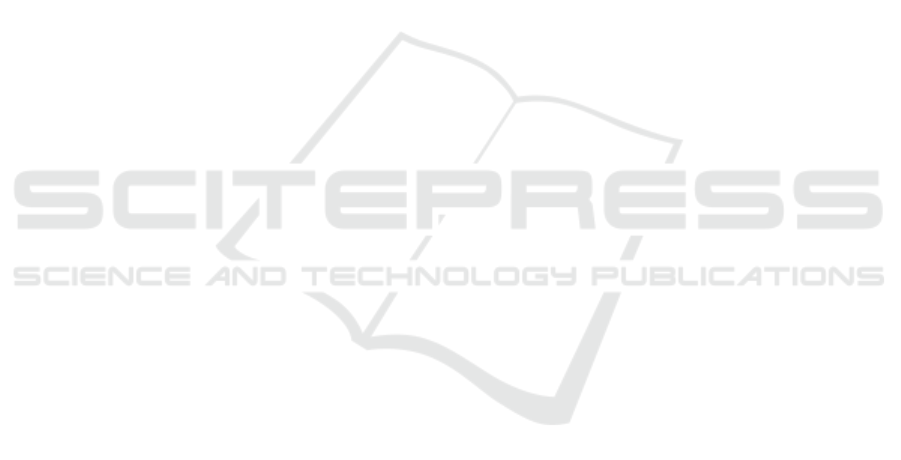
5 CONCLUSION
The development of MSL-ST, the first publicly avail-
able MS data batch search and export engine using
two of the most comprehensive MSLs is pivotal step
in MSL-chemoinformatics-based compound identifi-
cation. By offering a time-, cost- and labour-effective
solution for data extraction that can be easily im-
plemented in custom-made workflows, MSL-ST is
clearly addressing three of the current challenges of
chemoinformatics, that are: (1) centralization of mul-
tiple MSLs and uniformation of searching through the
empirical data they contain; (2) enabling search and
retrieval of batch data, instead of manually repeat-
ing the process, and (3) automated download of com-
pound data in a structured tabular format, instead of
time-consuming manual storage.
Since MSL-ST is the first MSLs batch search
and export engine, it can be easily assumed that
the idea of developing such tools in order to aim
chemoinformatics-assisted compound identification
is in its earliest infancy and thus, many more advance-
ments are to be added to the basic functionalities of
MSL-ST available in its first version, presented in this
paper. Consequently, its further upgrades would in-
clude:
• Addition of more publicly available MSLs, that
would allow access to larger amount of exepri-
mental and metadata, thus spreading the capabili-
ties of the MSL(s)-based chemoinformatics tools;
• The MS Type filter which indicates the number
and type of mass spectrometers used to generate
MS, and
• The Source Introduction filter that allows the se-
lection of the type of chromatography, whether it
is gas chromatography, liquid chromatography, or
capillary electrophoresis.
This would allow access to a large number of ex-
perimental data on compounds of different species
(metabolites, peptides, etc.). The process of real-
time data extraction would be expanded by searching
through other structured databases, using them as a
”living resource” that is updated daily.
The web application provides an easy way to se-
lect the characteristics of the mass spectrometry of the
whole pile of input compounds, which will be applied
in the search in the selected MSL. There is still room
for future work in improving the interface of the web
application, which would result in a better user expe-
rience and easier use of the application.
REFERENCES
DeBill, E. (2010). Module counts. WWW], http://www.
modulecounts. com/.[Haettu 1.11. 2016.].
Guijas, C., Montenegro-Burke, J. R., Domingo-Almenara,
X., Palermo, A., Warth, B., Hermann, G., Koel-
lensperger, G., Huan, T., Uritboonthai, W., Aisporna,
A. E., et al. (2018). Metlin: a technology platform for
identifying knowns and unknowns. Analytical chem-
istry, 90(5):3156–3164.
Holovaty, A. and Kaplan-Moss, J. (2009). The defini-
tive guide to Django: Web development done right.
Apress.
Horai, H., Suwa, K., Arita, M., Nihei, Y., and Nishioka,
T. (2020 (accessed November 16, 2020)). Massbank:
Mass spectral database for metabolome analysis. In
The 56th ASMS Conference on Mass Spectrometry
and Allied Topics, Denver, CO.
Hummel, J., Selbig, J., Walther, D., and Kopka, J. (2007).
The golm metabolome database: a database for gc-ms
based metabolite profiling. In Metabolomics, pages
75–95. Springer.
Hummel, J., Strehmel, N., Selbig, J., Walther, D., and
Kopka, J. (2010). Decision tree supported substruc-
ture prediction of metabolites from gc-ms profiles.
Metabolomics, 6(2):322–333.
Kind, T., Wohlgemuth, G., Lee, D. Y., Lu, Y., Pala-
zoglu, M., Shahbaz, S., and Fiehn, O. (2009). Fiehn-
lib: mass spectral and retention index libraries for
metabolomics based on quadrupole and time-of-flight
gas chromatography/mass spectrometry. Analytical
chemistry, 81(24):10038–10048.
Ljoncheva, Milka and Stepi
ˇ
snik, Toma
ˇ
z and D
ˇ
zeroski, Sa
ˇ
so
and Kosjek, Tina (2020). Cheminformatics in MS-
based environmental exposomics: Current achieve-
ments and future directions. Trends in Environmental
Analytical Chemistry, page e00099.
McLafferty, F. W. and Stauffer, D. (2009). Wiley registry of
mass spectral data, volume 662. John Wiley Hobo-
ken, NJ.
Mehta, S. (2020 (accessed November 16, 2020)). Mass-
bank of north america (mona): An open-access, auto-
curating mass spectral database for compound identi-
fication in metabolomics presentation.
NIST: National Institue of Standard and Technology (2020
(accessed November 16, 2020)). The nist mass spec-
trometry data center.
Rasche, F., Scheubert, K., Hufsky, F., Zichner, T., Kai, M.,
Svato
ˇ
s, A., and B
¨
ocker, S. (2012). Identifying the un-
knowns by aligning fragmentation trees. Analytical
chemistry, 84(7):3417–3426.
Sawada, Y., Nakabayashi, R., Yamada, Y., Suzuki, M.,
Sato, M., Sakata, A., Akiyama, K., Sakurai, T.,
Matsuda, F., Aoki, T., et al. (2012). Riken tan-
dem mass spectral database (respect) for phytochem-
icals: a plant-specific ms/ms-based data resource and
database. Phytochemistry, 82:38–45.
Scheubert, K., Hufsky, F., and B
¨
ocker, S. (2013). Computa-
tional mass spectrometry for small molecules. Journal
of cheminformatics, 5(1):12.
BIOINFORMATICS 2021 - 12th International Conference on Bioinformatics Models, Methods and Algorithms
202