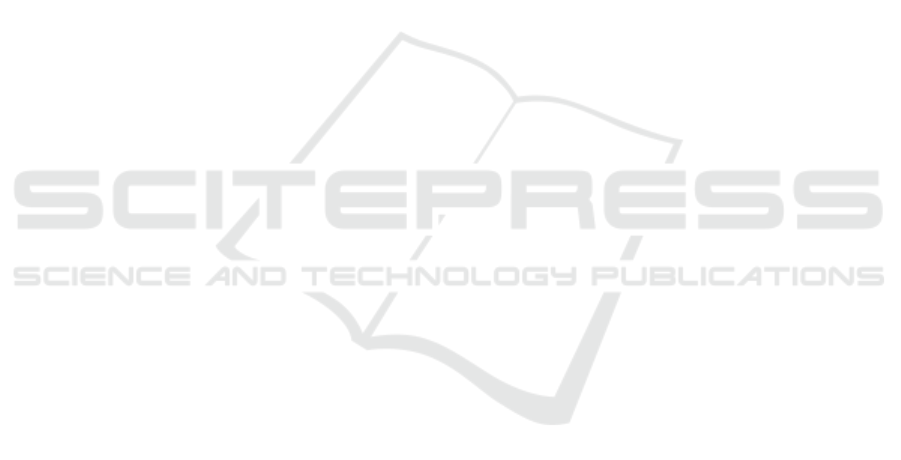
8 CONCLUSIONS
This study presents a computationally efficient
method to estimate the facial feature points as well
as several additional attributes (i.e., head orientation,
facial gestures and eye gaze orientation) in a single
computation.
The experimental section shows that even if meth-
ods like (Baltru
ˇ
saitis et al., 2018) have more accu-
rate landmark estimation and real-time performance,
our method uses significantly fewer resources (∼81%
faster) with a less significant accuracy loss (∼23%).
Considering hardware with computational con-
straints, and the results of the experiments as a ref-
erence, the proposed method maintains real-time per-
formance even on smartphones with ARM architec-
ture like the IphoneSE. Thus, it allows the integration
of real-time face tracking and analysis systems into
several types of constrained devices.
REFERENCES
Aneja, D., Colburn, A., Faigin, G., Shapiro, L., and Mones,
B. (2017). Modeling Stylized Character Expressions
via Deep Learning. In Lai, S.-H., Lepetit, V., Nishino,
K., and Sato, Y., editors, Computer Vision ACCV
2016, volume 1, pages 136—-153. Springer Interna-
tional Publishing.
Baltru
ˇ
saitis, T., Mahmoud, M., and Robinson, P. (2015).
Cross-dataset learning and person-specific normalisa-
tion for automatic Action Unit detection. In Interna-
tional Conference and Workshops on Automatic Face
and Gesture Recognition (FG), pages 1–6. IEEE.
Baltru
ˇ
saitis, T., Zadeh, A., Lim, Y. C., and Morency,
L. P. (2018). OpenFace 2.0: Facial behavior analy-
sis toolkit. In Proceedings - 13th IEEE International
Conference on Automatic Face and Gesture Recogni-
tion, FG 2018. IEEE.
Cao, C., Wu, H., Weng, Y., Shao, T., and Zhou, K. (2016).
Real-time facial animation with image-based dynamic
avatars. ACM Transactions on Graphics, 35(4):1–12.
Ekman, P., Friesen, W. V., and Hager, H. (2002). Facial
action coding system. Investigator’s Guide. Salt Lake
City.
Jain, N., Kumar, S., Kumar, A., Shamsolmoali, P., and
Zareapoor, M. (2018). Hybrid deep neural networks
for face emotion recognition. Pattern Recognition Let-
ters, 115:101 – 106. Multimodal Fusion for Pattern
Recognition.
Jin, X. and Tan, X. (2017). Face alignment in-the-wild: A
Survey. Computer Vision and Image Understanding,
162:1–22.
Kazemi, V. and Sullivan, J. (2014). One Millisecond Face
Alignment with an Ensemble of Regression Trees.
Computer Vision and Pattern Recognition (CVPR).
Li, W., Abtahi, F., and Zhu, Z. (2017). Action unit de-
tection with region adaptation, multi-labeling learning
and optimal temporal fusing. Proceedings - 30th IEEE
Conference on Computer Vision and Pattern Recogni-
tion, CVPR 2017, 2017-Janua:6766–6775.
Pons, G. and Masip, D. (2018). Multi-task, multi-label
and multi-domain learning with residual convolu-
tional networks for emotion recognition. CoRR.
Ranjan, A., Bolkart, T., Sanyal, S., and Black, M. J. (2018).
Generating 3d faces using convolutional mesh autoen-
coders. In Ferrari, V., Hebert, M., Sminchisescu, C.,
and Weiss, Y., editors, European Conference on Com-
puter Vision (ECCV), pages 725–741, Cham. Springer
International Publishing.
Rathee, N. and Ganotra, D. (2018). An efficient approach
for facial action unit intensity detection using distance
metric learning based on cosine similarity. Signal, Im-
age and Video Processing, 12(6):1141–1148.
Ren, S., Cao, X., Wei, Y., and Sun, J. (2014). Face align-
ment at 3000 FPS via regressing local binary features.
Proceedings of the IEEE Computer Society Confer-
ence on Computer Vision and Pattern Recognition,
1(1):1685–1692.
Sagonas, C., Antonakos, E., Tzimiropoulos, G., Zafeiriou,
S., and Pantic, M. (2016). 300 faces In-the-wild chal-
lenge: Database and results. Image and Vision Com-
puting, 47:3–18.
Sanchez-Lozano, E., Tzimiropoulos, G., and Valstar, M.
(2018). Joint Action Unit localisation and intensity es-
timation through heatmap regression. In BMVC, pages
1–12.
Saragih, J. M., Lucey, S., and Cohn, J. F. (2009). Face
alignment through subspace constrained mean-shifts.
In IEEE 12th International Conference on Computer
Vision, pages 1034–1041.
Shao, Z., Liu, Z., Cai, J., and Ma, L. (2018). Deep Adaptive
Attention for Joint Facial Action Unit Detection and
Face Alignment. ArXiv.
Wood, E., Baltru
ˇ
saitis, T., Morency, L.-P., Robinson, P., and
Bulling, A. (2016). Learning an appearance-based
gaze estimator from one million synthesised images.
In Proceedings of the Ninth Biennial ACM Symposium
on Eye Tracking Research & Applications, pages 131–
138.
Wu, Y. and Ji, Q. (2018). Facial Landmark Detection: a
Literature Survey. International Journal of Computer
Vision.
Zadeh, A., Baltru
ˇ
saitis, T., and Morency, L. P. (2017). Con-
volutional Experts Constrained Local Model for Fa-
cial Landmark Detection. In IEEE Computer Society
Conference on Computer Vision and Pattern Recogni-
tion Workshops, volume 2017-July, pages 2051–2059.
IEEE.
Zhang, Z., Luo, P., Loy, C. C., and Tang, X. (2014). Fa-
cial landmark detection by deep multi-task learning.
In European Conference on Computer Vision (ECCV),
pages 94–108. Springer International Publishing.
Efficient Multi-task based Facial Landmark and Gesture Detection in Monocular Images
687