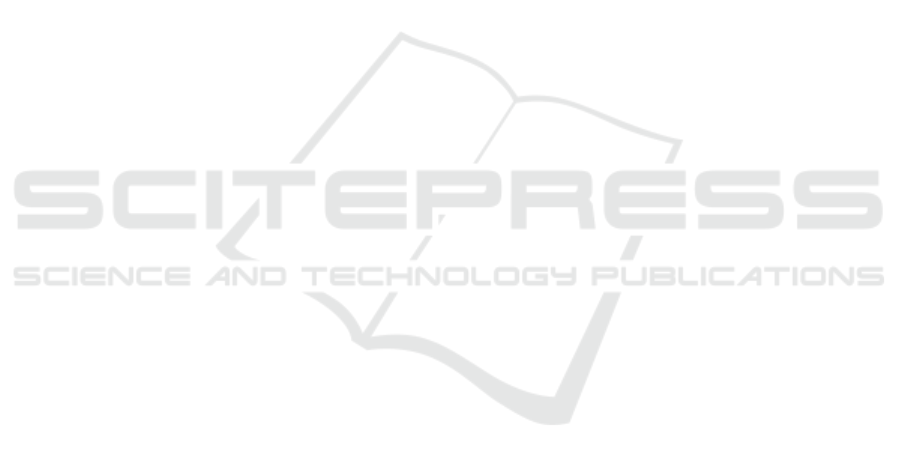
ACKNOWLEDGEMENTS
Supported by Innosuisse Project Nr. 26281.2 PFES-
ES.
REFERENCES
Bhowmick, S. S., Choi, B., and Zhou, S. (2013). VOGUE:
Towards A Visual Interaction-aware Graph Query
Processing Framework. In Cidr. Citeseer.
Francis, N., Green, A., Guagliardo, P., Libkin, L., Lin-
daaker, T., Marsault, V., Plantikow, S., Rydberg, M.,
Selmer, P., and Taylor, A. (2018). Cypher: An evolv-
ing query language for property graphs. In Proceed-
ings of the 2018 International Conference on Manage-
ment of Data, pages 1433–1445.
Gladisch, S., Schumann, H., and Tominski, C. (2013). Nav-
igation recommendations for exploring hierarchical
graphs. In International Symposium on Visual Com-
puting, pages 36–47. Springer.
Jayaram, N., Khan, A., Li, C., Yan, X., and Elmasri, R.
(2015). Querying knowledge graphs by example en-
tity tuples. IEEE Transactions on Knowledge and
Data Engineering, 27(10):2797–2811.
Jin, C., Bhowmick, S. S., Choi, B., and Zhou, S. (2012).
PRAGUE: A practical framework for blending visual
subgraph query formulation and query processing. In
In ICDE, volume 10. Citeseer.
Liang, S., Stockinger, K., de Farias, T. M., Anisimova, M.,
and Gil, M. (2021). Querying knowledge graphs in
natural language. Journal of Big Data, 8(1):1–23.
Lissandrini, M., Mottin, D., Palpanas, T., and Velegrakis,
Y. (2020a). Graph-query suggestions for knowledge
graph exploration. In Proceedings of The Web Con-
ference 2020, pages 2549–2555.
Lissandrini, M., Pedersen, T. B., Hose, K., and Mottin, D.
(2020b). Knowledge graph exploration: where are we
and where are we going? ACM SIGWEB Newsletter,
(Summer 2020):1–8.
May, T., Steiger, M., Davey, J., and Kohlhammer, J. (2012).
Using signposts for navigation in large graphs. In
Computer Graphics Forum, volume 31, pages 985–
994. Wiley Online Library.
Mohanty, M. and Ramanath, M. (2019). Insta-search: To-
wards effective exploration of knowledge graphs. In
Proceedings of the 28th ACM International Confer-
ence on Information and Knowledge Management,
pages 2909–2912.
Mottin, D., Bonchi, F., and Gullo, F. (2015). Graph
query reformulation with diversity. In Proceedings of
the 21th ACM SIGKDD International Conference on
Knowledge Discovery and Data Mining, pages 825–
834.
Namaki, M. H., Wu, Y., and Zhang, X. (2018). Gexp: Cost-
aware graph exploration with keywords. In Proceed-
ings of the 2018 International Conference on Manage-
ment of Data, pages 1729–1732.
P
´
erez, J., Arenas, M., and Gutierrez, C. (2009). Seman-
tics and complexity of sparql. ACM Transactions on
Database Systems (TODS), 34(3):1–45.
Pienta, R., Hohman, F., Endert, A., Tamersoy, A., Roundy,
K., Gates, C., Navathe, S., and Chau, D. H. (2017).
Vigor: interactive visual exploration of graph query
results. IEEE transactions on visualization and com-
puter graphics, 24(1):215–225.
Riesen, K. (2020). A novel data set for information re-
trieval on the basis of subgraph matching. In Struc-
tural, Syntactic, and Statistical Pattern Recognition:
Joint IAPR International Workshops, S+ SSPR 2020,
Padua, Italy, January 21–22, 2021, Proceedings, page
205.
Saha, A., Pahuja, V., Khapra, M. M., Sankaranarayanan, K.,
and Chandar, S. (2018). Complex sequential question
answering: Towards learning to converse over linked
question answer pairs with a knowledge graph. In
Thirty-Second AAAI Conference on Artificial Intelli-
gence.
Shekarpour, S., Marx, E., Auer, S., and Sheth, A. (2017).
Rquery: rewriting natural language queries on knowl-
edge graphs to alleviate the vocabulary mismatch
problem. In Proceedings of the AAAI Conference on
Artificial Intelligence, volume 31.
Tong, P., Zhang, Q., and Yao, J. (2019). Leveraging domain
context for question answering over knowledge graph.
Data Science and Engineering, 4(4):323–335.
Van Ham, F. and Perer, A. (2009). “search, show con-
text, expand on demand”: Supporting large graph ex-
ploration with degree-of-interest. IEEE Transactions
on Visualization and Computer Graphics, 15(6):953–
960.
White, S. and Smyth, P. (2003). Algorithms for estimat-
ing relative importance in networks. In Proceedings
of the ninth ACM SIGKDD international conference
on Knowledge discovery and data mining, pages 266–
275.
Witschel, H. F., Riesen, K., and Grether, L. (2020). Kvgr: A
graph-based interface for explorative sequential ques-
tion answering on heterogeneous information sources.
In European Conference on Information Retrieval,
pages 760–773. Springer.
Wu, Y., Yang, S., Srivatsa, M., Iyengar, A., and Yan, X.
(2013). Summarizing answer graphs induced by key-
word queries. Proceedings of the VLDB Endowment,
6(14):1774–1785.
Yang, S., Xie, Y., Wu, Y., Wu, T., Sun, H., Wu, J., and Yan,
X. (2014). SLQ: a user-friendly graph querying sys-
tem. In Proceedings of the 2014 ACM SIGMOD Inter-
national Conference on Management of Data, pages
893–896. ACM.
Yi, P., Choi, B., Bhowmick, S. S., and Xu, J. (2017). Autog:
a visual query autocompletion framework for graph
databases. The VLDB Journal, 26(3):347–372.
Yogev, S., Roitman, H., Carmel, D., and Zwerdling, N.
(2012). Towards expressive exploratory search over
entity-relationship data. In Proceedings of the 21st
International Conference on World Wide Web, pages
83–92.
KDIR 2021 - 13th International Conference on Knowledge Discovery and Information Retrieval
102