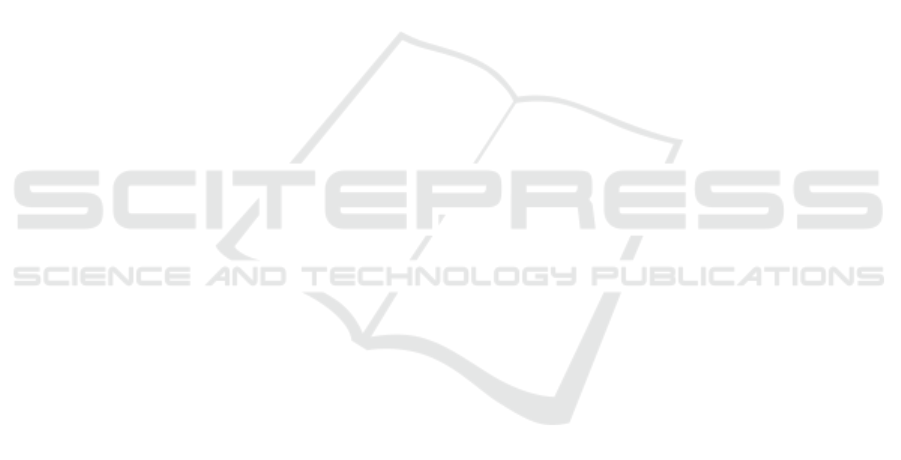
software platform for management, integration, and
analysis of histology for cancer research. Cancer Re-
search, 77(21):e75–e78.
Heusel, M., Ramsauer, H., Unterthiner, T., Nessler, B., and
Hochreiter, S. (2017). GANs Trained by a Two Time-
Scale Update Rule Converge to a Local Nash Equilib-
rium. arXiv:1706.08500.
Karras, T., Aila, T., Laine, S., and Lehtinen, J. (2018). Pro-
gressive Growing of GANs for Improved Quality, Sta-
bility, and Variation. arXiv:1710.10196 [cs, stat].
Karras, T., Aittala, M., Hellsten, J., Laine, S., Lehtinen, J.,
and Aila, T. (2020). Training Generative Adversar-
ial Networks with Limited Data. arXiv:2006.06676
[cs.CV].
Karras, T., Laine, S., and Aila, T. (2019). A style-based
generator architecture for generative adversarial net-
works. In Proceedings of the Conference on Computer
Vision and Pattern Recognition (CVPR).
Karras, T., Laine, S., Aittala, M., Hellsten, J., Lehtinen, J.,
and Aila, T. (2020). Analyzing and improving the im-
age quality of stylegan. In Proceedings of the Con-
ference on Computer Vision and Pattern Recognition,
pages 8110–8119.
Kazeminia, S., Baur, C., Kuijper, A., van Ginneken,
B., Navab, N., Albarqouni, S., and Mukhopadhyay,
A. (2018). GANs for Medical Image Analysis.
arXiv:1809.06222.
Li, W., Li, J., Sarma, K. V., Ho, K. C., Shen, S., Knudsen,
B. S., Gertych, A., and Arnold, C. W. (2019). Path
R-CNN for Prostate Cancer Diagnosis and Gleason
Grading of Histological Images. IEEE Transactions
on Medical Imaging, 38(4):945–954.
Martinez, K. and Cupitt, J. (2005). VIPS: a highly tuned im-
age processing software architecture. In IEEE Inter-
national Conference on Image Processing (31/08/05),
pages 574–577.
McGarry, S. D., Bukowy, J. D., Iczkowski, K. A., Un-
teriner, J. G., Duvnjak, P., Lowman, A. K., Jacob-
sohn, K., Hohenwalter, M., Griffin, M. O., Barring-
ton, A. W., Foss, H. E., Keuter, T., Hurrell, S. L., See,
W. A., Nevalainen, M. T., Banerjee, A., and LaVio-
lette, P. S. (2019). Gleason Probability Maps: A Ra-
diomics Tool for Mapping Prostate Cancer Likelihood
in MRI Space. Tomography, 5(1):127–134.
MICCAI (2020). Kaggle MICCAI 2020 prostate cancer
challenge.
Quiros, A. C., Murray-Smith, R., and Yuan, K. (2020).
PathologyGAN: Learning deep representations of
cancer tissue. arXiv:1907.02644 [cs, eess, stat].
Rawla, P. (2019). Epidemiology of Prostate Cancer. World
Journal of Oncology, 10(2):63–89.
Skandarani, Y., Painchaud, N., Jodoin, P.-M., and Lalande,
A. (2020). On the effectiveness of GAN generated
cardiac MRIs for segmentation. arXiv:2005.09026.
Xu, Z., Wang, X., Shin, H.-C., Yang, D., Roth, H., Milletari,
F., Zhang, L., and Xu, D. (2019). Correlation via syn-
thesis: end-to-end nodule image generation and radio-
genomic map learning based on generative adversarial
network. arXiv:1907.03728 [cs, eess].
Young, K., Booth, G., Simpson, B., Dutton, R., and Shrap-
nel, S. (2019). Deep neural network or dermatologist?
arXiv:1908.06612.
BIOIMAGING 2021 - 8th International Conference on Bioimaging
110