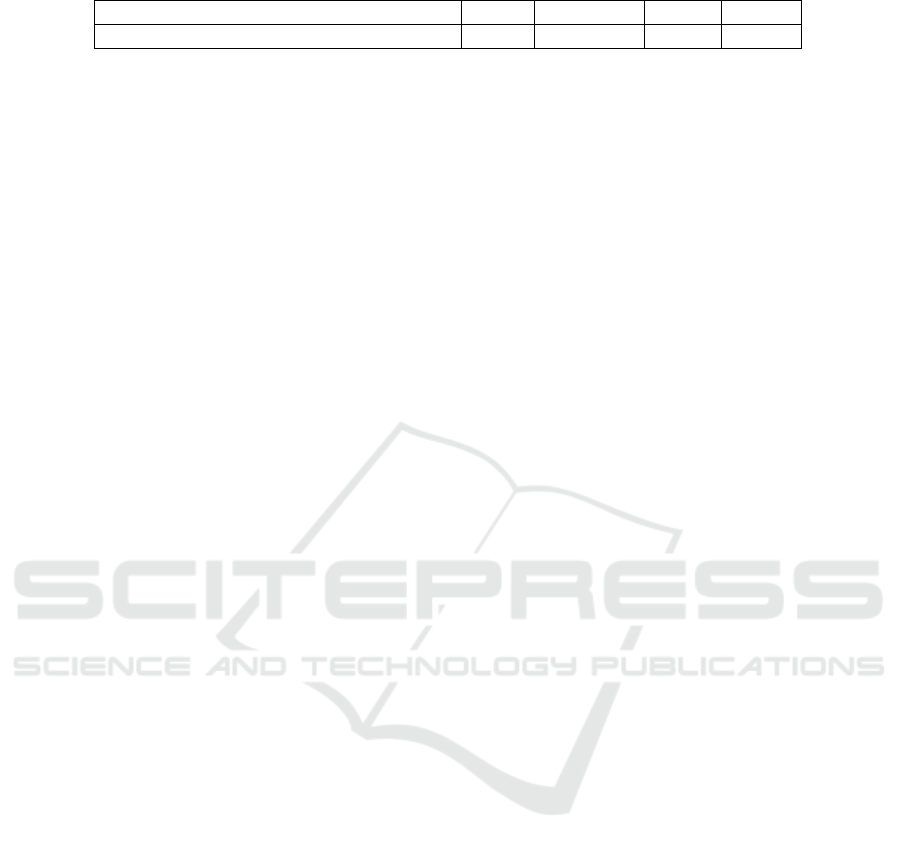
Table 8: Complete Stacking BERT models.
Model \Dataset AJGT LargeASA LABR HARD
ASA-medium BERT+ mBERT +AraBERT 93% 91% 88% 95%
5 CONCLUSION
In this paper, we proposed a stacked generalization
approach for Arabic sentiment analysis. We have
used Medium Arabic BERT, AraBERT and mBERT
as base models. Firstly, we proved that by implement-
ing a single arabic medium BERT model we outper-
form the state of the art for ASA. Secondly, the ex-
periment results showed that the stacking strategy im-
proves the accuracy. As a continuity of this contribu-
tion, we plan to generalize our results to the sentiment
analysis with intensities case.
REFERENCES
Abdelali, A., Hassan, S., Mubarak, H., Darwish, K.,
and Samih, Y. (2021). Pre-training bert on ara-
bic tweets: Practical considerations. arXiv preprint
arXiv:2102.10684.
Abdul-Mageed, M., Elmadany, A., and Nagoudi, E. M. B.
(2020). Arbert & marbert: Deep bidirectional trans-
formers for arabic. arXiv preprint arXiv:2101.01785.
Al-Rubaiee, H., Qiu, R., and Li, D. (2016). Identifying
mubasher software products through sentiment analy-
sis of arabic tweets. In 2016 International Conference
on Industrial Informatics and Computer Systems (CI-
ICS), pages 1–6. IEEE.
Alhumoud, S., Albuhairi, T., and Alohaideb, W. (2015a).
Hybrid sentiment analyser for arabic tweets using r.
