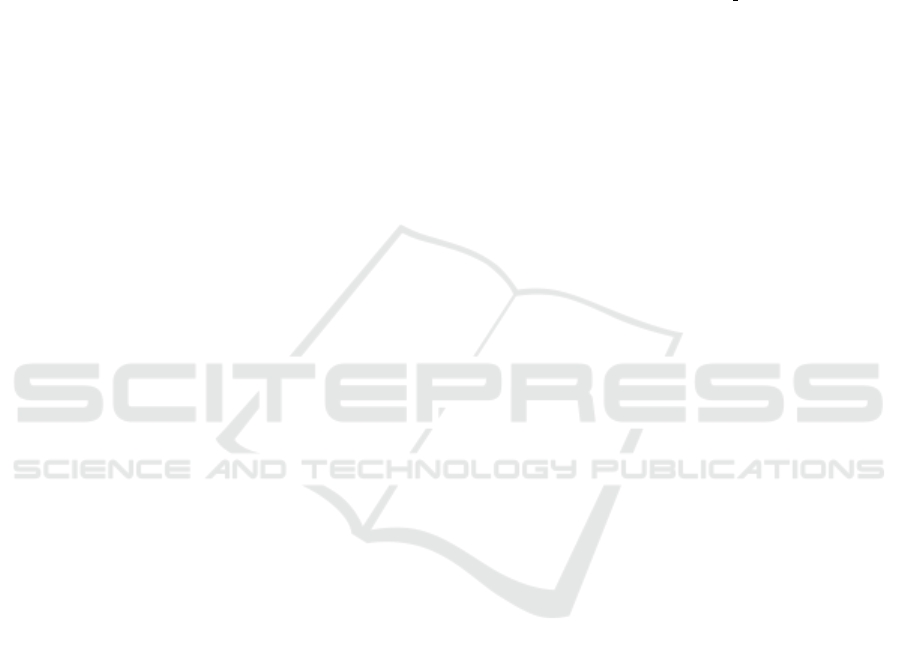
ACKNOWLEDGEMENTS
This research has been co-financed by the European
Union and Greek national funds through the Oper-
ational Program Competitiveness, Entrepreneurship
and Innovation, under the call RESEARCH – CRE-
ATE – INNOVATE (project code: BeHEALTHIER -
T2EDK-04207).
REFERENCES
Bair, E., Hastie, T., Paul, D., and Tibshirani, R.
(2006). Prediction by supervised principal compo-
nents. Journal of the American Statistical Association,
101(473):119–137.
Bair, E. and Tibshirani, R. (2004). Semi-supervised meth-
ods to predict patient survival from gene expression
data. PLoS Biol, 2(4):e108.
Bou-Hamad, I., Larocque, D., Ben-Ameur, H., et al. (2011).
A review of survival trees. Statistics surveys, 5:44–71.
Chapfuwa, P., Li, C., Mehta, N., Carin, L., and Henao, R.
(2020). Survival cluster analysis. In Proceedings of
the ACM Conference on Health, Inference, and Learn-
ing, pages 60–68.
Ciampi, A., Chang, C.-H., Hogg, S., and McKinney, S.
(1987). Recursive partition: A versatile method for
exploratory-data analysis in biostatistics. In Biostatis-
tics, pages 23–50. Springer.
Clark, T. G., Bradburn, M. J., Love, S. B., and Altman,
D. G. (2003). Survival analysis part i: basic con-
cepts and first analyses. British journal of cancer,
89(2):232–238.
Cox, D. R. (1972). Regression models and life-tables. Jour-
nal of the Royal Statistical Society: Series B (Method-
ological), 34(2):187–202.
Dunn, O. J. and Clark, V. A. (2009). Basic statistics: a
primer for the biomedical sciences. John Wiley &
Sons.
Gaynor, S. and Bair, E. (2017). Identification of relevant
subtypes via preweighted sparse clustering. Compu-
tational statistics & data analysis, 116:139–154.
