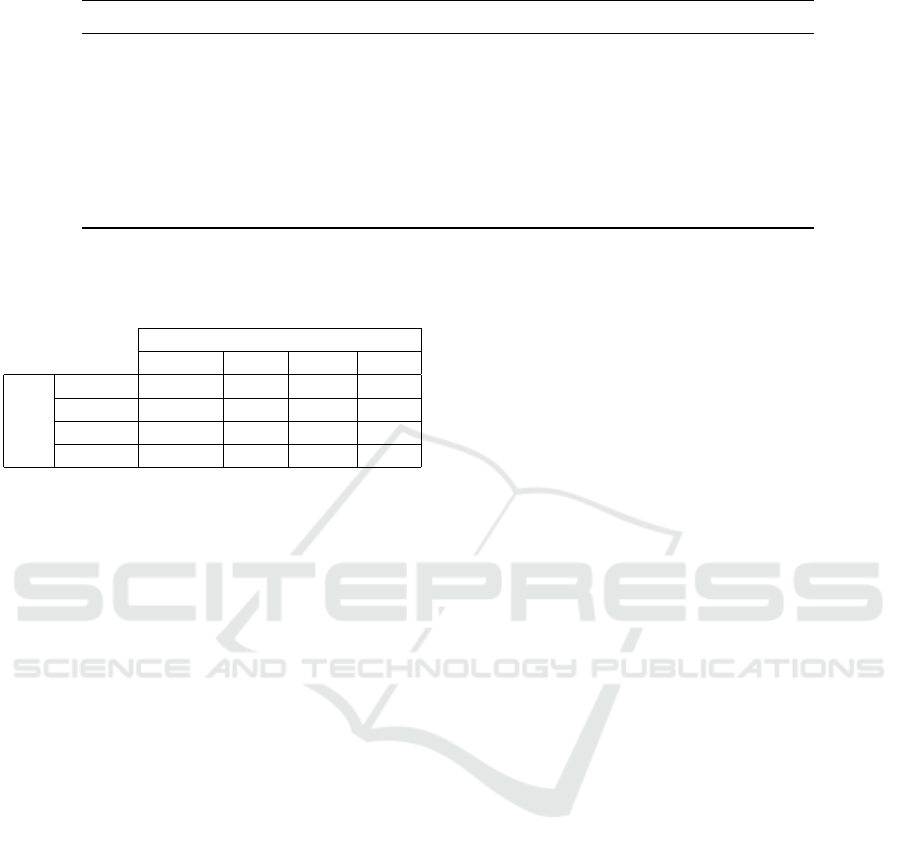
Table 6: Comparison of performances by different combinations of sensors.
# Fixed camera Smart watch EOG EEG ETG ECG 3 classes 2 classes
1 X 51.1 66.7
2 X 53.3 67.8
3 X X 56.7 66.7
4 X X X 52.2 67.8
5 X X X 53.3 70.0
6 X X X 53.3 72.2
7 X X X 68.5 75.8
8 X X X X X X 74.4 82.2
Table 7: Evaluation result of leave-one-subject-out cross
validation. Time: time-pressure condition, Multi: multi-
task condition, GT: ground truth.
Estimated
Normal Time Multi Total
GT
Normal 1.67 0.44 0.56 2.67
Time 0.67 1.22 0.44 2.33
Multi 0.56 0.56 1.67 2.78
Total 2.89 2.22 2.67 7.78
portant role in estimating human-error potential. Our
future work includes generalizing out method to new
workers by finding out subject-independent features.
REFERENCES
Akiba, T., Sano, S., Yanase, T., Ohta, T., and Koyama, M.
(2019). Optuna: A next-generation hyperparameter
optimization framework. In International conference
on knowledge discovery & data mining.
Bergstra, J., Bardenet, R., Bengio, Y., and K
´
egl, B. (2011).
Algorithms for hyper-parameter optimization. In 25th
annual conference on neural information processing
systems (NIPS).
Edmondson, A. C. (2004). Learning from mistakes is easier
said than done: Group and organizational influences
on the detection and correction of human error. The
Journal of Applied Behavioral Science, 40(1):66–90.
Hale, A., Stoop, J., and Hommels, J. (1990). Human er-
ror models as predictors of accident scenarios for de-
signers in road transport systems. Ergonomics, 33(10-
11):1377–1387.
Hawkins, F. H. (1987). Human Factors in Flight. Ashgate
Publishing.
Panicker, S. S. and Gayathri, P. (2019). A survey of ma-
chine learning techniques in physiology based mental
stress detection systems. Biocybernetics and Biomed-
ical Engineering, 39(2):444–469.
Pavllo, D., Feichtenhofer, C., Grangier, D., and Auli, M.
(2019). 3D human pose estimation in video with tem-
poral convolutions and semi-supervised training. In
Conference on Computer Vision and Pattern Recogni-
tion (CVPR).
Ralph, B. C., Thomson, D. R., Cheyne, J. A., and Smilek,
D. (2014). Media multitasking and failures of at-
tention in everyday life. Psychological research,
78(5):661–669.
Ramzan, M., Khan, H. U., Awan, S. M., Ismail, A., Ilyas,
M., and Mahmood, A. (2019). A survey on state-of-
the-art drowsiness detection techniques. IEEE Access,
7:61904–61919.
Rasmussen, J. (1983). Skills, rules, and knowledge; signals,
signs, and symbols, and other distinctions in human
performance models. IEEE transactions on systems,
man, and cybernetics, SMC-13(3):257–266.
Reason, J. (1990). Human Error. Cambridge University
Press.
Sahayadhas, A., Sundaraj, K., and Murugappan, M. (2012).
Detecting Driver Drowsiness Based on Sensors: A
Review. Sensors, 12(12):16937–16953.
Sikander, G. and Anwar, S. (2019). Driver Fatigue Detec-
tion Systems: A Review. IEEE Transactions on Intel-
ligent Transportation Systems, 20(6):2339–2352.
Sun, F.-T., Kuo, C., Cheng, H.-T., Buthpitiya, S., Collins, P.,
and Griss, M. (2010). Activity-aware Mental Stress
Detection Using Physiological Sensors. In Interna-
tional conference on Mobile computing, applications,
and services, pages 282–301. Springer.
Sun, M., Tsujikawa, M., Onishi, Y., Ma, X., Nishino, A.,
and Hashimoto, S. (2018). A neural-network-based
investigation of eye-related movements for accurate
drowsiness estimation. In International Conference
of the IEEE Engineering in Medicine and Biology So-
ciety (EMBC), pages 5207–5210.
Swain, A. D. and Guttmann, H. E. (1983). Handbook of
human reliability analysis with emphasis on nuclear
power plant applications. NUREG/CR-1278, SAND
80-0200.
Tsujikawa, M., Onishi, Y., Kiuchi, Y., Ogatsu, T., Nishino,
A., and Hashimoto, S. (2018). Drowsiness estimation
from low-frame-rate facial videos using eyelid vari-
ability features. In International Conference of the
IEEE Engineering in Medicine and Biology Society
(EMBC), pages 5203–5206.
Uema, Y. and Inoue, K. (2017). JINS MEME Algorithm for
Estimation and Tracking of Concentration of Users. In
Proceedings of the ACM International Joint Confer-
ence on Pervasive and Ubiquitous Computing and the
Human-error-potential Estimation based on Wearable Biometric Sensors
119