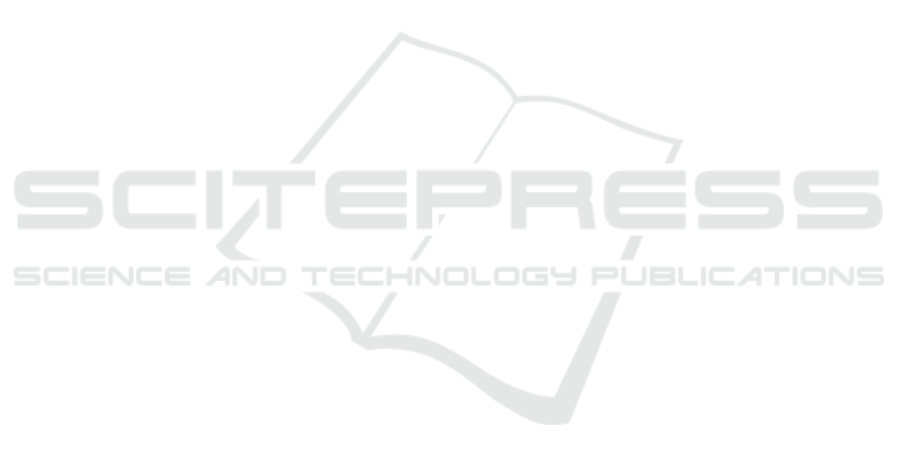
C¸ ic¸ek, O., Abdulkadir, A., Lienkamp, S., Brox, T., and Ron-
neberger, O. (2016). 3D U-Net: Learning Dense Vol-
umetric Segmentation from Sparse Annotation. In In-
ternational Conference on Medical Image Computing
and Computer-Assisted Intervention, pages 424–432.
Deng, J., Dong, W., Socher, R., Li, L.-J., Li, K., and Fei-
Fei, L. (2009). ImageNet: A Large-Scale Hierarchical
Image Database. In 2009 IEEE Conference on Com-
puter Vision and Pattern Recognition, pages 248–255.
IEEE.
Esteva, A., Kuprel, B., Novoa, R. A., Ko, J., Swetter, S. M.,
Blau, H. M., and Thrun, S. (2017). Dermatologist-
level classification of skin cancer with deep neural net-
works. Nature, 542(7639):115–118.
Geirhos, R., Rubisch, P., Michaelis, C., Bethge, M., Wich-
mann, F. A., and Brendel, W. (2018). ImageNet-
trained CNNs are biased towards texture; increasing
shape bias improves accuracy and robustness. arXiv
preprint arXiv:1811.12231.
Gil, J. Y. and Kimmel, R. (2002). Efficient Dilation, Ero-
sion, Opening,and Closing Algorithms. IEEE Trans-
actions on Pattern Analysis and Machine Intelligence,
24(12):1606–1617.
Hanssen, N., Burgielski, Z., Jansen, T., Lievin, M., Ritter,
L., von Rymon-Lipinski, B., and Keeve, E. (2004).
Nerves-level sets for interactive 3d segmentation of
nerve channels. In 2004 2nd IEEE International
Symposium on Biomedical Imaging: Nano to Macro
(IEEE Cat No. 04EX821), volume 1, pages 201–204.
Hwang, J.-J., Jung, Y.-H., Cho, B.-H., and Heo, M.-S.
(2019). An overview of deep learning in the field of
dentistry. Imaging science in dentistry, 49(1):1–7.
Jaju, P. P. and Jaju, S. P. (2014). Clinical utility of dental
cone-beam computed tomography: current perspec-
tives. Clinical, cosmetic and investigational dentistry,
6:29.
Kass, M., Witkin, A., and Terzopoulos, D. (1988). Snakes:
Active contour models. International Journal of Com-
puter Vision, 1(4):321–331.
Kondo, T., Ong, S., and Foong, K. W. (2004). Computer-
based extraction of the inferior alveolar nerve canal
in 3-D space. Computer Methods and Programs in
Biomedicine, 76(3):181–191.
Kwak, H., Kwak, E.-J., Song, J.-M., Park, H., Jung, Y.-H.,
Cho, B.-H., Hui, P., and Hwang, J. (2020). Automatic
mandibular canal detection using a deep convolutional
neural network. Scientific Reports, 10.
Li, Y., Yosinski, J., Clune, J., Lipson, H., and Hopcroft, J. E.
(2015). Convergent Learning: Do different neural net-
works learn the same representations? In Proceed-
ings of the 1st International Workshop on Feature Ex-
traction: Modern Questions and Challenges at NIPS
2015, pages 196–212.
Ligabue, G., Pollastri, F., Fontana, F., Leonelli, M., Furci,
L., Giovanella, S., Alfano, G., Cappelli, G., Testa, F.,
Bolelli, F., Grana, C., and Magistroni, R. (2020). Eval-
uation of the Classification Accuracy of the Kidney
Biopsy Direct Immunofluorescence through Convolu-
tional Neural Networks. Clinical Journal of the Amer-
ican Society of Nephrology, 15(10):1445–1454.
Loubele, M., Bogaerts, R., Van Dijck, E., Pauwels, R., Van-
heusden, S., Suetens, P., Marchal, G., Sanderink, G.,
and Jacobs, R. (2009). Comparison between effec-
tive radiation dose of CBCT and MSCT scanners for
dentomaxillofacial applications. European Journal of
Radiology, 71(3):461–468.
Ludlow, J. B. and Ivanovic, M. (2008). Comparative
dosimetry of dental CBCT devices and 64-slice CT for
oral and maxillofacial radiology. Oral Surgery, Oral
Medicine, Oral Pathology, Oral Radiology, and En-
dodontology, 106(1):106–114.
Nysj
¨
o, J. (2016). Interactive 3D Image Analysis for Cranio-
Maxillofacial Surgery Planning and Orthopedic Ap-
plications. PhD thesis, Uppsala University.
Pollastri, F., Bolelli, F., Paredes, R., and Grana, C. (2019).
Augmenting Data with GANs to Segment Melanoma
Skin Lesions. Multimedia Tools and Applications
Journal, 79(21-22):15575–15592.
Pollastri, F., Maro
˜
nas, J., Bolelli, F., Ligabue, G., Pare-
des, R., Magistroni, R., and Grana, C. (2021). Confi-
dence Calibration for Deep Renal Biopsy Immunoflu-
orescence Image Classification. In 2020 25th Interna-
tional Conference on Pattern Recognition (ICPR).
Ronneberger, O., Fischer, P., and Brox, T. (2015). U-Net:
Convolutional Networks for Biomedical Image Seg-
mentation. LNCS, 9351:234–241.
Silva, M. A. G., Wolf, U., Heinicke, F., Bumann, A., Visser,
H., and Hirsch, E. (2008). Cone-beam computed to-
mography for routine orthodontic treatment planning:
a radiation dose evaluation. American Journal of Or-
thodontics and Dentofacial Orthopedics, 133(5):640–
e1.
Sotthivirat, S. and Narkbuakaew, W. (2006). Automatic De-
tection of Inferior Alveolar Nerve Canals on CT Im-
ages. In 2006 IEEE Biomedical Circuits and Systems
Conference, pages 142–145.
Vinayahalingam, S., Xi, T., Berge, S., Maal, T., and
De Jong, G. (2019). Automated detection of third mo-
lars and mandibular nerve by deep learning. Scientific
Reports, 9.
A Cone Beam Computed Tomography Annotation Tool for Automatic Detection of the Inferior Alveolar Nerve Canal
731