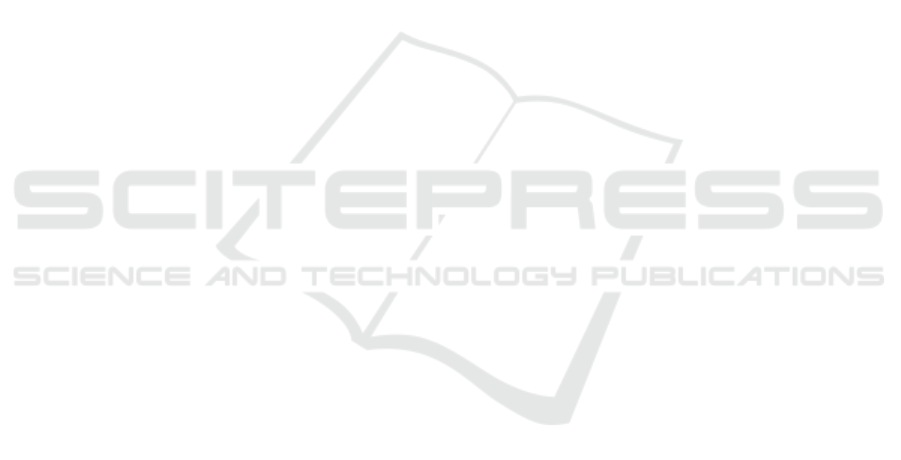
a large number of semantic similarity measures that
take into account different ontology properties and
object properties, providing more sophisticated sim-
ilarity measures(). There are several challenges in
measuring semantic similarity in the biomedical do-
main, namely how to address the multiple aspects
that a KG can represent in the context of a specific
application (Sousa et al., 2020), how to adequately
consider the specificity of ontology classes (Aouicha
and Taieb, 2016) and how to employ multiple ontolo-
gies(Ferreira and Couto, 2019). We have extensive
experience in biomedical semantic similarity, having
developed methods for its computation and evalua-
tion, e.g. (Pesquita, 2017; Cardoso et al., 2020).
4 CONCLUSIONS
This work proposes a methodology to enable seman-
tic explanations of machine learning applications in
the biomedical domain. The methodology tackles
the main challenges in providing human-centric ex-
planations based on a contextualized understanding
of the data and AI outcomes. It leverages the large
amounts of freely available biomedical data and meta-
data in the form of Knowledge Graphs, and builds
upon state of the art solutions for semantic annota-
tion and integration to embed the data and AI out-
comes with already established knowledge within the
domain. It then explores semantic similarity between
instances and between instances and outcomes to sup-
port similarity-based explanations. This methodology
affords post-hoc explanations that are built indepen-
dently of the machine learning algorithms employed,
and can thus be integrated into any application for
which data can be semantically annotated with exist-
ing biomedical ontologies.
In future work, we will employ this methodol-
ogy to build a semantic explanation system integrat-
ing our existing contributions in semantic annotation,
integration and similarity and apply it to the explana-
tion of biomedical machine learning applications, in-
cluding protein-protein interaction prediction, gene-
disease association and disease progression predic-
tion.
ACKNOWLEDGEMENTS
This work was funded by the Portuguese FCT through
the LASIGE Research Unit (UIDB/00408/2020 and
UIDP/00408/2020), and also by the SMILAX project
(PTDC/EEI-ESS/4633/2014).
REFERENCES
Alsentzer, E., Murphy, J., Boag, W., Weng, W.-H., Jindi,
D., Naumann, T., and McDermott, M. (2019). Pub-
licly available clinical bert embeddings. In Proceed-
ings of the 2nd Clinical Natural Language Processing
Workshop, pages 72–78.
Aouicha, M. B. and Taieb, M. A. H. (2016). Computing
semantic similarity between biomedical concepts us-
ing new information content approach. Journal of
biomedical informatics, 59:258–275.
Cardoso, C., Sousa, R. T., K
¨
ohler, S., and Pesquita, C.
(2020). A collection of benchmark data sets for
knowledge graph-based similarity in the biomedical
domain. Database, 2020.
Chari, S., Gruen, D. M., Seneviratne, O., and McGuinness,
D. L. (2020). Directions for explainable knowledge-
enabled systems.
Chen, J., Lecue, F., Pan, J., Horrocks, I., and Chen, H.
(2018). Knowledge-based transfer learning explana-
tion. In 16th International Conference on Principles
of Knowledge Representation and Reasoning, pages
349–358. AAAI Press.
d’Amato, C. (2020). Machine learning for the semantic
web: Lessons learnt and next research directions. Se-
mantic Web, (Preprint):1–9.
Faria, D., Pesquita, C., Mott, I., Martins, C., Couto, F. M.,
and Cruz, I. F. (2018). Tackling the challenges of
matching biomedical ontologies. Journal of biomedi-
cal semantics, 9(1):4.
Faria, D., Pesquita, C., Santos, E., Cruz, I. F., and Couto,
F. M. (2014). Automatic background knowledge se-
lection for matching biomedical ontologies. PloS one,
9(11):e111226.
Ferreira, J. D. and Couto, F. M. (2019). Multi-domain se-
mantic similarity in biomedical research. BMC bioin-
formatics, 20(10):23–31.
Ferreira, J. D., Teixeira, D. C., and Pesquita, C. (2020).
Biomedical ontologies: Coverage, access and use.
In Wolkenhauer, O., editor, Systems Medicine Inte-
grative, Qualitative and Computational Approaches,
pages 382 – 395. Academic Press, Elsevier.
Gonc¸alves, R. S., Kamdar, M. R., and Musen, M. A. (2019).
Aligning biomedical metadata with ontologies using
clustering and embeddings. In European Semantic
Web Conference, pages 146–161. Springer.
Guidotti, R., Monreale, A., Ruggieri, S., Turini, F., Gian-
notti, F., and Pedreschi, D. (2018). A survey of meth-
ods for explaining black box models. ACM computing
surveys (CSUR), 51(5):1–42.
Hitzler, P., Bianchi, F., Ebrahimi, M., and Sarker, M. K.
(2020). Neural-symbolic integration and the semantic
web. Semantic Web, 11(1):3–11.
Holzinger, A., Biemann, C., Pattichis, C. S., and Kell,
D. B. (2017). What do we need to build explainable
ai systems for the medical domain? arXiv preprint
arXiv:1712.09923.
Holzinger, A., Langs, G., Denk, H., Zatloukal, K., and
M
¨
uller, H. (2019). Causability and explainability of
HEALTHINF 2021 - 14th International Conference on Health Informatics
752