Giunchiglia, E., Nemchenko, A., and van der Schaar, M.
(2018). Rnn-surv: A deep recurrent model for sur-
vival analysis. In International Conference on Artifi-
cial Neural Networks, pages 23–32. Springer.
Ibrahim, J. G., Chen, M.-H., and Sinha, D. (2014). B
ayesian survival analysis. Wiley StatsRef: Statistics
Reference Online.
Kaplan, E. L. and Meier, P. (1958). Nonparametric esti-
mation from incomplete observations. Journal of the
American statistical association, 53(282):457–481.
Katzman, J. L., Shaham, U., Cloninger, A., Bates, J., Jiang,
T., and Kluger, Y. (2018). Deepsurv: personalized
treatment recommender system using a cox propor-
tional hazards deep neural network. BMC medical re-
search methodology, 18(1):24.
Kononenko, I. (1993). Inductive and bayesian learning in
medical diagnosis. Applied Artificial Intelligence an
International Journal, 7(4):317–337.
Kuiper, N. H. (1960). Tests concerning random points on a
circle. In Nederl. Akad. Wetensch. Proc. Ser. A, num-
ber 1 in 63, pages 38–47.
Lee, E. T. and Wang, J. (2003). Statistical methods for sur-
vival data analysis, volume 476. John Wiley & Sons.
Li, H. and Gui, J. (2004). Partial cox regression analy-
sis for high-dimensional microarray gene expression
data. Bioinformatics, 20(suppl 1):i208–i215.
Liverani, S., Leigh, L., Hudson, I. L., and Byles, J. E.
(2020). Clustering method for censored and collinear
survival data. Computational Statistics, pages 1–26.
Molitor, J., Papathomas, M., Jerrett, M., and Richardson, S.
(2010). Bayesian profile regression with an applica-
tion to the national survey of children’s health. Bio-
statistics, 11(3):484–498.
Molitor, J., Su, J. G., Molitor, N.-T., Rubio, V. G., Richard-
son, S., Hastie, D., Morello-Frosch, R., and Jerrett, M.
(2011). Identifying vulnerable populations through an
examination of the association between multipollutant
profiles and poverty. Environmental Science & Tech-
nology, 45(18):7754–7760.
Mouli, S. C., Naik, A., Ribeiro, B., and Neville, J.
(2017). Identifying user survival types via cluster-
ing of censored social network data. arXiv preprint
arXiv:1703.03401.
Mouli, S. C., Teixeira, L., Ribeiro, B., and Neville, J.
(2019). Deep lifetime clustering. arXiv preprint
arXiv:1910.00547.
Nowak, G. and Tibshirani, R. (2008). Complementary hier-
archical clustering. Biostatistics, 9(3):467–483.
Prinja, S., Gupta, N., and Verma, R. (2010). Censoring in
clinical trials: review of survival analysis techniques.
Indian journal of community medicine: official pub-
lication of Indian Association of Preventive & Social
Medicine, 35(2):217.
Rupert Jr, G. et al. (2012). Simultaneous statistical infer-
ence. Springer Science & Business Media.
Shivaswamy, P. K., Chu, W., and Jansche, M. (2007). A
support vector approach to censored targets. In Sev-
enth IEEE International Conference on Data Mining
(ICDM 2007), pages 655–660. IEEE.
Van Belle, V., Pelckmans, K., Suykens, J., and Van Huffel,
S. (2007). Support vector machines for survival analy-
sis. In Proceedings of the Third International Confer-
ence on Computational Intelligence in Medicine and
Healthcare (CIMED2007), pages 1–8.
Van Dongen, S. (2001). Graph clustering by flow simula-
tion. 2000. University of Utrecht.
Zhang, W., Tang, J., and Wang, N. (2016). Using the
machine learning approach to predict patient sur-
vival from high-dimensional survival data. In 2016
IEEE International Conference on Bioinformatics and
Biomedicine (BIBM), pages 1234–1238. IEEE.
A Survey of Survival Analysis Techniques
723