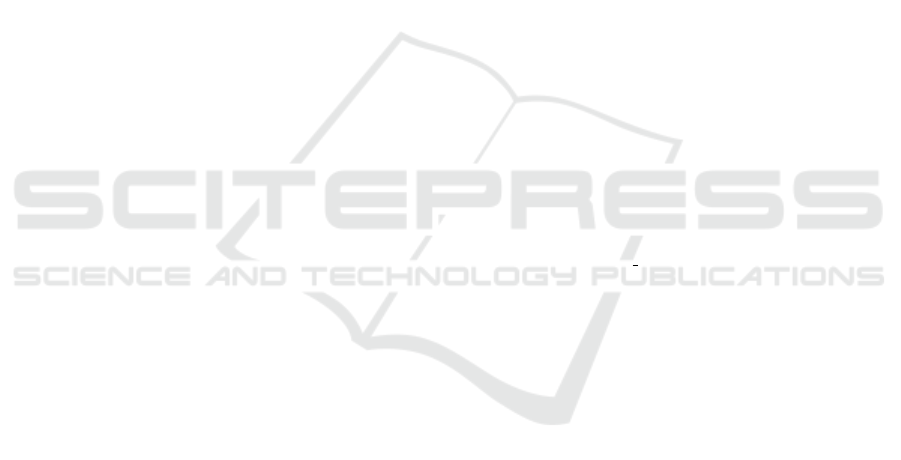
Delogu, F., Kunath, B., Evans, P., Arntzen, M., Hvid-
sten, T., and Pope, P. (2020). Integration of abso-
lute multi-omics reveals dynamic protein-to-rna ratios
and metabolic interplay within mixed-domain micro-
biomes. Nature Communications, 11(1):1–12.
Dinu, I., Potter, J. D., Mueller, T., Liu, Q., Adewale, A. J.,
Jhangri, G. S., Einecke, G., Famulski, K. S., Halloran,
P., and Yasui, Y. (2007). Improving gene set analysis
of microarray data by sam-gs. BMC bioinformatics,
8(1):242.
Doshi-Velez, F. and Kim, B. (2017). Towards a rigorous sci-
ence of interpretable machine learning. arXiv preprint
arXiv:1702.08608.
Drouin, A., Gigu
`
ere, S., D
´
eraspe, M., Marchand, M., Tyers,
M., Loo, V. G., Bourgault, A.-M., Laviolette, F., and
Corbeil, J. (2016). Predictive computational pheno-
typing and biomarker discovery using reference-free
genome comparisons. BMC genomics, 17(1):1–15.
Freedman, M. L., Monteiro, A. N., Gayther, S. A., Coet-
zee, G. A., Risch, A., Plass, C., Casey, G., De Biasi,
M., Carlson, C., Duggan, D., et al. (2011). Principles
for the post-gwas functional characterization of cancer
risk loci. Nature genetics, 43(6):513–518.
G
¨
unther, O. P., Chen, V., Freue, G. C., Balshaw, R. F.,
Tebbutt, S. J., Hollander, Z., Takhar, M., McMaster,
W. R., McManus, B. M., Keown, P. A., et al. (2012). A
computational pipeline for the development of multi-
marker bio-signature panels and ensemble classifiers.
BMC bioinformatics, 13(1):326.
Heuschkel, M. A., Skenteris, N. T., Hutcheson, J. D.,
van der Valk, D. D., Bremer, J., Goody, P., Hjortnaes,
J., Jansen, F., Bouten, C. V., van den Bogaerdt, A.,
et al. (2020). Integrative multi-omics analysis in cal-
cific aortic valve disease reveals a link to the formation
of amyloid-like deposits. Cells, 9(10):2164.
Iorio, M. V., Ferracin, M., Liu, C.-G., Veronese, A., Spizzo,
R., Sabbioni, S., Magri, E., Pedriali, M., Fabbri, M.,
Campiglio, M., et al. (2005). Microrna gene expres-
sion deregulation in human breast cancer. Cancer re-
search, 65(16):7065–7070.
Karczewski, K. J. and Snyder, M. P. (2018). Integrative
omics for health and disease. Nature Reviews Genet-
ics, 19(5):299.
Komatsu, S., Ichikawa, D., Takeshita, H., Morimura, R.,
Hirajima, S., Tsujiura, M., Kawaguchi, T., Miyamae,
M., Nagata, H., Konishi, H., et al. (2014). Circulat-
ing mir-18a: a sensitive cancer screening biomarker
in human cancer. in vivo, 28(3):293–297.