In 2015 7th International Joint Conference on Knowl-
edge Discovery, Knowledge Engineering and Knowl-
edge Management (IC3K), volume 1, pages 417–424.
IEEE.
Alhumoud, S., Albuhairi, T., and Alohaideb, W. (2015b).
Hybrid sentiment analyser for arabic tweets using r.
In 2015 7th International Joint Conference on Knowl-
edge Discovery, Knowledge Engineering and Knowl-
edge Management (IC3K), volume 1, pages 417–424.
IEEE.
Alomari, K. M., ElSherif, H. M., and Shaalan, K. (2017).
Arabic tweets sentimental analysis using machine
learning. In International Conference on Industrial,
Engineering and Other Applications of Applied Intel-
ligent Systems, pages 602–610. Springer.
Aly, M. and Atiya, A. (2013). Labr: A large scale ara-
bic book reviews dataset. In Proceedings of the 51st
Annual Meeting of the Association for Computational
Linguistics (Volume 2: Short Papers), pages 494–498.
Antoun, W., Baly, F., and Hajj, H. (2020). Arabert:
Transformer-based model for arabic language under-
standing. arXiv preprint arXiv:2003.00104.
Baly, R., Khaddaj, A., Hajj, H., El-Hajj, W., and Sha-
ban, K. B. (2019). Arsentd-lev: A multi-topic corpus
for target-based sentiment analysis in arabic levantine
tweets. arXiv preprint arXiv:1906.01830.
Chouikhi, H., Chniter, H., and Jarray, F. (2021). Arabic
sentiment analysis using bert model. In 13th Interna-
tional Conference on Computational Collective Intel-
ligence (ICCCI). Springer, Cham.
Dahou, A., Elaziz, M. A., Zhou, J., and Xiong, S. (2019).
Arabic sentiment classification using convolutional
neural network and differential evolution algorithm.
Computational intelligence and neuroscience, 2019.
Devlin, J., Chang, M.-W., Lee, K., and Toutanova, K.
(2018). Bert: Pre-training of deep bidirectional trans-
formers for language understanding. arXiv preprint
arXiv:1810.04805.
Dragoni, M., Poria, S., and Cambria, E. (2018). Ontosentic-
net: A commonsense ontology for sentiment analysis.
IEEE Intelligent Systems, 33(3):77–85.
ElJundi, O., Antoun, W., El Droubi, N., Hajj, H., El-Hajj,
W., and Shaban, K. (2019). hulmona: The univer-
sal language model in arabic. In Proceedings of the
Fourth Arabic Natural Language Processing Work-
shop, pages 68–77.
Elnagar, A., Khalifa, Y. S., and Einea, A. (2018). Ho-
tel arabic-reviews dataset construction for sentiment
analysis applications. In Intelligent natural language
processing: Trends and applications, pages 35–52.
Springer.
Eskander, R. and Rambow, O. (2015). Slsa: A sentiment
lexicon for standard arabic. In Proceedings of the
2015 conference on empirical methods in natural lan-
guage processing, pages 2545–2550.
Farha, I. A. and Magdy, W. (2020). From arabic sentiment
analysis to sarcasm detection: The arsarcasm dataset.
In Proceedings of the 4th Workshop on Open-Source
Arabic Corpora and Processing Tools, with a Shared
Task on Offensive Language Detection, pages 32–39.
Ghanem, B., Karoui, J., Benamara, F., Moriceau, V., and
Rosso, P. (2019). Idat at fire2019: Overview of the
track on irony detection in arabic tweets. In Proceed-
ings of the 11th Forum for Information Retrieval Eval-
uation, pages 10–13.
Harrat, S., Meftouh, K., and Smaili, K. (2019). Machine
translation for arabic dialects (survey). Information
Processing & Management, 56(2):262–273.
Imran, A., Faiyaz, M., and Akhtar, F. (2018). An enhanced
approach for quantitative prediction of personality in
facebook posts. International Journal of Education
and Management Engineering (IJEME), 8(2):8–19.
Jacob, D., Ming-Wei, C., Kenton, L., and Toutanova, K.
(2019). Bert: Pre-training of deep bidirectional trans-
formers for language understanding. In Proceedings
Stacking BERT based Models for Arabic Sentiment Analysis
149