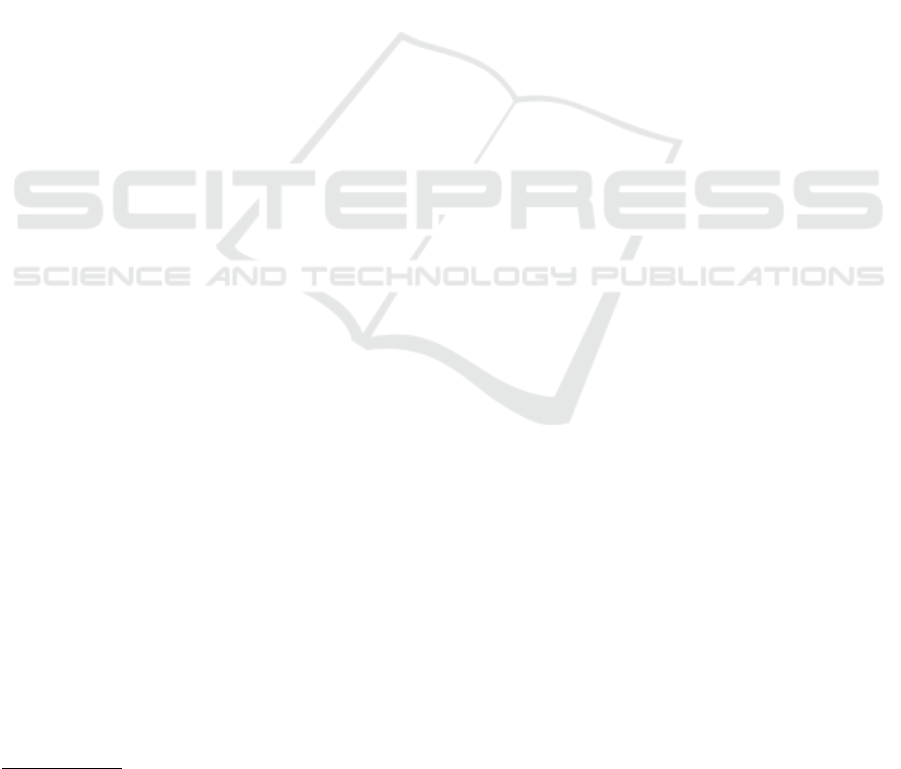
ticipants. For example, as shown in Figure 5, P1 and
P2 completed the test scenario with less than ten col-
lisions. With the current setup, it was not possible to
determine whether their performance was due to the
information shown on the HMI or they had some level
of expertise in using this kind of simulation. How-
ever, we were unable to incorporate this feature in
this study, since it was tricky to define the criteria of
collision avoidance. For example, since there were
multiple objects on both left and right sides along the
passage (see Figure 2), it was possible to avoid one
object, but accidentally hitting another object.
We believe that the proposed method is also ap-
plicable for other studies given that the following re-
quirements are fulfilled. Firstly, due to its nature, our
method is only suitable for studies within simulated
environments, where it is possible to fully record and
observe the event of interest. Although in this study
we used a mixed reality simulation, our method can
also be applied in a virtual reality simulation, given
that the headset being used has a built-in eye tracker,
such as Vive Pro Eye
5
. Secondly, here we used the oc-
currence of collisions as the trigger and the synchro-
nization point for both sources of data. Therefore, it is
best to assume that the proposed method would be ap-
plicable in studies where participants are expected to
make some errors. Thirdly, in order to use the method
as what we proposed here, there should be a support-
ive visualization system as part of the experimental
setup. Although the method could still be used with-
out a supportive visualization system as part of the
experimental setup, the collision classification would
be limited to whether the colliding object is visible
from the participant’s perspective.
8 CONCLUSION
In this study, we have classified the occurring col-
lisions based on the data from the eye tracker and
the automatic logging mechanism in the simulation.
The classification was made based on two visual per-
ception conditions: (1) the visibility of the collid-
ing objects from the participants’ perspective and
(2) whether the participants saw the information pre-
sented on the HMI before the collisions occurred.
This approach enabled us to interpret the occurring
collisions differently, compared to the traditional ap-
proach that directly interprets the total number of col-
lisions as the representation of participants’ perfor-
mance. As demonstrated in this study, the collisions
5
https://www.vive.com/eu/product/vive-pro-
eye/overview/
could occur due to different conditions. By under-
standing the underlying conditions behind the colli-
sions, designers and researchers could be more reflec-
tive when interpreting the collected data.
ACKNOWLEDGEMENTS
This research has received funding from CrossCon-
trol AB, the Swedish Knowledge Foundation (KK-
stiftelsen) through the ITS-EASY program, and the
European Union’s Horizon 2020 research and inno-
vation programme under the Marie SkłodowskaCurie
grant agreement number 764951.
REFERENCES
Albers, D., Radlmayr, J., Loew, A., Hergeth, S., Naujoks,
F., Keinath, A., and Bengler, K. (2020). Usability
evaluation—advances in experimental design in the
context of automated driving human–machine inter-
faces. Information, 11(5):240:1–240:15.
Beck, F., Blascheck, T., Ertl, T., and Weiskopf, D. (2015).
Exploring word-sized graphics for visualizing eye
tracking data within transcribed experiment record-
ings. In Proceedings of the First Workshop on
Eye Tracking and Visualization, pages 1–5, Chicago,
USA. ETVIS.
Blascheck, T., John, M., Koch, S., Bruder, L., and Ertl, T.
(2016). Triangulating user behavior using eye move-
ment, interaction, and think aloud data. In Proceed-
ings of the Ninth Biennial ACM Symposium on Eye
Tracking Research & Applications, ETRA ’16, pages
175–182, New York, USA. ACM.
Brehmer, M. and Munzner, T. (2013). A multi-level ty-
pology of abstract visualization tasks. IEEE Trans-
actions on Visualization and Computer Graphics,
19(12):2376–2385.
Cacciabue, P. C. (2004). Guide to Applying Human Factors
Methods: Human Error and Accident Management in
Safety-critical Systems. Springer, London, UK.
Crowe, E. C. and Narayanan, N. H. (2000). Comparing
interfaces based on what users watch and do. In Pro-
ceedings of the 2000 Symposium on Eye Tracking Re-
search & Applications, ETRA ’00, page 29–36, New
York, USA. ACM.
Dey, A., Billinghurst, M., Lindeman, R. W., and Swan, J. E.
(2018). A systematic review of 10 years of augmented
reality usability studies: 2005 to 2014. Frontiers in
Robotics and AI, 5:37.
Ebling, M. R. and John, B. E. (2000). On the contributions
of different empirical data in usability testing. In Pro-
ceedings of the 3rd Conference on Designing Inter-
active Systems: Processes, Practices, Methods, and
Techniques, DIS ’00, page 289–296, New York, NY,
USA. ACM.
Classifying Excavator Collisions based on Users’ Visual Perception in the Mixed Reality Environment
261