Lao, V. V. and Grady, W. M. (2011). Epigenetics and col-
orectal cancer. Nature reviews Gastroenterology &
hepatology, 8(12):686.
Lehmann, B. D., Bauer, J. A., Chen, X., Sanders, M. E.,
Chakravarthy, A. B., Shyr, Y., and Pietenpol, J. A.
(2011). Identification of human triple-negative breast
cancer subtypes and preclinical models for selection
of targeted therapies. The Journal of clinical investi-
gation, 121(7):2750–2767.
Li, X., Wu, Y., Liu, A., and Tang, X. (2016). Long
non-coding rna uca1 enhances tamoxifen resistance
in breast cancer cells through a mir-18a-hif1α feed-
back regulatory loop. Tumor Biology, 37(11):14733–
14743.
Liberzon, A., Subramanian, A., Pinchback, R., Thor-
valdsd
´
ottir, H., Tamayo, P., and Mesirov, J. P. (2011).
Molecular signatures database (msigdb) 3.0. Bioinfor-
matics, 27(12):1739–1740.
Liu, Y., Devescovi, V., Chen, S., and Nardini, C. (2013).
Multilevel omic data integration in cancer cell lines:
advanced annotation and emergent properties. BMC
systems biology, 7(1):14.
Marchand, M. and Shawe-Taylor, J. (2002). The set cover-
ing machine. Journal of Machine Learning Research,
3(Dec):723–746.
Miller, T. (2019). Explanation in artificial intelligence: In-
sights from the social sciences. Artificial Intelligence,
267:1–38.
Molnar, C. (2019). Interpretable Machine Learning. https:
//christophm.github.io/interpretable-ml-book/.
Rappoport, N., Safra, R., and Shamir, R. (2020). Monet:
Multi-omic module discovery by omic selection.
PLOS Computational Biology, 16(9):e1008182.
Ritchie, M. D., Holzinger, E. R., Li, R., Pendergrass, S. A.,
and Kim, D. (2015). Methods of integrating data to
uncover genotype-phenotype interactions. Nature re-
views. Genetics, 16(2):85.
Singh, A., Gautier, B., Shannon, C. P., Vacher, M., Rohart,
F., Tebutt, S. J., and Le Cao, K.-A. (2016). Diablo-
an integrative, multi-omics, multivariate method for
multi-group classification. bioRxiv, page 067611.
Stark, C., Breitkreutz, B.-J., Reguly, T., Boucher, L., Bre-
itkreutz, A., and Tyers, M. (2006). Biogrid: a general
repository for interaction datasets. Nucleic acids re-
search, 34(suppl 1):D535–D539.
Tian, S., Wang, C., and Wang, B. (2019). Incorporating
pathway information into feature selection towards
better performed gene signatures. BioMed research
international, 2019.
Weigelt, B., Baehner, F. L., and Reis-Filho, J. S. (2010).
The contribution of gene expression profiling to breast
cancer classification, prognostication and prediction:
a retrospective of the last decade. The Journal of
Pathology: A Journal of the Pathological Society of
Great Britain and Ireland, 220(2):263–280.
Weizmann, I. o. S. (2020a). Mir18a. https://www.
genecards.org/cgi-bin/carddisp.pl?gene=MIR18A.
Accessed: 2020-10-21.
Weizmann, I. o. S. (2020b). Mir190b. https://www.
genecards.org/cgi-bin/carddisp.pl?gene=MIR190B.
Accessed: 2020-10-21.
Wu, D., Lim, E., Vaillant, F., Asselin-Labat, M.-L., Vis-
vader, J. E., and Smyth, G. K. (2010). Roast: ro-
tation gene set tests for complex microarray experi-
ments. Bioinformatics, 26(17):2176–2182.
Yuan, Y., Liu, L., Chen, H., Wang, Y., Xu, Y., Mao, H., Li,
J., Mills, G. B., Shu, Y., Li, L., et al. (2016). Com-
prehensive characterization of molecular differences
in cancer between male and female patients. Cancer
cell, 29(5):711–722.
Zhang, N., Zhang, H., Liu, Y., Su, P., Zhang, J., Wang, X.,
Sun, M., Chen, B., Zhao, W., Wang, L., et al. (2019).
Applying PySCMGroup to Breast Cancer Biomarkers Discovery
81