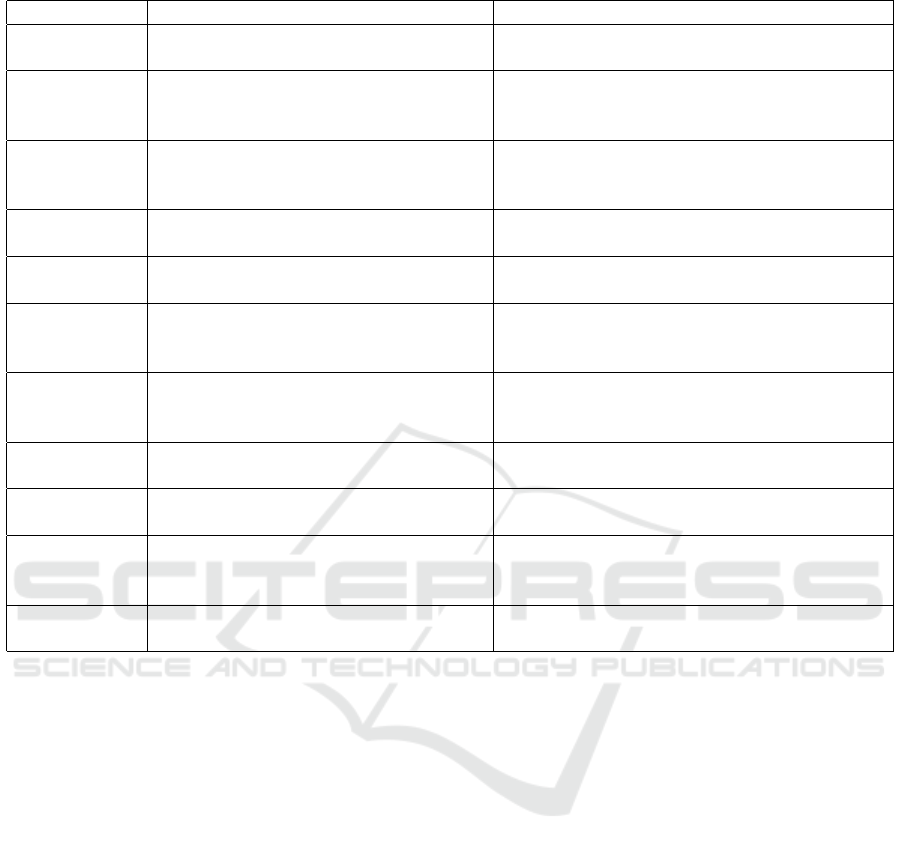
Table 1: Top words for all meaningfull topics with k = 15 of English and German data.
Topic English top words German top words
Environment pesticide, plant, soil, use, crop, fertilize,
pesticide, garden, herbicide, grow
pflanze, pestizid, dunger, boden, gulle, garten,
anbau, gemuse, tomate, feld
Retailers store, whole, shop, groceries, supermar-
ket, local, market, amazon, price, online,
discount
aldi, supermarkt, lidl, kauf, laden, lebensmittel,
cent, einkauf, wochenmarkt
GMO
& organic
gmo, label, gmos, monsanto, product,
certificate, usda, genetic, product
produkt, bioprodukt, lebensmittel, gesund, kon-
ventionell, biodiesel, herstellung, enthalt, mon-
santo, pestizid
Food products
& taste
taste, milk, sugar, cook, eat, fresh, flavor,
fruit, potato, sweet
kase, schmeckt, gurke, essen, analogkase,
schmeckt, tomate, milch, geschmack, kochen
Food safety chemical, cancer, body, acid, effect,
cause, toxic, toxin, glyphosat, disease
dioxin, gift, grenzwert, ddt, menge, giftig, tox-
isch, substanz, chemisch, antibiotika
Research science, study, scientific, research, gene,
scientist, genetic, human, stanford, na-
ture
gentechnik, natur, mensch, wissenschaft,
lebenserwartung, genetisch, studie, men-
schlich, planet
Health
& nutrition
eat, diet, healthy, nutritious, health, fat,
calory, obesity, junk
lebensmittel, essen, ernahrung, gesund,
nahrungsmittel, lebensmittel, nahrung, fett,
billig
Politics
& policy
govern, public, politic, corporate, regu-
lation, law, obama, vote
politik, skandal, verantwortung, bundestag,
schaltet, bestraft, strafe, kontrolle, kriminell
Animals
& meat
meat, chicken, anim, cow, beef, egg, fed,
raise, pig, grass
tier, fleisch, eier, huhn, schwein, futter, kuh,
verunsichert, vergiftet, deutsch
Farming farm, farmer, agriculture, land, sustain,
crop, yield, acre, grow, local
landwirtschaft, landwirt, bau, flache, okolo-
gisch, nachhaltig, konventionell, landbau, pro-
duktion, ertrag
Prices & profit price, consume, market, company, profit,
product, cost, amazon, money
verbrauch, preis, produkt, billig, qualitat,
kunde, kauf, geld, unternehmen, kosten
(De Smedt and Daelemans, 2012). Since the subjec-
tivity assignment is not well-developed in Textblob-
de, we filter out sentences with polarity equals to 0 for
both English and German sentences in order to derive
comparable results.
3.6 Topic and Sentiment Distributions
After assigning a labeled cluster, i.e., a topic, and a
sentiment score to each sentence of the corpus, we
derive the corresponding distributions.
For topic distributions, all sentences from a doc-
ument are counted per topic. The distribution is then
normalized to be comparable. For sentiment distri-
butions, all sentences from a document are grouped
per topic. Topic-wise sentiment distribution is derived
based on the sentence-wise polarity scores and the re-
spective median and quartiles. A document in that
regard is either an article or all of its comments, i.e.,
its comment section.
4 TOPIC COHERENCE
In this section, we evaluate the feasibility and se-
mantic coherence of our cross-lingual topic modeling
qualitatively. Instead of providing quantitative coher-
ence scores, we aim at a detailed, qualitative analy-
sis of textual examples. We depict representative sen-
tences and words of each topic in subsection 4.2. We
investigate to what extent these are semantically co-
herent, also across languages. We expose the ratio
of coherent and incoherent topics and how it devel-
ops with increasing number of topics in in subsection
4.3. Eventually, we show the distribution of topics in
selected newspaper articles and their respective com-
ment sections to relate the discussed content with our
actual topic model on English and German texts.
4.1 Data
The collection of the data used in this study is de-
scribed in another publication (Danner et al., 2021) as
follows. For the analysis we downloaded ”news arti-
KDIR 2021 - 13th International Conference on Knowledge Discovery and Information Retrieval
20