, Part B (Cybernetics),
39(1):64–84.
Ilyas, C. M. A., Haque, M. A., Rehm, M., Nasrollahi, K.,
and Moeslund, T. B. (2018a). Facial expression recog-
nition for traumatic brain injured patients. In VISI-
GRAPP (4: VISAPP), pages 522–530.
Ilyas, C. M. A., Nasrollahi, K., Rehm, M., and Moes-
lund, T. B. (2018b). Rehabilitation of traumatic brain
injured patients: Patient mood analysis from multi-
modal video. In 2018 25th IEEE International Confer-
ence on Image Processing (ICIP), pages 2291–2295.
IEEE.
Jerritta, S., Murugappan, M., Nagarajan, R., and Wan, K.
(2011). Physiological signals based human emotion
recognition: a review. In 2011 IEEE 7th Interna-
tional Colloquium on Signal Processing and its Ap-
plications, pages 410–415. IEEE.
Karpouzis, K., Caridakis, G., Kessous, L., Amir, N.,
Raouzaiou, A., Malatesta, L., and Kollias, S. (2007).
Modeling naturalistic affective states via facial, vocal,
and bodily expressions recognition. In Artifical intelli-
gence for human computing, pages 91–112. Springer.
Khorrami, P., Le Paine, T., Brady, K., Dagli, C., and Huang,
T. S. (2016). How deep neural networks can improve
emotion recognition on video data. In 2016 IEEE in-
ternational conference on image processing (ICIP),
pages 619–623. IEEE.
Kim, J. and Andr
´
e, E. (2008). Emotion recognition based on
physiological changes in music listening. IEEE trans-
actions on pattern analysis and machine intelligence,
30(12):2067–2083.
Kim, K., Cha, Y.-S., Park, J.-M., Lee, J.-Y., and You, B.-J.
(2011). Providing services using network-based hu-
manoids in a home environment. IEEE Transactions
on Consumer Electronics, 57(4):1628–1636.
Kingma, D. P. and Ba, J. (2014). Adam: A
method for stochastic optimization. arXiv preprint
arXiv:1412.6980.
Lang, K., Dapelo, M. M., Khondoker, M., Morris, R., Sur-
guladze, S., Treasure, J., and Tchanturia, K. (2015).
Exploring emotion recognition in adults and ado-
lescents with anorexia nervosa using a body mo-
tion paradigm. European Eating Disorders Review,
23(4):262–268.
Lin, T.-Y., RoyChowdhury, A., and Maji, S. (2017). Bilin-
ear cnns for fine-grained visual recognition. In Trans-
actions of Pattern Analysis and Machine Intelligence
(PAMI).
Mano, L. Y., Faic¸al, B. S., Nakamura, L. H., Gomes, P. H.,
Libralon, G. L., Meneguete, R. I., Geraldo Filho, P.,
Giancristofaro, G. T., Pessin, G., Krishnamachari, B.,
et al. (2016). Exploiting iot technologies for enhanc-
ing health smart homes through patient identification
and emotion recognition. Computer Communications,
89:178–190.
Mart
´
ınez-Rodrigo, A., Zangr
´
oniz, R., Pastor, J. M., Latorre,
J. M., and Fern
´
andez-Caballero, A. (2015). Emo-
tion detection in ageing adults from physiological sen-
sors. In Ambient Intelligence-Software and Applica-
tions, pages 253–261. Springer.
Mehrabian, A. et al. (1971). Silent messages, volume 8.
Wadsworth Belmont, CA.
Nguyen, D., Nguyen, K., Sridharan, S., Dean, D., and
Fookes, C. (2018). Deep spatio-temporal feature fu-
sion with compact bilinear pooling for multimodal
emotion recognition. Computer Vision and Image Un-
derstanding, 174:33–42.
Noroozi, F., Kaminska, D., Corneanu, C., Sapinski, T., Es-
calera, S., and Anbarjafari, G. (2018). Survey on emo-
tional body gesture recognition. IEEE transactions on
affective computing.
Piana, S., Stagliano, A., Odone, F., Verri, A., and Camurri,
A. (2014). Real-time automatic emotion recognition
from body gestures. arXiv preprint arXiv:1402.5047.
Picard, R. W., Vyzas, E., and Healey, J. (2001). Toward
machine emotional intelligence: Analysis of affective
VISAPP 2021 - 16th International Conference on Computer Vision Theory and Applications
678