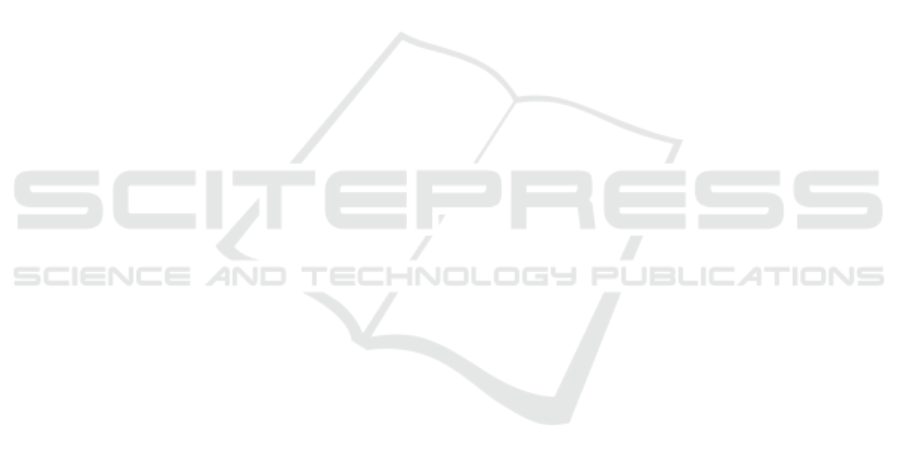
came the technical limitations of document-pivot and
feature-pivot approaches, and recalled more key and
non-key topics than Zhao et al. (2011)’s algorithm.
Beyond the gains in performance, the combination of
document-pivot and feature-pivot techniques allowed
ELD to understand events better by extracting both
tweets and topical keywords.
Still, TDT’s road to harness Twitter’s advan-
tages remains a long one. It is still necessary to
find a balance between detecting non-key topics dur-
ing an event and minimizing noise, especially in
emotionally-charged events. Moreover, while elimi-
nating noise remains a significant challenge, tracking
also needs to improve to minimize redundant report-
ing, which remains an understated problem in TDT.
ACKNOWLEDGEMENTS
The research work disclosed in this publication is par-
tially funded by the Endeavour Scholarship Scheme
(Malta). Scholarships are part-financed by the Euro-
pean Union - European Social Fund (ESF) - Oper-
ational Programme II - Cohesion Policy 2014-2020
“Investing in human capital to create more opportu-
nities and promote the well-being of society”.
REFERENCES
Aiello, L. M., Petkos, G., Martin, C., Corney, D., Pa-
padopoulos, S., Skraba, R., Goker, A., Kompatsiaris,
I., and Jaimes, A. (2013). Sensing Trending Top-
ics in Twitter. IEEE Transactions on Multimedia,
15(6):1268–1282.
Carbonell, J. and Goldstein, J. (1998). The Use of
MMR, Diversity-Based Reranking for Reordering
Documents and Producing Summaries. In SIGIR ’98:
Proceedings of the 21st Annual International ACM SI-
GIR Conference on Research and Development in In-
formation Retrieval, page 335–336, Melbourne, Aus-
tralia. Association for Computing Machinery.
Cataldi, M., Caro, L. D., and Schifanella, C. (2013). Per-
sonalized Emerging Topic Detection Based on a Term
Aging Model. ACM Transactions on Intelligent Sys-
tems and Technology (TIST), 5(1):1–27.
Farzindar, A. and Khreich, W. (2015). A Survey of Tech-
niques for Event Detection in Twitter. Computational
Intelligence, 31(1):132–164.
Gillani, M., Ilyas, M. U., Saleh, S., Alowibdi, J. S., Aljo-
hani, N., and Alotaibi, F. S. (2017). Post Summariza-
tion of Microblogs of Sporting Events. In Proceed-
ings of the 26th International Conference on World
Wide Web Companion, pages 59–68, Perth, Australia.
International World Wide Web Conferences Steering
Committee.
Ifrim, G., Shi, B., and Brigadir, I. (2014). Event Detection
in Twitter using Aggressive Filtering and Hierarchical
Tweet Clustering. In Proceedings of the SNOW 2014
Data Challenge, page 33–40, Seoul, Korea. CEUR.
Lanagan, J. and Smeaton, A. F. (2011). Using Twitter to
Detect and Tag Important Events in Live Sports. In
Proceedings of the Fifth International AAAI Confer-
ence on Weblogs and Social Media, page 542–545,
Barcelona, Spain. Association for the Advancement
of Artificial Intelligence.
Mamo, N. and Azzopardi, J. (2017). FIRE: Finding Impor-
tant News REports. In Szyma
´
nski, J. and Velegrakis,
Y., editors, Semantic Keyword-Based Search on Struc-
tured Data Sources, pages 20–31, Gdansk, Poland.
Springer International Publishing.
Mamo, N., Azzopardi, J., and Layfield, C. (2019). ELD:
Event TimeLine Detection – A Participant-Based Ap-
proach to Tracking Events. In HT ’19: Proceedings
of the 30th ACM Conference on Hypertext and Social
Media, pages 267–268, Hof, Germany. ACM.
Meladianos, P., Nikolentzos, G., Rousseau, F., Stavrakas,
Y., and Vazirgiannis, M. (2015). Degeneracy-Based
Real-Time Sub-Event Detection in Twitter Stream. In
Proceedings of the Ninth International AAAI Confer-
ence on Web and Social Media, page 248–257, Ox-
ford, United Kingdom. The AAAI Press.
Reed, J. W., Jiao, Y., Potok, T. E., Klump, B. A., Elmore,
M. T., and Hurson, A. R. (2006). TF-ICF: A New
Term Weighting Scheme for Clustering Dynamic Data
Streams. In 2006 5th International Conference on Ma-
chine Learning and Applications (ICMLA’06), pages
258–263, Orlando, Florida, USA. IEEE.
Saeed, Z., Abbasi, R. A., Maqbool, O., Sadaf, A., Razzak,
I., Daud, A., Aljohani, N. R., and Xu, G. (2019a).
What’s Happening Around the World? A Survey and
Framework on Event Detection Techniques on Twit-
ter. Journal of Grid Computing, 17(2):279–312.
Saeed, Z., Abbasi, R. A., Razzak, I., Maqbool, O., Sadaf,
A., and Xu, G. (2019b). Enhanced Heartbeat Graph
for Emerging Event Detection on Twitter using Time
Series Networks. Expert Systems with Applications,
136:115–132.
Unankard, S., Li, X., and Sharaf, M. A. (2015). Emerg-
ing Event Detection in Social Networks with Location
Sensitivity. World Wide Web, 18:1393–1417.
Weiler, A., Beel, J., Gipp, B., and Grossniklaus, M. (Oct
13, 2016). Stability Evaluation of Event Detection
Techniques for Twitter. In Lecture Notes in Com-
puter Science book series (LNCS, volume 9897), page
368–380, Stockholm, Sweden. Springer.
Weiler, A., Schilling, H., Kircher, L., and Grossniklaus, M.
(Jun 14, 2019). Towards Reproducible Research of
Event Detection Techniques for Twitter . In 2019 6th
Swiss Conference on Data Science (SDS), pages 69–
74, Bern, Switzerland. IEEE.
Zhao, S., Zhong, L., Wickramasuriya, J., and Vasudevan,
V. (2011). Human as real-time sensors of social and
physical events: A case study of twitter and sports
games.
KDIR 2021 - 13th International Conference on Knowledge Discovery and Information Retrieval
86