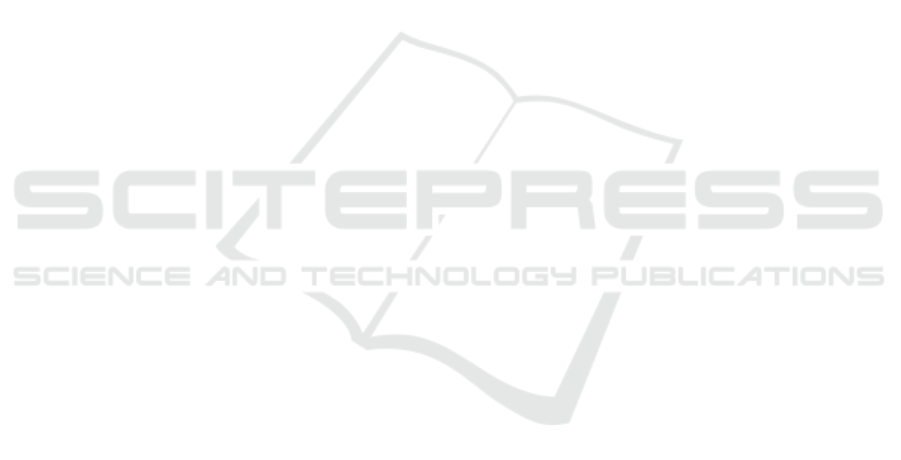
accounts for carbon emissions (Lin et al., 2014), or
VRP with soft timing constraints, which if violated
we must account for a penalty. Ambitious future work
can also account for traffic, i.e. higher cost routes in
city centers (Rizzoli et al., 2004) in what is known as
time dependent VRPTW. Another extension could be
to target split delivery vehicle routing problem with
time windows (SDVRPTW) where the delivery to a
customer can be split between any number of vehicles
resulting in considerable improvements in terms num-
ber of vehicles used and cost (Da Silva et al., 2014;
Feillet et al., 2006).
On the other hand, this work can be generalized to
address a different class of VRP altogether, namely
the VRP with backhauls (VRPB) (Toth and Vigo,
2001). Lastly, it is also interesting for future work
to add problem knowledge to the reproduction oper-
ators such as the one proposed in (Hsu and Chiang,
2012).
ACKNOWLEDGEMENTS
We thank dearly Dr. Janka Chlebikova and Dr. Mo-
hamad Bader-El-Den from the School of Computing
at University of Portsmouth for their valuable support.
Numerical computations were done on the Sciama
High Performance Compute (HPC) cluster which is
supported by the ICG, SEPNet and the University of
Portsmouth.
REFERENCES
Bychkov, I. and Batsyn, M. (2018). A hybrid approach
for the capacitated vehicle routing problem with time
windows. In OPTA-SCL. CEUR Workshop Proceed-
ings.
Chiang, T.-C. and Hsu, W.-H. (2014). A knowledge-based
evolutionary algorithm for the multiobjective vehicle
routing problem with time windows. Computers &
Operations Research, 45:25–37.
Czech, Z. J. and Czarnas, P. (2002). Parallel simulated
annealing for the vehicle routing problem with time
windows. In Proceedings 10th Euromicro workshop
on parallel, distributed and network-based process-
ing, pages 376–383. IEEE.
Da Silva, M. D. M., Subramanian, A., and Ochi, L. S.
(2014). An iterated local search heuristic for the split
delivery vehicle routing problem. In ROADEF-15
`
eme
congr
`
es annuel de la Soci
´
et
´
e franc¸aise de recherche
op
´
erationnelle et d’aide
`
a la d
´
ecision.
Dash, M. and Anekal, B. I. (2014). Variables reduction in
vehicle routing problems. SPC ERA IJBM, 2(6).
Dixit, A., Mishra, A., and Shukla, A. (2019). Vehicle rout-
ing problem with time windows using meta-heuristic
algorithms: a survey. In Harmony search and na-
ture inspired optimization algorithms, pages 539–546.
Springer.
Feillet, D., Dejax, P., Gendreau, M., and Gueguen, C.
(2006). Vehicle routing with time windows and split
deliveries. Technical Paper, 851.
Garcia-Najera, A. and Bullinaria, J. A. (2011). An im-
proved multi-objective evolutionary algorithm for the
vehicle routing problem with time windows. Comput-
ers & Operations Research, 38(1):287–300.
Hsu, W.-H. and Chiang, T.-C. (2012). A multiobjective evo-
lutionary algorithm with enhanced reproduction oper-
ators for the vehicle routing problem with time win-
dows. In 2012 IEEE Congress on Evolutionary Com-
putation, pages 1–8. IEEE.
Ishibuchi, H., Imada, R., Setoguchi, Y., and Nojima, Y.
(2018). How to specify a reference point in hyper-
volume calculation for fair performance comparison.
Evolutionary computation, 26(3):411–440.
Jawarneh, S. and Abdullah, S. (2015). Sequential insertion
heuristic with adaptive bee colony optimisation algo-
rithm for vehicle routing problem with time windows.
PloS one, 10(7):e0130224.
King, R. A., Deb, K., and Rughooputh, H. (2010). Compar-
ison of nsga-ii and spea2 on the multiobjective envi-
ronmental/economic dispatch problem. University of
Mauritius Research Journal, 16(1):485–511.
Li, W., Dai, H., and Zhang, D. (2015). The relationship
between maximum completion time and total comple-
tion time in flowshop production. Procedia Manufac-
turing, 1:146–156.
Lin, C., Choy, K. L., Ho, G. T., Chung, S. H., and Lam, H.
(2014). Survey of green vehicle routing problem: past
and future trends. Expert systems with applications,
41(4):1118–1138.
Luke, S. (2013). Essentials of metaheuristics. lulu, 2013.
Metaheuristics in large-scale global continues op-
timization: A survey, available at: http://cs. gmu.
edu/ sean/book/metaheuristics.
Marrouche, W., Farah, R., and Harmanani, H. M. (2018).
A strength pareto evolutionary algorithm for optimiz-
ing system-on-chip test schedules. International Jour-
nal of Computational Intelligence and Applications,
17(02):1850010.
Meryem, B. and Abdelmadjid, B. (2015). Resolving a ve-
hicle routing problem with heterogeneous fleet, mixed
backhauls and time windows using cuckoo behaviour
approach. International Journal of Operational Re-
search, 24(2):132–144.
Messaoud, E., El Idrissi, A. E. B., and Alaoui, A. E. (2018).
The green dynamic vehicle routing problem in sus-
tainable transport. In 2018 4th International Con-
ference on Logistics Operations Management (GOL),
pages 1–6. IEEE.
Minocha, B., Tripathi, S., and Mohan, C. (2011). Solving
vehicle routing and scheduling problems using hybrid
genetic algorithm. In 2011 3rd international confer-
ence on electronics computer technology, volume 2,
pages 189–193. Ieee.
A Strength Pareto Evolutionary Algorithm for Solving the Capacitated Vehicle Routing Problem with Time Windows
105